Yoshua Bengio
(113 articles)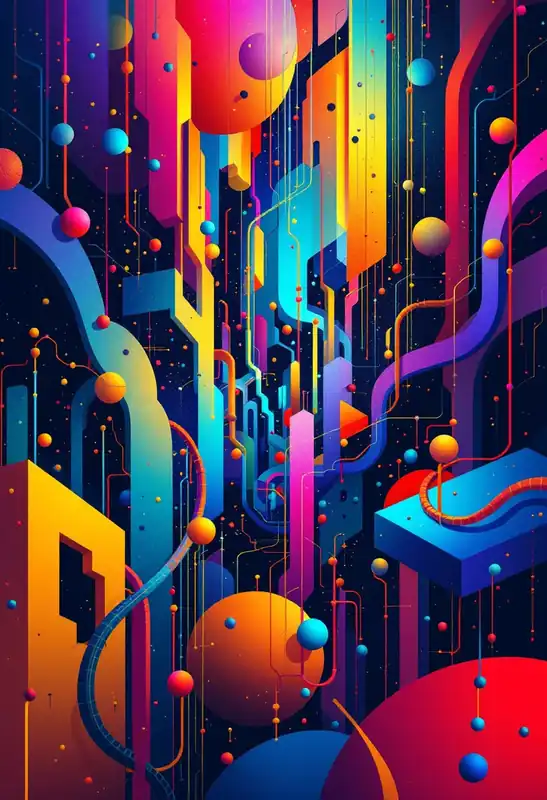
Parameterized
Model or function in AI that utilizes parameters to make predictions or decisions.
Generality: 796
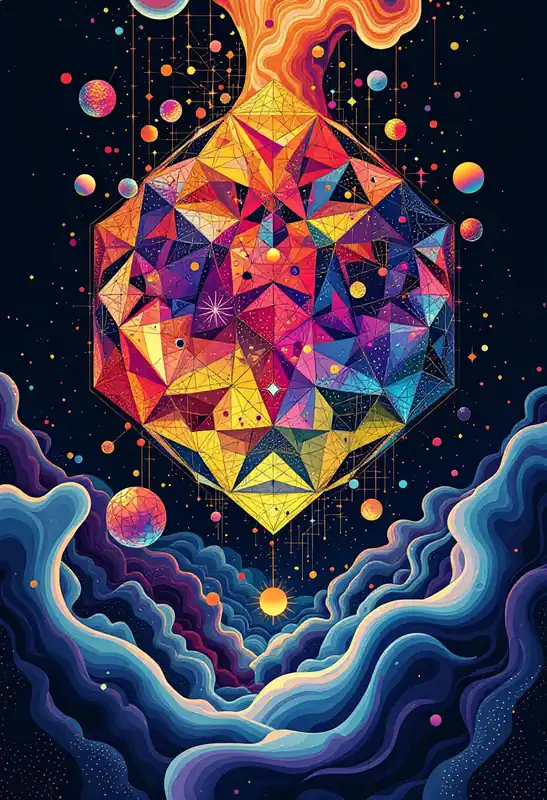
Loss Optimization
Process of adjusting a model's parameters to minimize the difference between the predicted outputs and the actual outputs, measured by a loss function.
Generality: 886
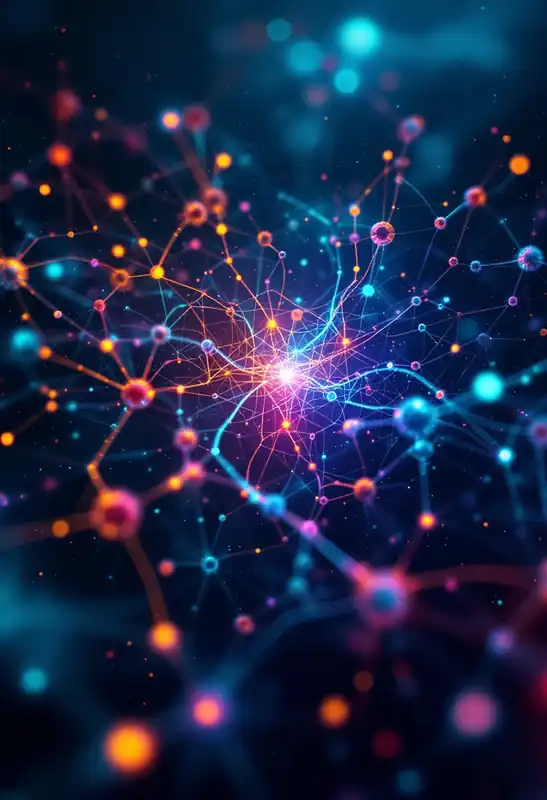
ANN
Artificial Neural Networks
Artificial Neural Networks
Computing systems inspired by the biological neural networks that constitute animal brains, designed to progressively improve their performance on tasks by considering examples.
Generality: 875
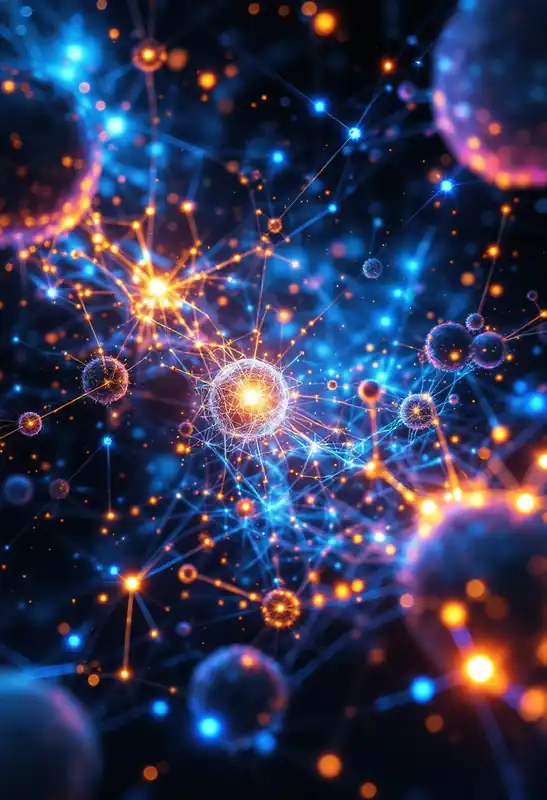
Neural Network
Computing system designed to simulate the way human brains analyze and process information, using a network of interconnected nodes that work together to solve specific problems.
Generality: 932
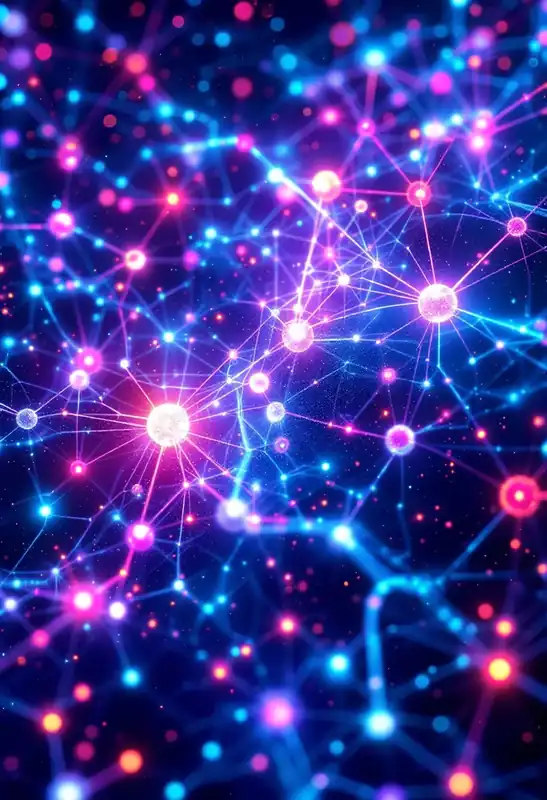
Connectionist AI
Set of computational models in AI that simulate the human brain's network of neurons to process information and learn from data.
Generality: 900
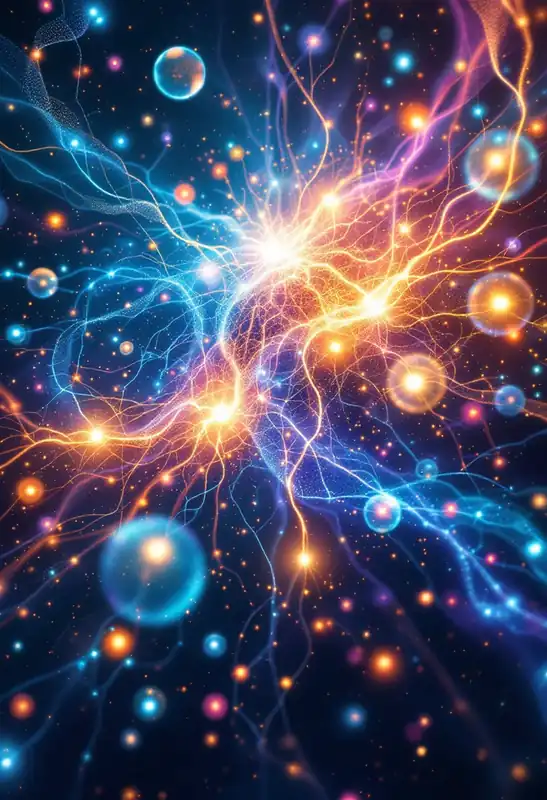
Next Word Prediction
Enables language models to predict the most probable subsequent word in a text sequence using generative AI techniques.
Generality: 780
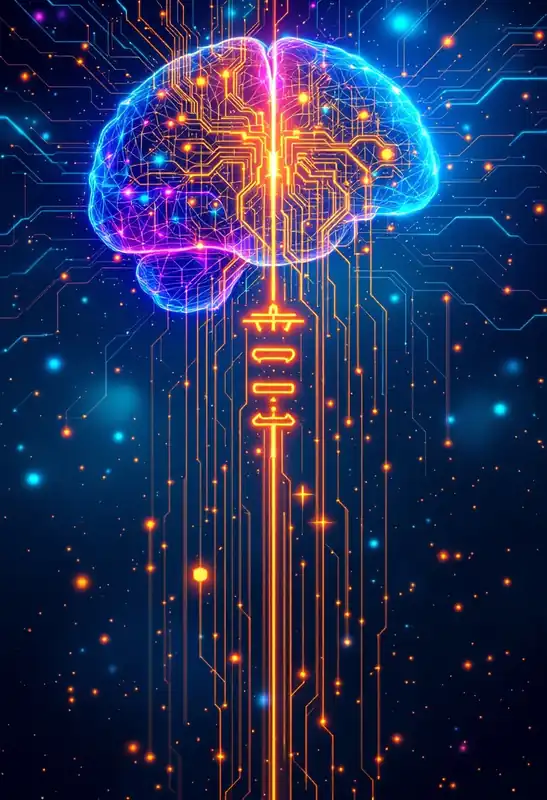
NLP
Natural Language Processing
Natural Language Processing
Field of AI that focuses on the interaction between computers and humans through natural language.
Generality: 931
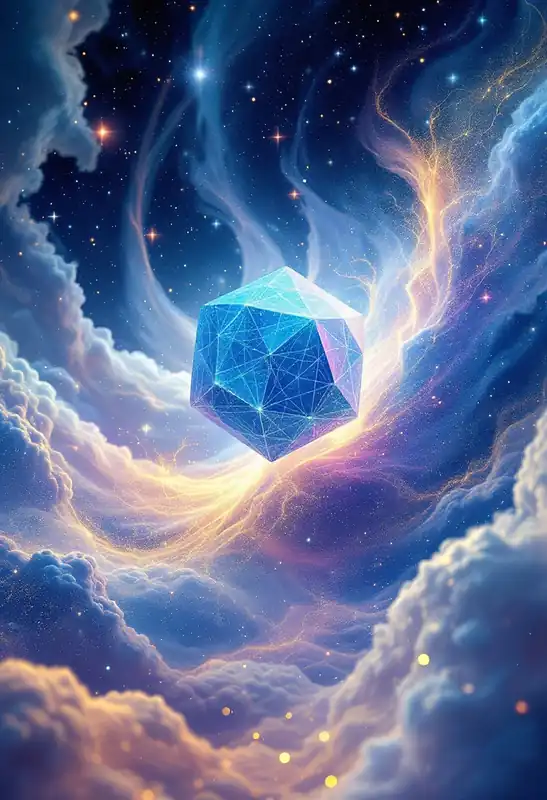
Generalization
Ability of a ML model to perform well on new, unseen data that was not included in the training set.
Generality: 891
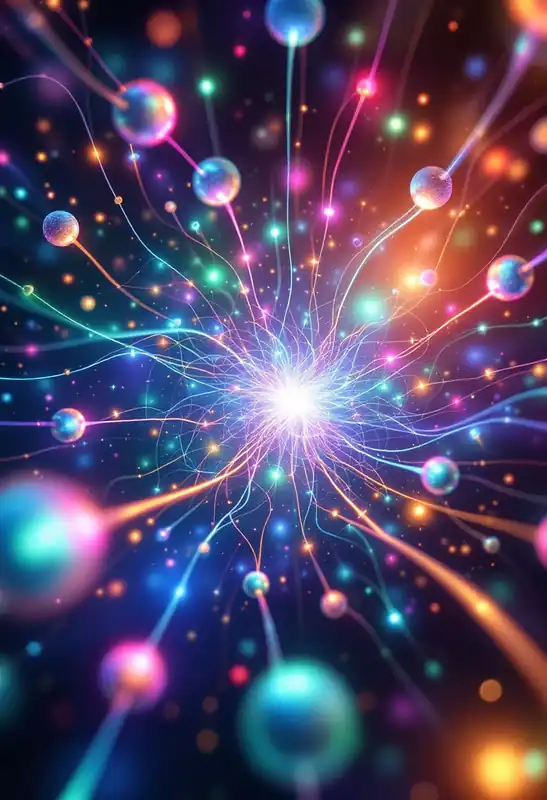
Supervision
Use of labeled data to train ML models, guiding the learning process by providing input-output pairs.
Generality: 890
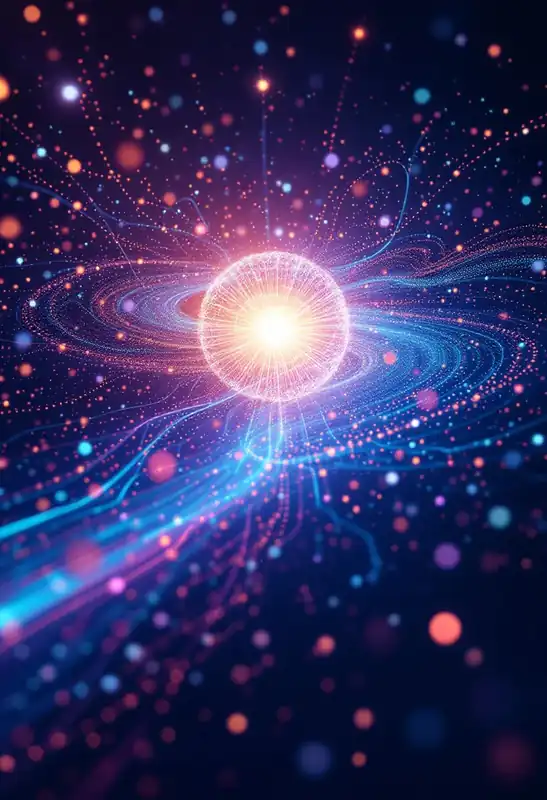
Training
Process of teaching a ML model to make accurate predictions or decisions, by adjusting its parameters based on data.
Generality: 940
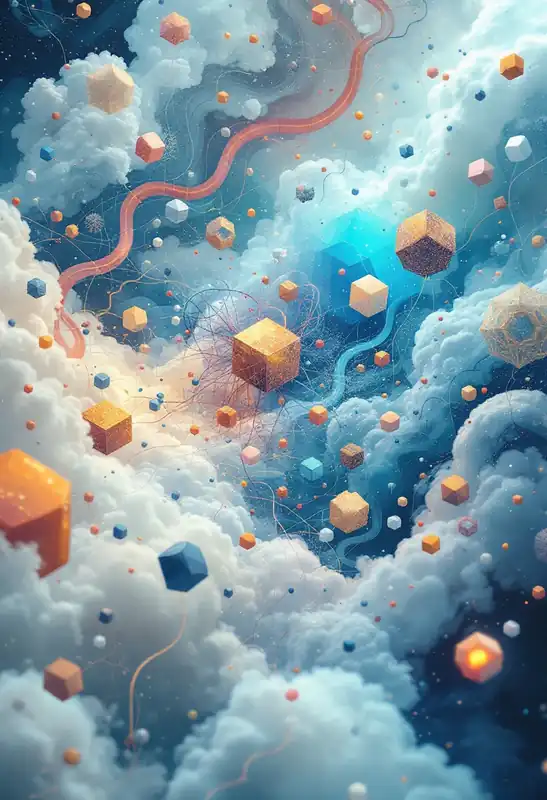
Unsupervised Learning
Type of ML where algorithms learn patterns from untagged data, without any guidance on what outcomes to predict.
Generality: 905
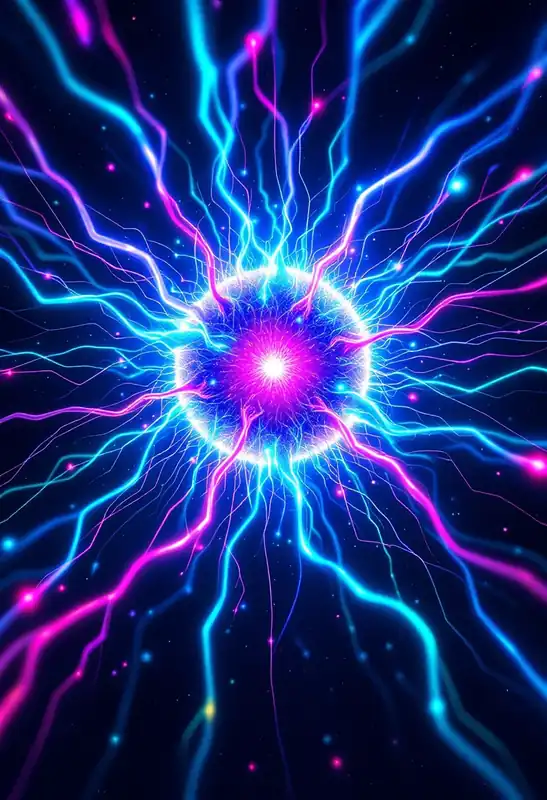
Artificial Neuron
Computational models inspired by biological neurons, serving as the foundational units of artificial neural networks to process input and output signals.
Generality: 825
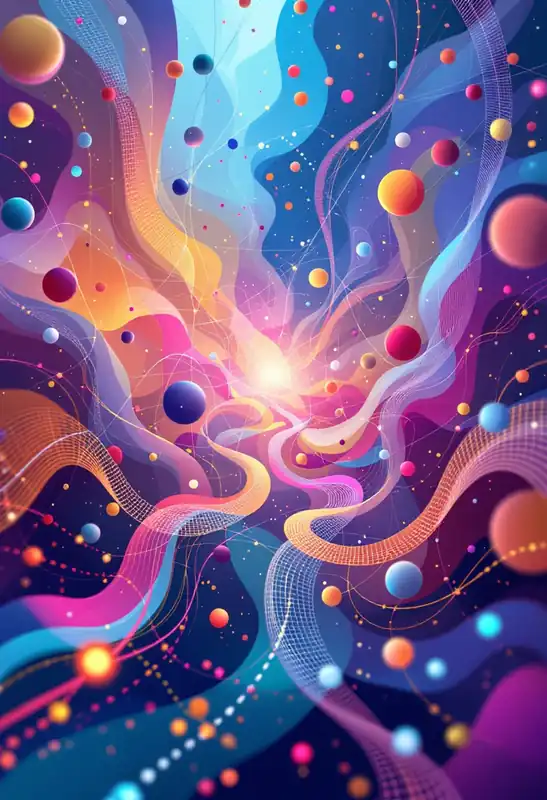
Pattern Recognition
The identification and classification of patterns in data using computational algorithms, essential for enabling machines to interpret, learn from, and make decisions based on complex datasets.
Generality: 825
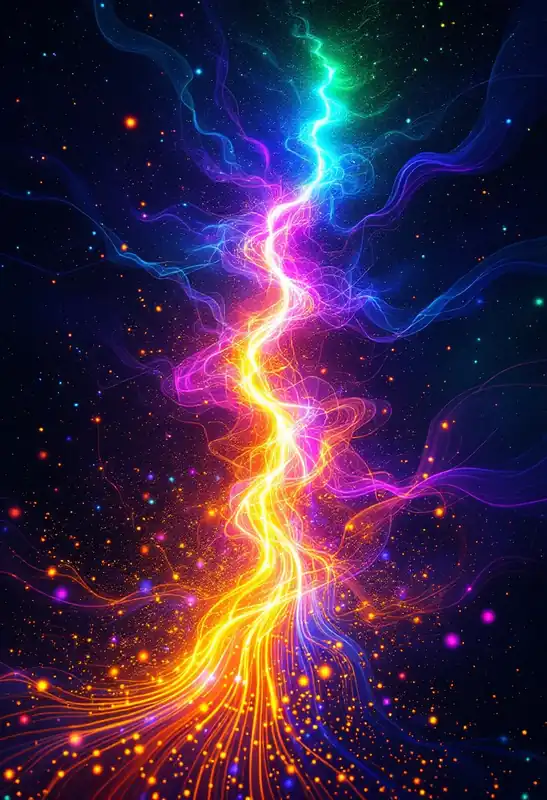
Feed Forward
Essential structure of an artificial neural network that directs data or information from the input layer towards the output layer without looping back.
Generality: 860
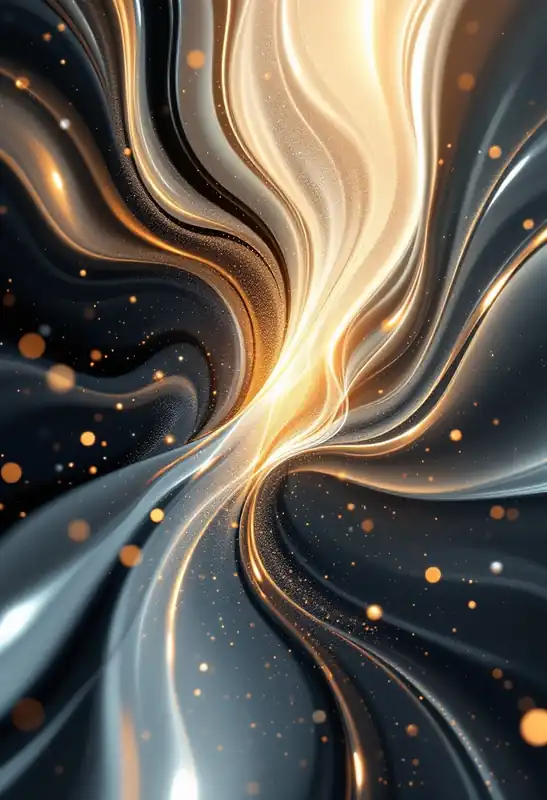
Invariance
Property of a model or algorithm that ensures its output remains unchanged when specific transformations are applied to the input data.
Generality: 830
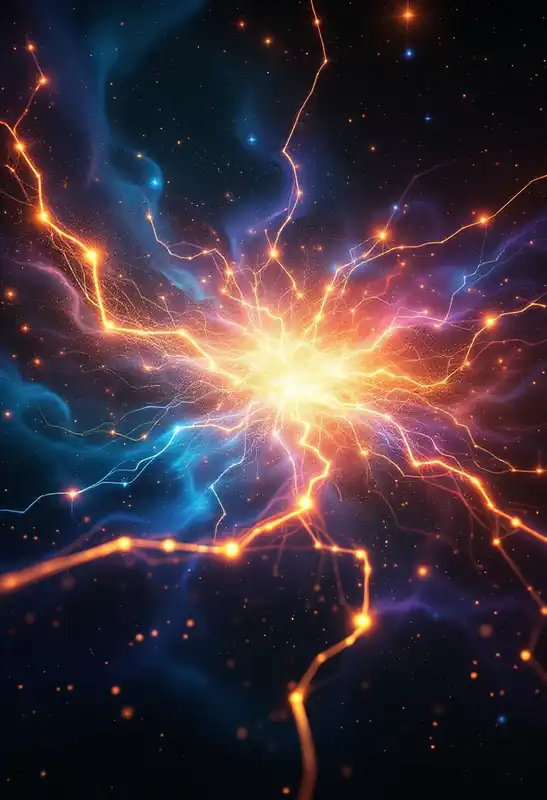
Inference
Process by which a trained neural network applies learned patterns to new, unseen data to make predictions or decisions.
Generality: 861
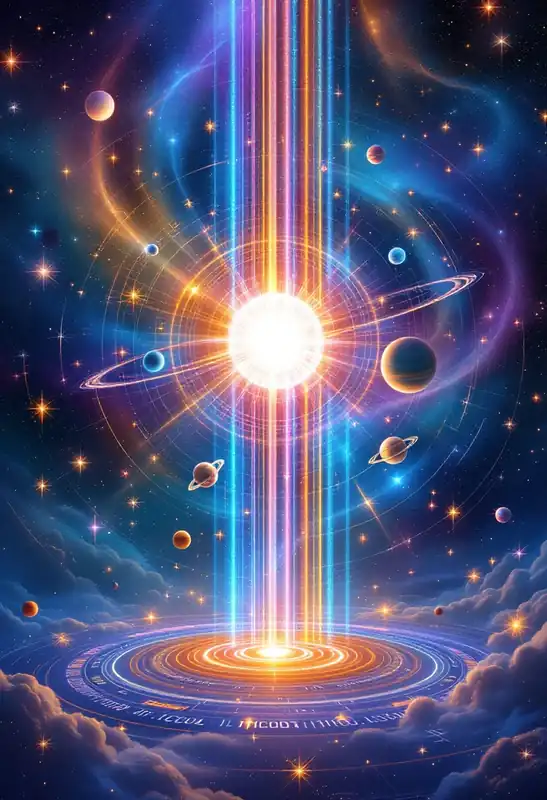
NLU
Natural Language Understanding
Natural Language Understanding
Subfield of NLP focused on enabling machines to understand and interpret human language in a way that is both meaningful and contextually relevant.
Generality: 894
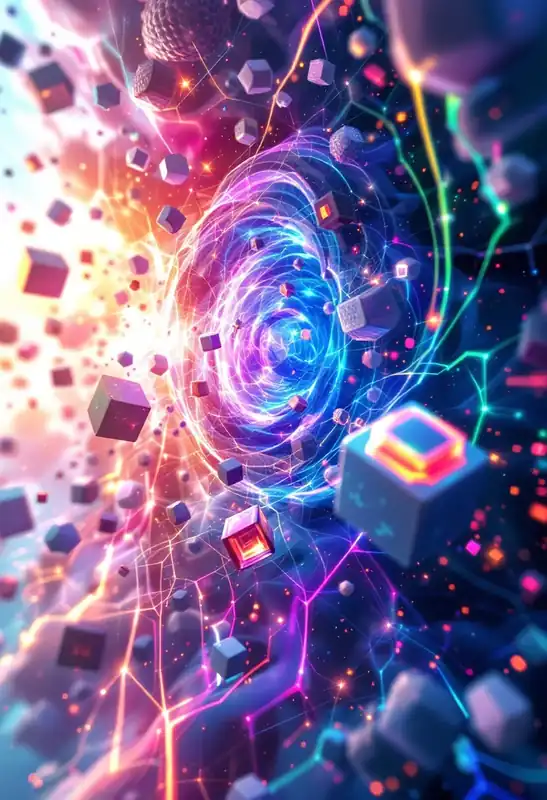
CNN
Convolutional Neural Network
Convolutional Neural Network
Deep learning algorithm that can capture spatial hierarchies in data, particularly useful for image and video recognition tasks.
Generality: 916
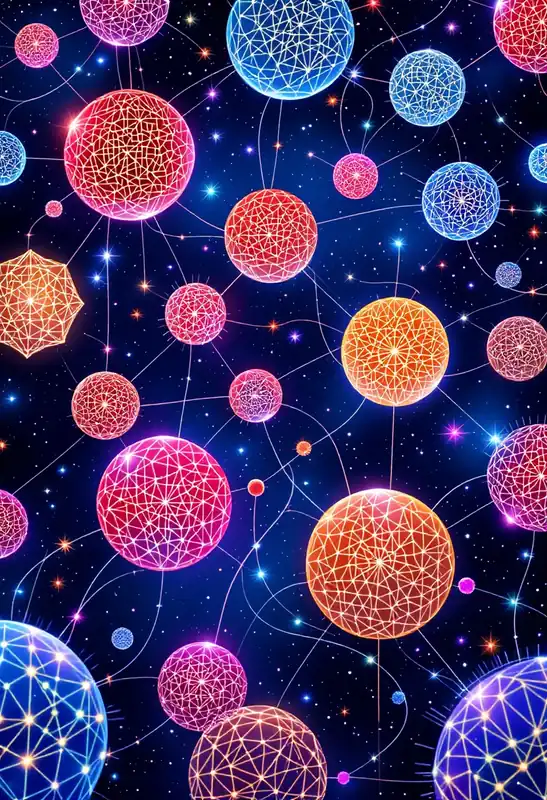
Local Weight Sharing
Technique where the same weights are used across different positions in an input, enhancing the network's ability to recognize patterns irrespective of their spatial location.
Generality: 690
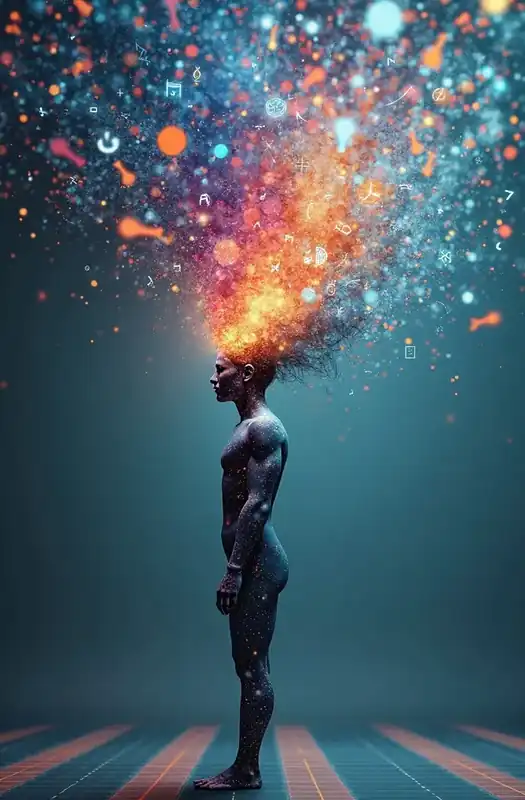
Generative
Subset of AI technologies capable of generating new content, ideas, or data that mimic human-like outputs.
Generality: 840
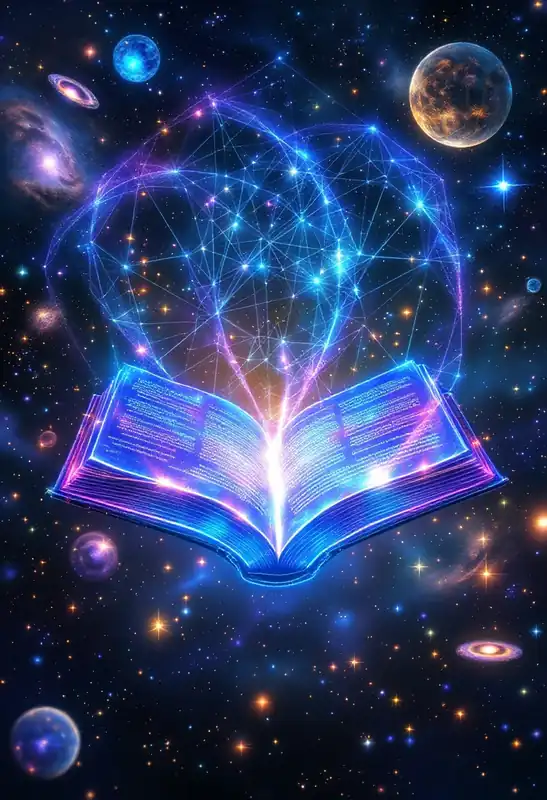
Universal Learning Algorithms
Theoretical frameworks aimed at creating systems capable of learning any task to human-level competency, leveraging principles that could allow for generalization across diverse domains.
Generality: 840
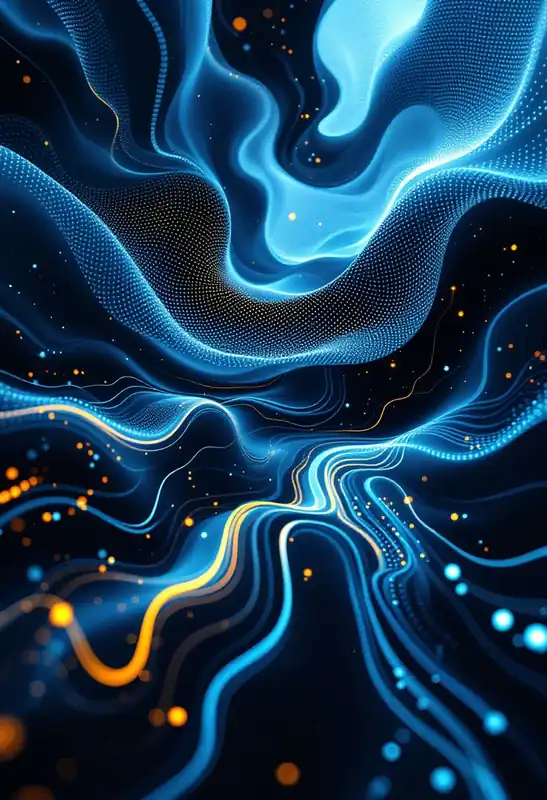
Learnability
Capacity of an algorithm or model to effectively learn from data, often measured by how well it can generalize from training data to unseen data.
Generality: 847
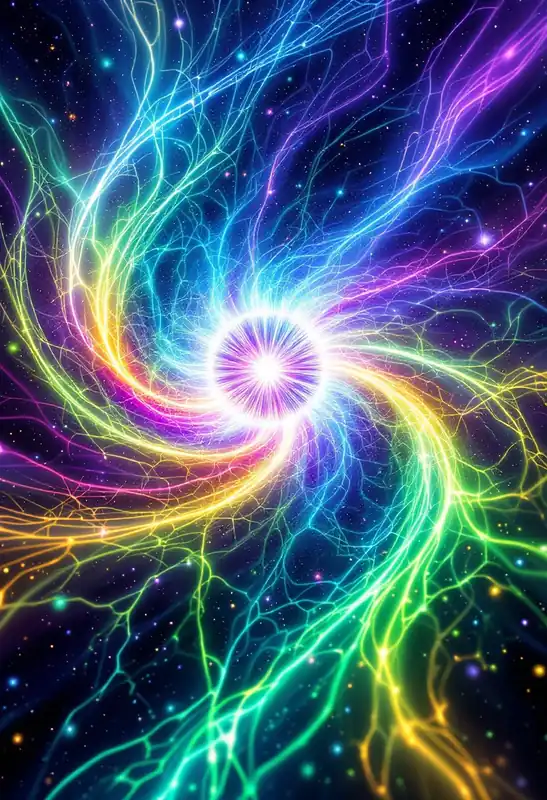
EBM
Energy-Based Model
Energy-Based Model
Class of deep learning models that learn to associate lower energy levels with more probable configurations of the input data.
Generality: 625
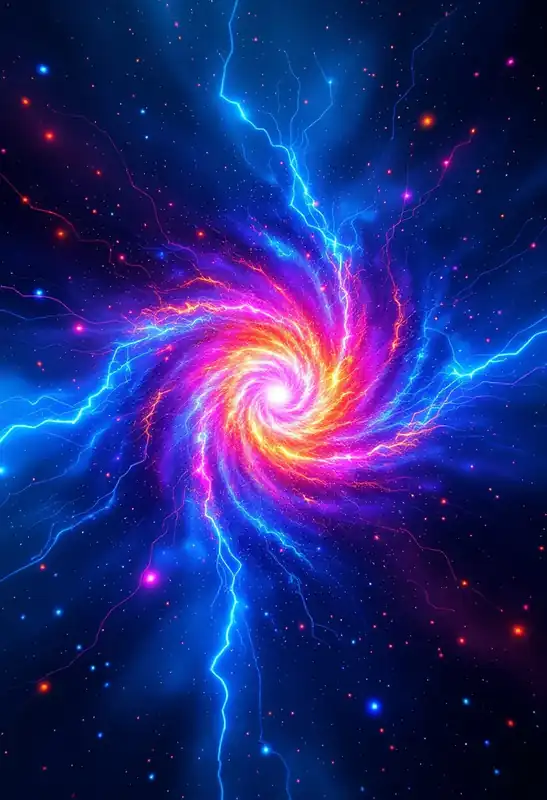
Saturating Non-Linearities
Activation functions in neural networks that reach a point where their output changes very little, or not at all, in response to large input values.
Generality: 575
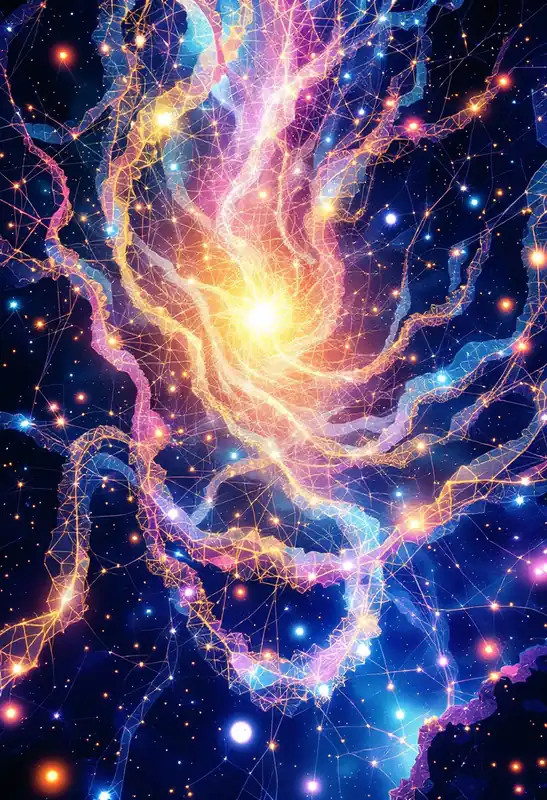
DL
Deep Learning
Deep Learning
Subset of machine learning that involves neural networks with many layers, enabling the modeling of complex patterns in data.
Generality: 905
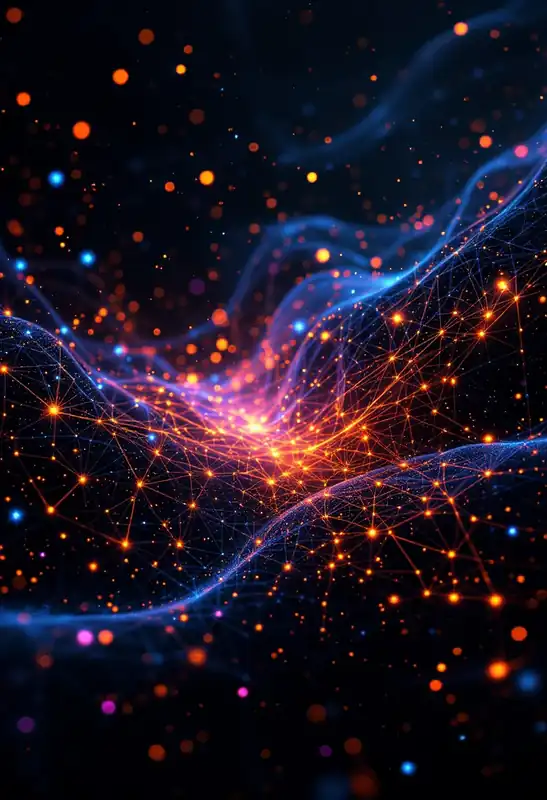
DNN
Deep Neural Networks
Deep Neural Networks
Advanced neural network architectures with multiple layers that enable complex pattern recognition and learning from large amounts of data.
Generality: 916
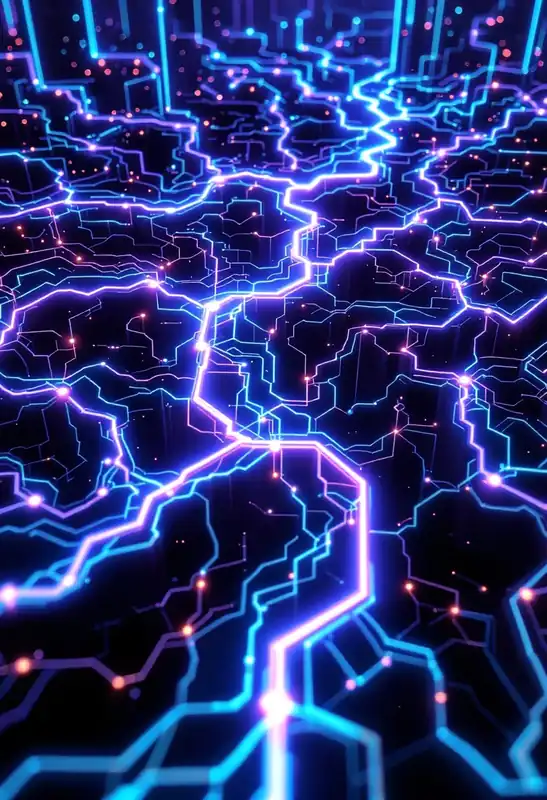
Subsymbolic AI
AI approaches that do not use explicit symbolic representation of knowledge but instead rely on distributed, often neural network-based methods to process and learn from data.
Generality: 900
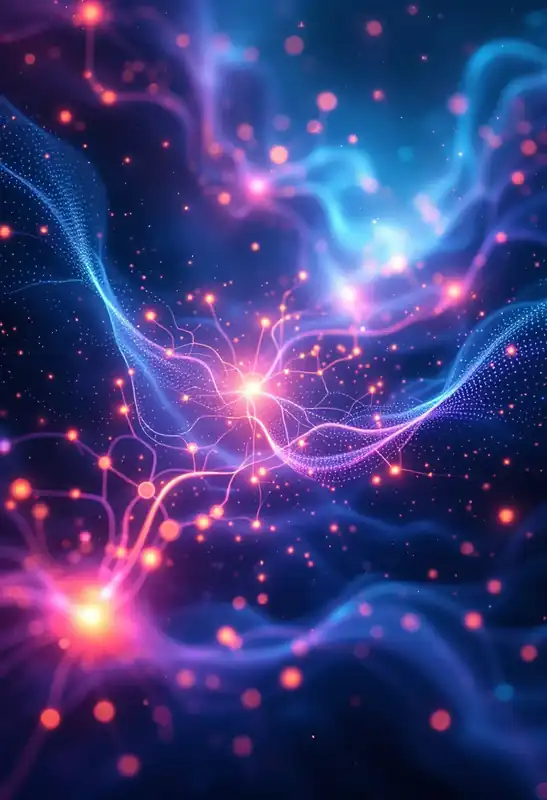
RNN
Recurrent Neural Network
Recurrent Neural Network
Class of neural networks where connections between nodes form a directed graph along a temporal sequence, enabling them to exhibit temporal dynamic behavior for a sequence of inputs.
Generality: 892
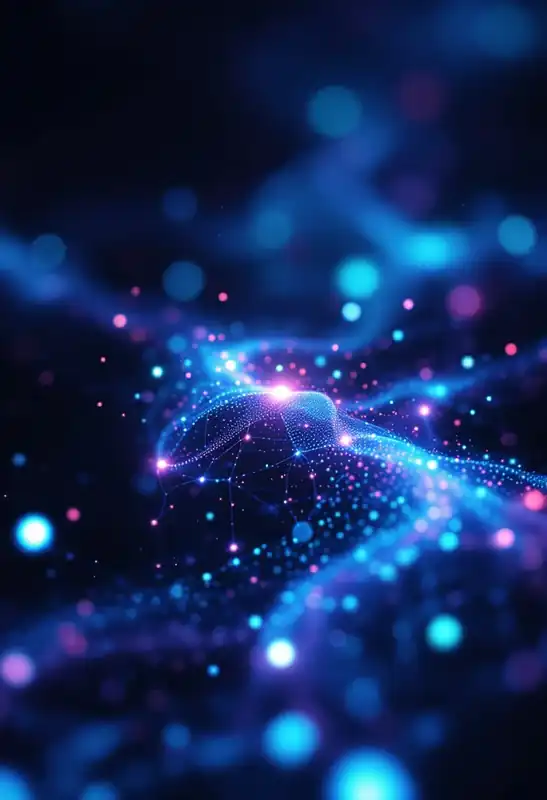
Hidden Layer
Layer of neurons in an artificial neural network that processes inputs from the previous layer, transforming the data before passing it on to the next layer, without direct exposure to the input or output data.
Generality: 861
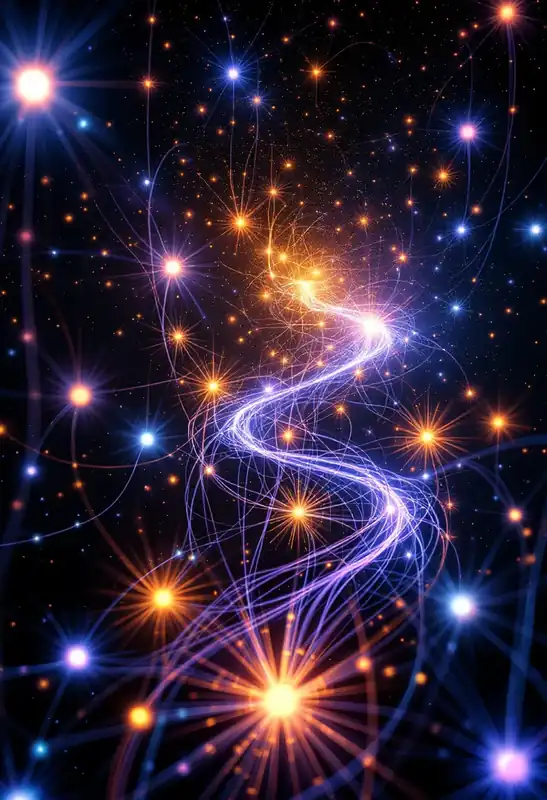
Forward Propagation
Process in a neural network where input data is passed through layers of the network to generate output.
Generality: 830
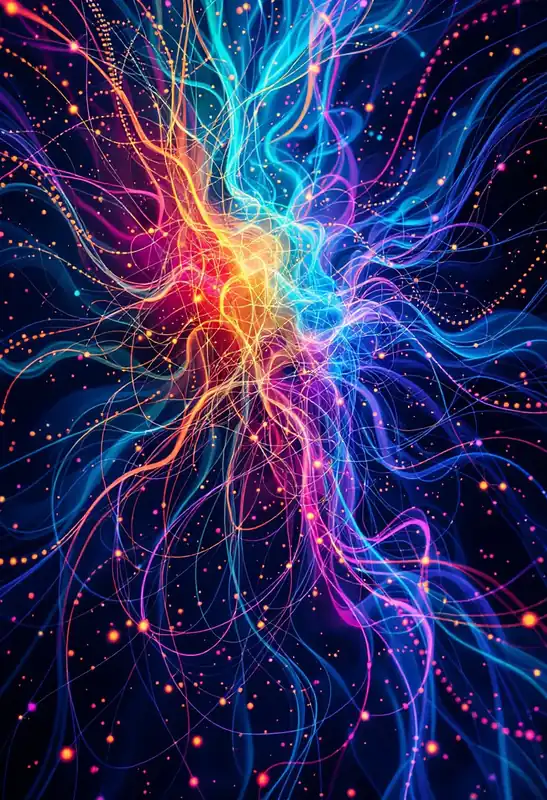
Weight Initialization
An essential process in neural network training that involves setting the initial values of the model's weights to influence learning effectiveness and convergence.
Generality: 675
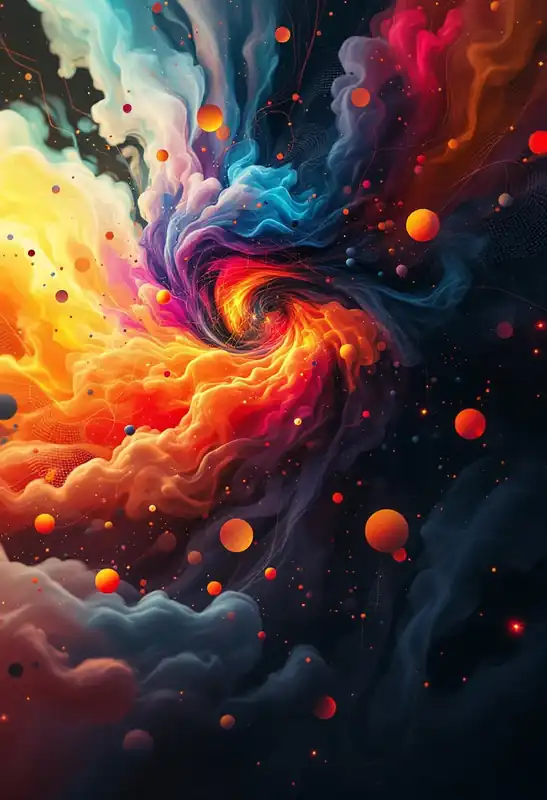
Prediction Error
The discrepancy between predicted outcomes by an AI model and the actual observed results in a dataset.
Generality: 675
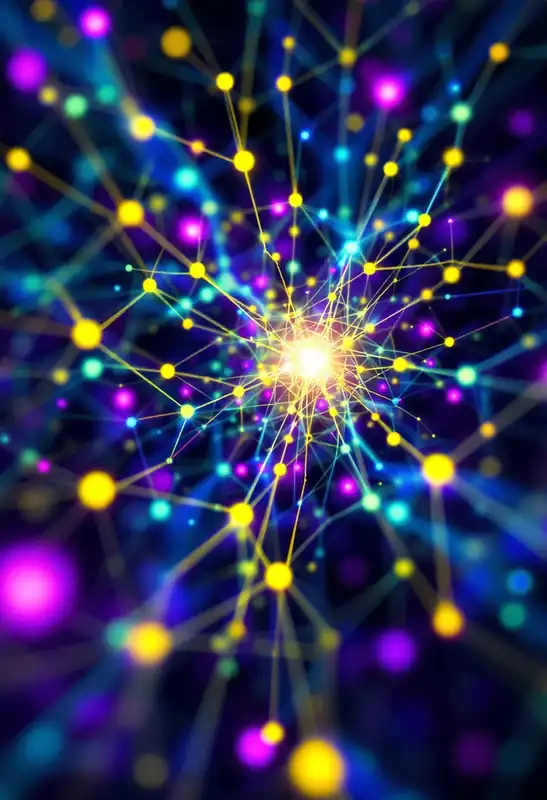
Node
A fundamental unit within a neural network or graph that processes inputs to produce outputs, often reflecting the biological concept of neurons.
Generality: 500
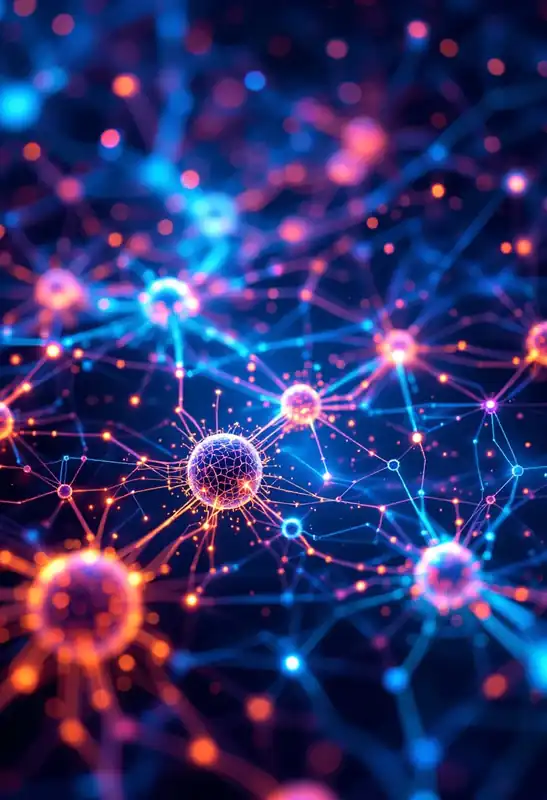
Batch
A collection of data samples processed simultaneously in a single step of a neural network's training process.
Generality: 500
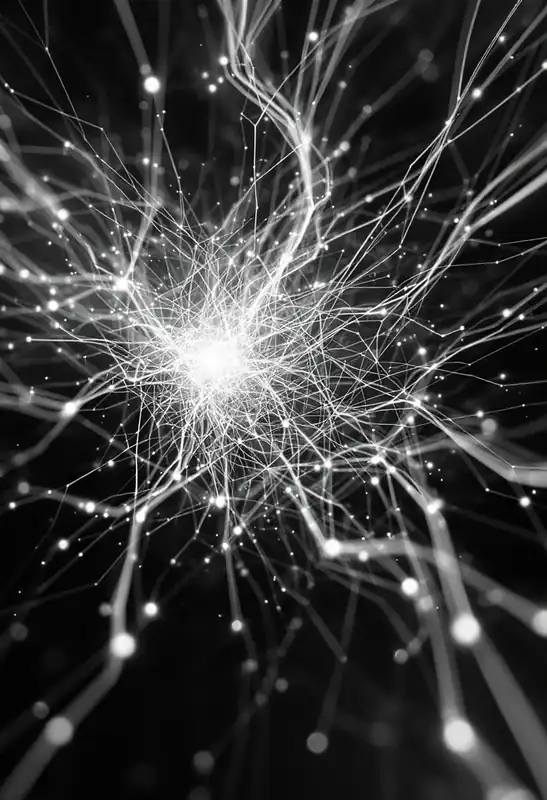
Weight Decay
Regularization technique used in training neural networks to prevent overfitting by penalizing large weights.
Generality: 730
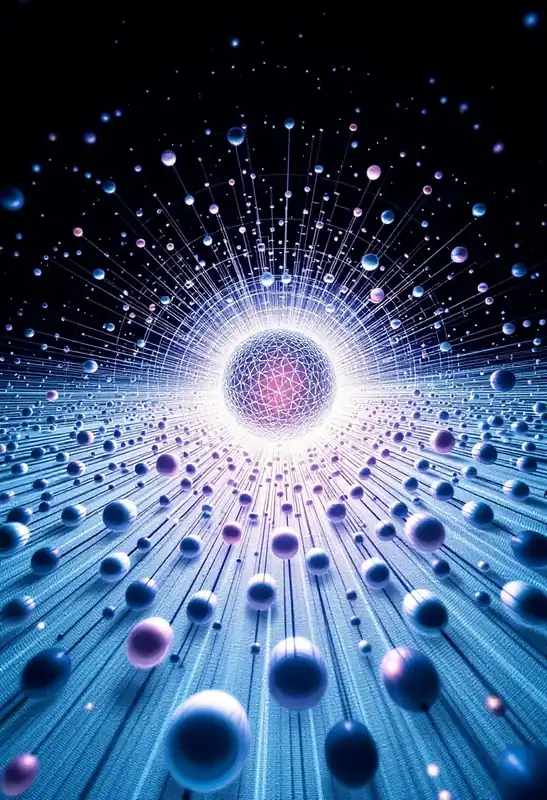
Autoencoder
Type of artificial neural network used to learn efficient codings of unlabeled data, typically for the purpose of dimensionality reduction or feature learning.
Generality: 815
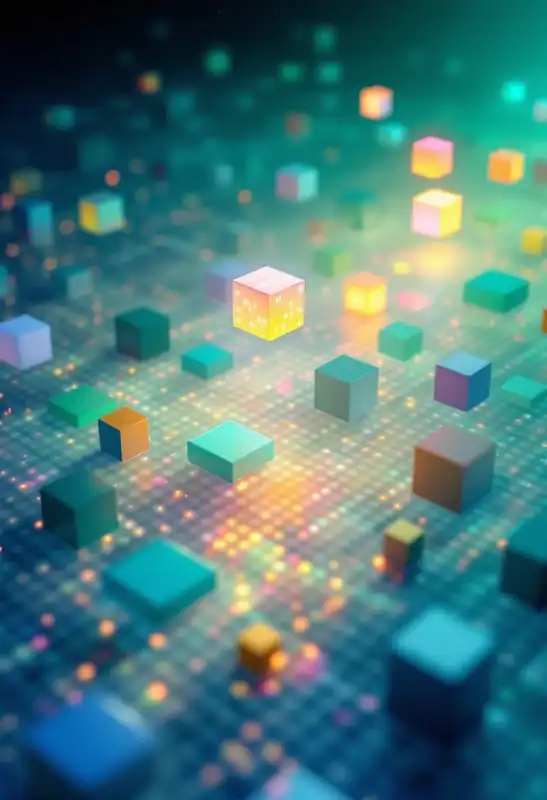
Max Pooling
Downsampling technique that reduces the dimensionality of input data by selecting the maximum value from a specified subset of the data.
Generality: 695
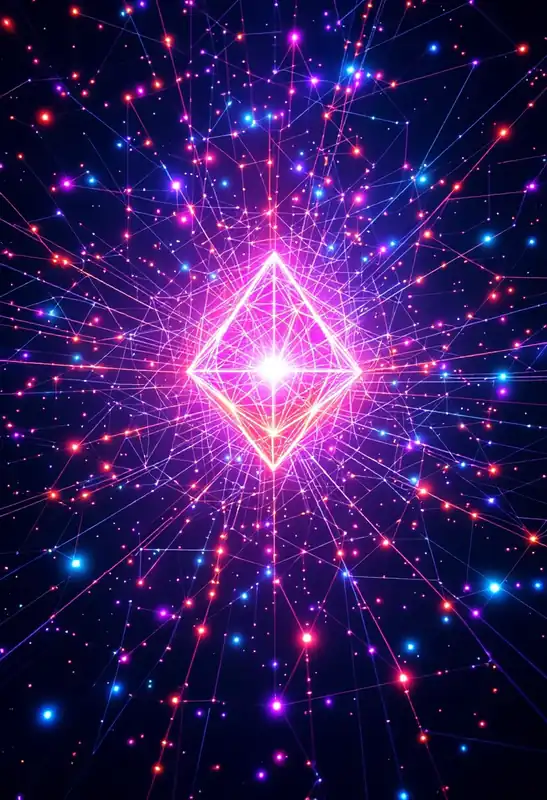
SotA
State of the Art
State of the Art
The highest level of performance achieved in a specific field, particularly in AI, where it denotes the most advanced model or algorithm.
Generality: 720
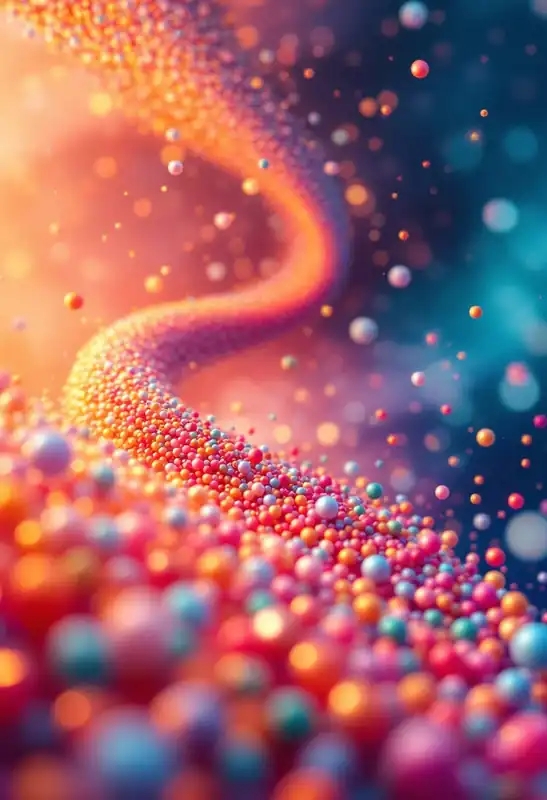
Incremental Learning
A method where AI systems continuously acquire new data and knowledge while retaining previously learned information without retraining from scratch.
Generality: 750
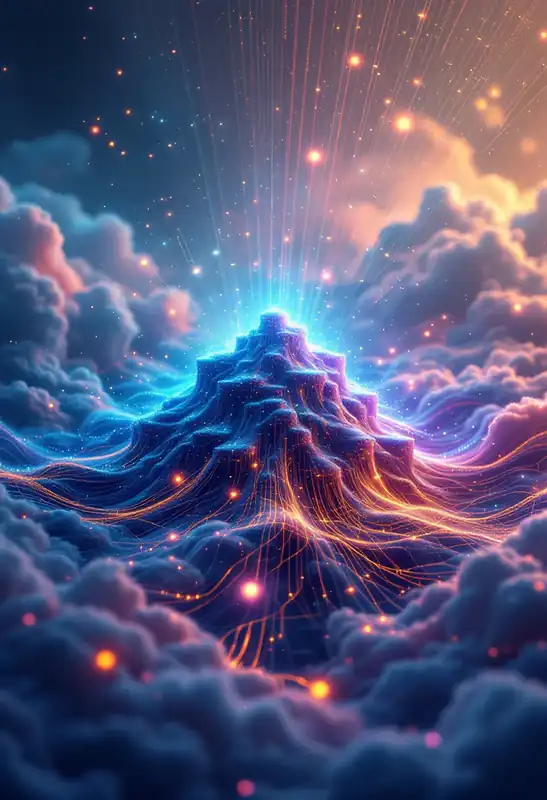
Vanishing Gradient
Phenomenon in neural networks where gradients of the network's parameters become very small, effectively preventing the weights from changing their values during training.
Generality: 773
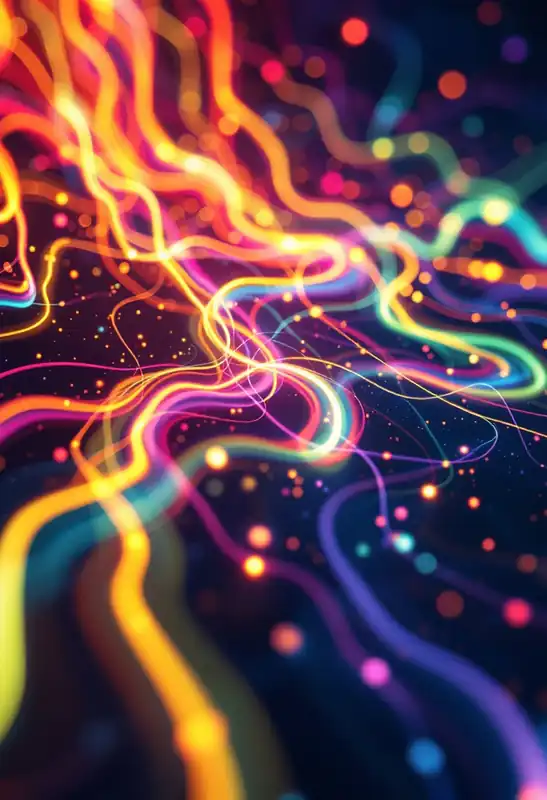
Policy Gradient Algorithm
Type of RL algorithm that optimizes the policy directly by computing gradients of expected rewards with respect to policy parameters.
Generality: 805
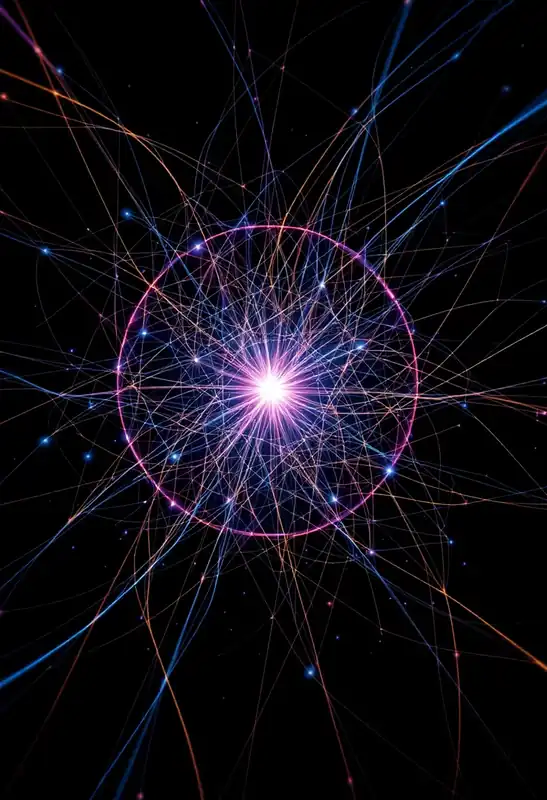
MTL
Multi-Task Learning
Multi-Task Learning
ML approach where a single model is trained simultaneously on multiple related tasks, leveraging commonalities and differences across tasks to improve generalization.
Generality: 761
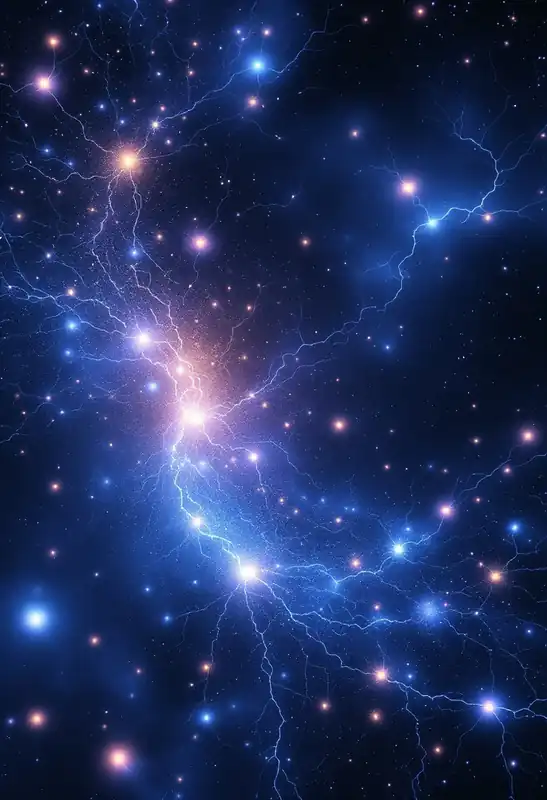
Wake Sleep
Biologically inspired algorithm used within unsupervised learning to train deep belief networks.
Generality: 540
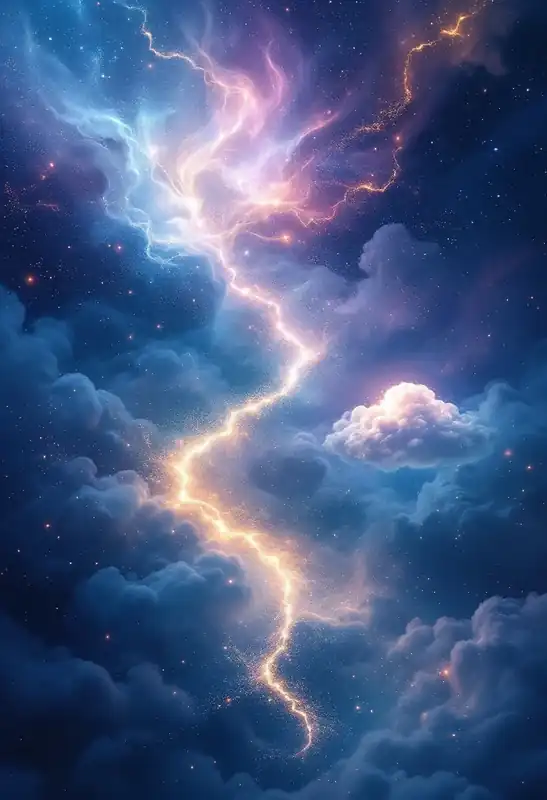
Transfer Learning
ML method where a model developed for a task is reused as the starting point for a model on a second task, leveraging the knowledge gained from the first task to improve performance on the second.
Generality: 870
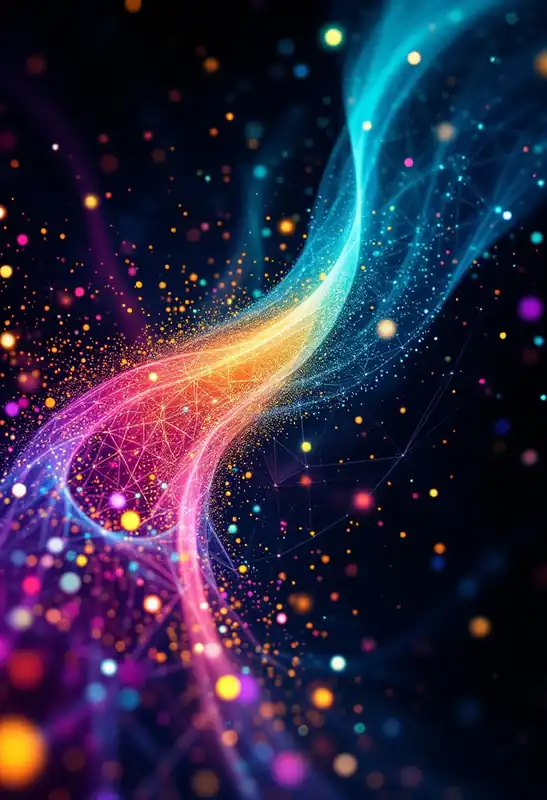
Continuous Learning
Systems and models that learn incrementally from a stream of data, updating their knowledge without forgetting previous information.
Generality: 870
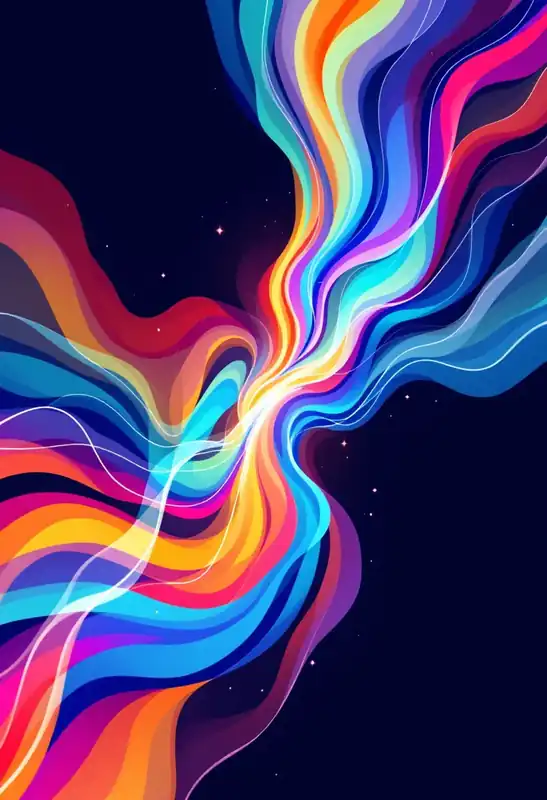
Early Stopping
A regularization technique used to prevent overfitting in ML models by halting training when performance on a validation set begins to degrade.
Generality: 675
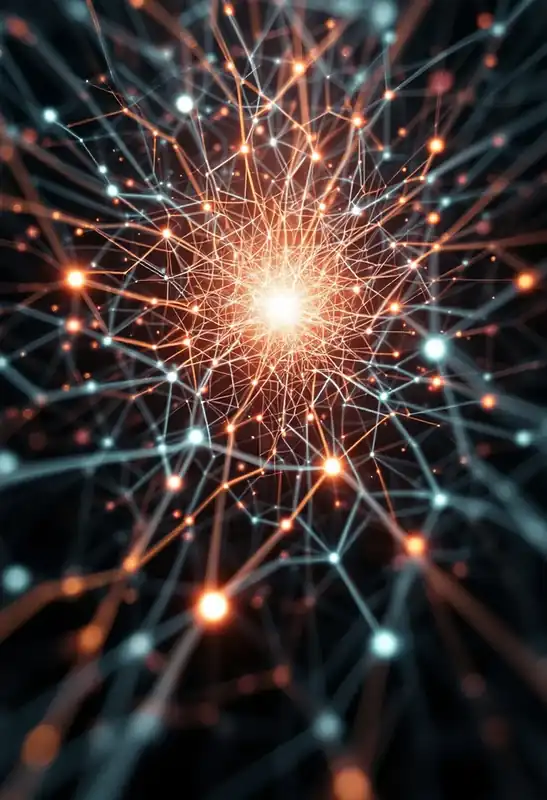
SNN
Spiking Neural Network
Spiking Neural Network
Type of artificial neural network that mimics the way biological neural networks in the brain process information, using spikes of electrical activity to transmit and process information.
Generality: 830
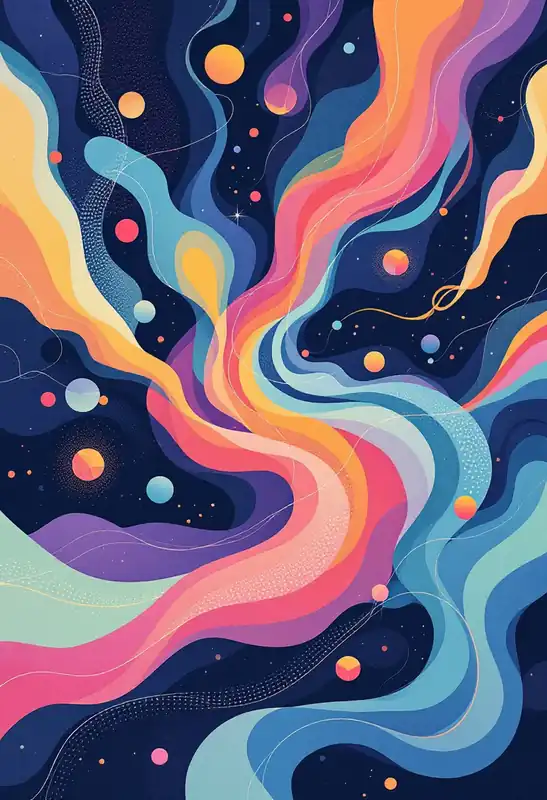
Transfer Capability
A feature of AI systems that allows acquired knowledge in one domain or task to be applied to another distinct but related domain or task.
Generality: 775
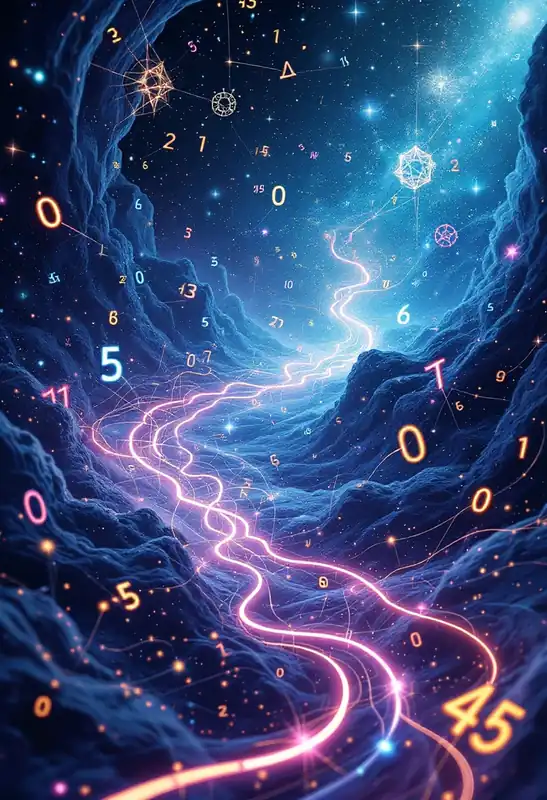
Word Vector
Numerical representations of words that capture their meanings, relationships, and context within a language.
Generality: 690
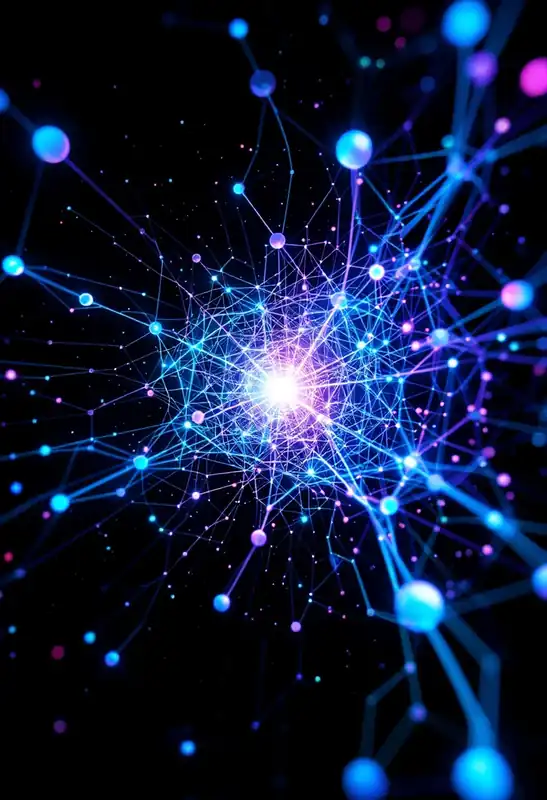
DBN
Deep Belief Network
Deep Belief Network
A type of artificial neural network that is deeply structured with multiple layers of latent variables, or hidden units.
Generality: 851
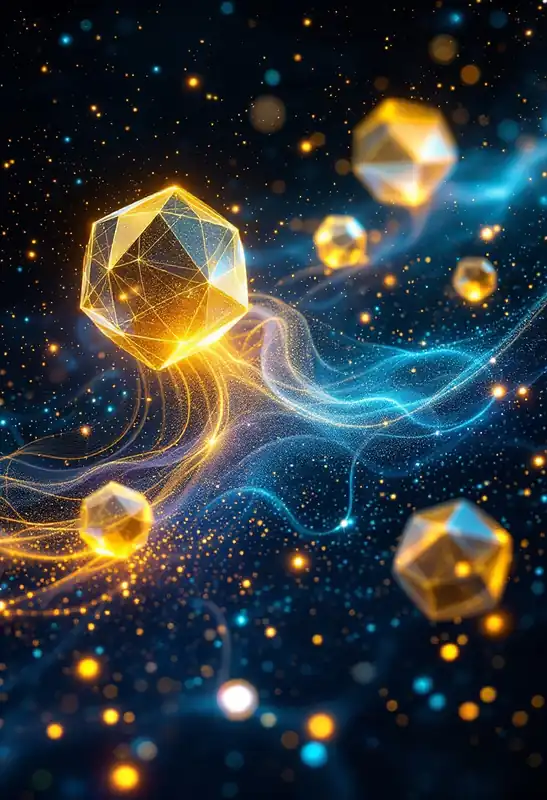
Semi-Supervised Learning
ML approach that uses a combination of a small amount of labeled data and a large amount of unlabeled data for training models.
Generality: 800
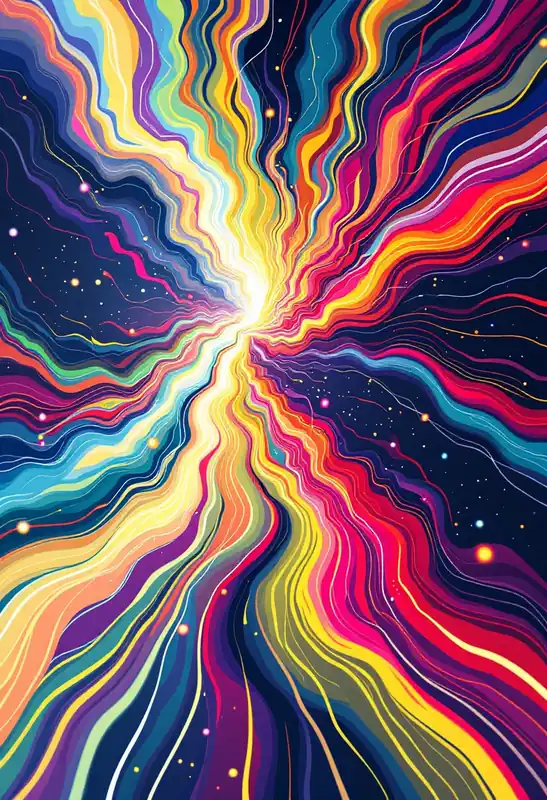
Feature Learning
Automatically learning representations or features from raw input data in order to improve model performance and reduce dependency on manual feature engineering.
Generality: 500
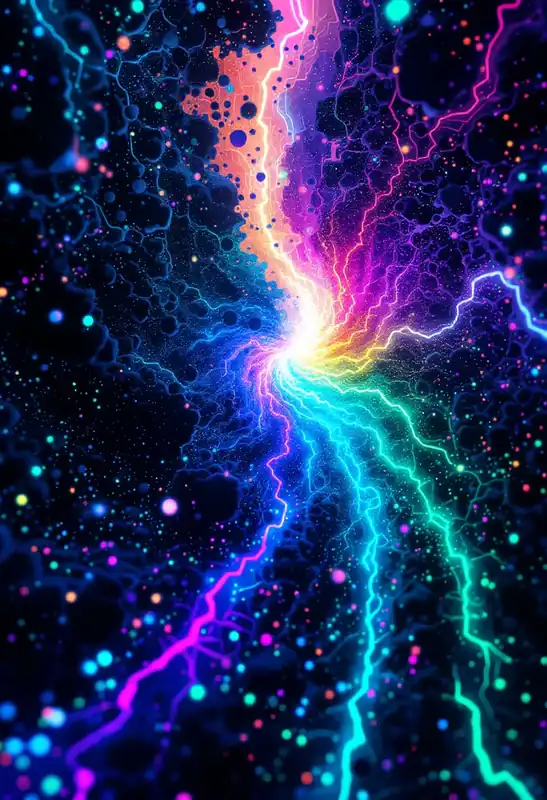
Denoising Autoencoder
A neural network designed to reconstruct a clean input from a corrupted version, enhancing feature extraction by learning robust data representations.
Generality: 806
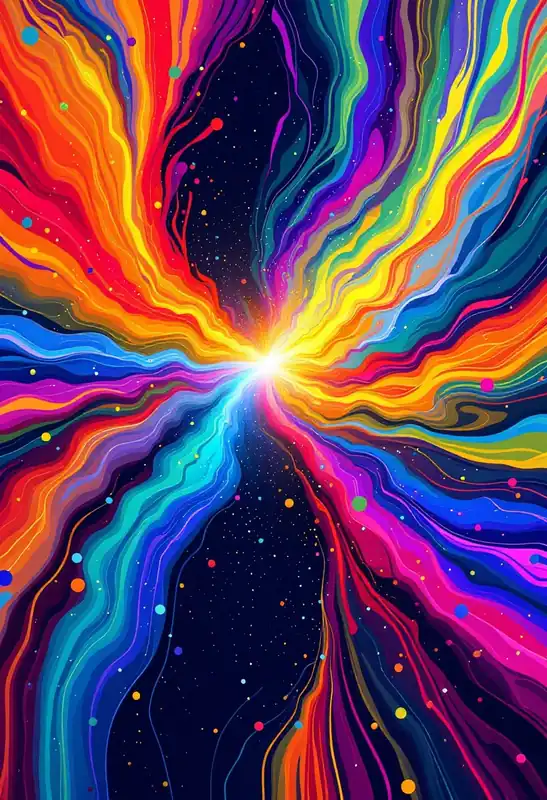
Xavier's Initialization
Weight initialization technique designed to keep the variance of the outputs of a neuron approximately equal to the variance of its inputs across layers in a deep neural network.
Generality: 669
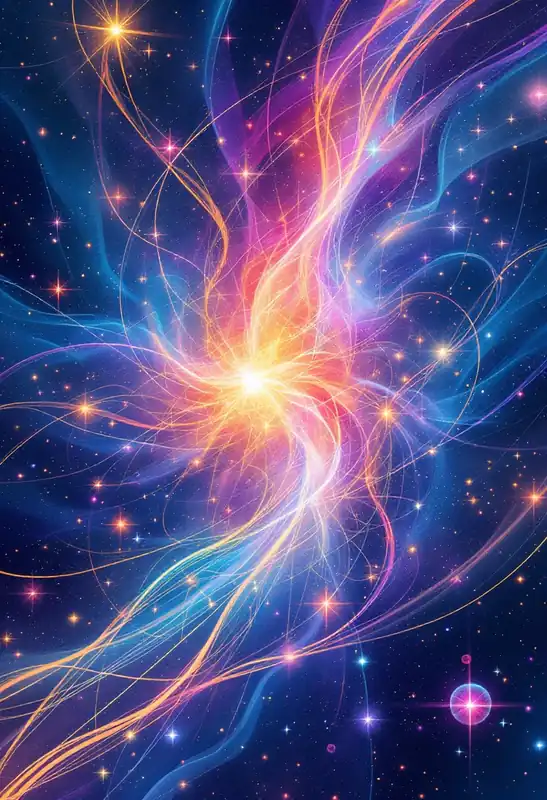
Initialization
Process of setting the initial values of the parameters (weights and biases) of a model before training begins.
Generality: 865
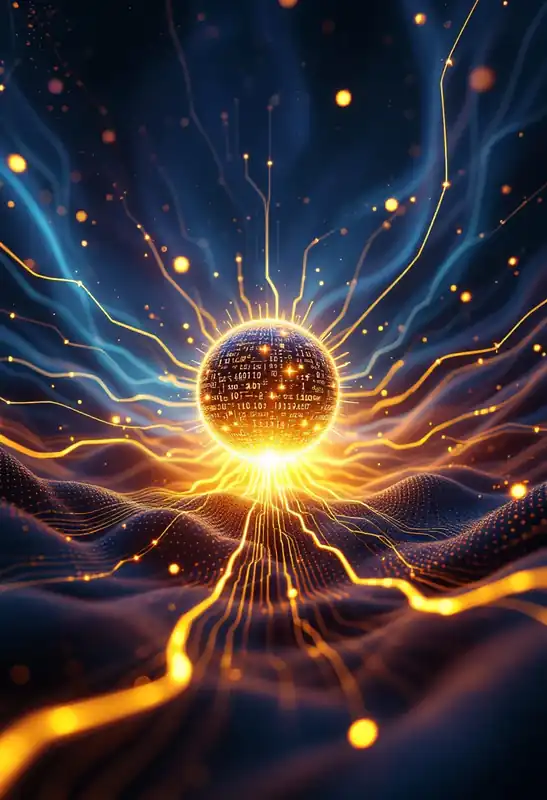
Data Efficient Learning
ML approach that requires fewer data to train a functional model.
Generality: 791
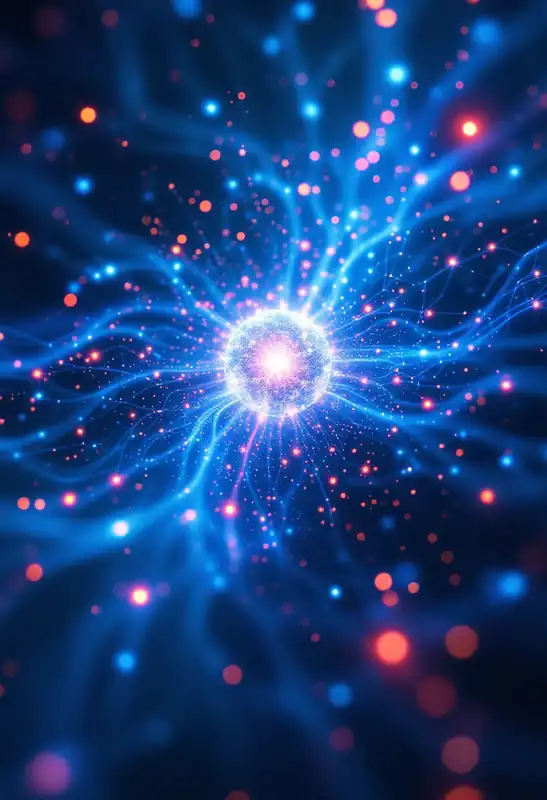
Similarity Learning
A technique in AI focusing on training models to measure task-related similarity between data points.
Generality: 675
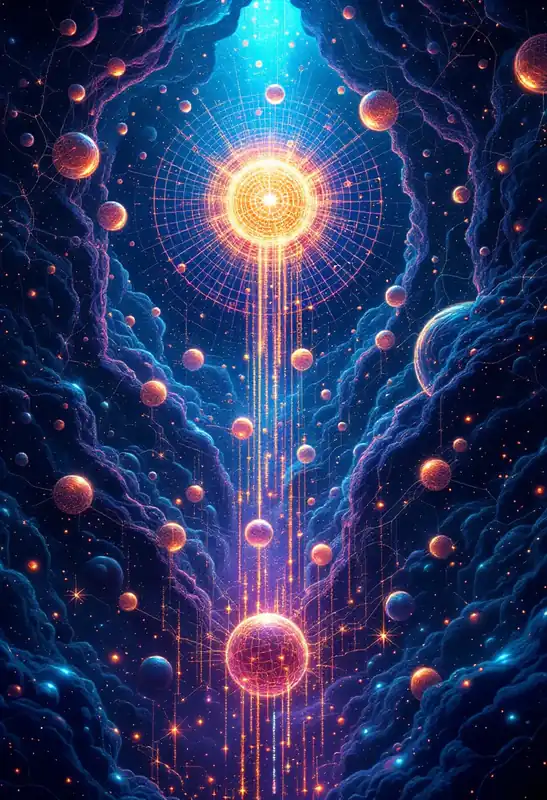
Pretrained Model
ML model that has been previously trained on a large dataset and can be fine-tuned or used as is for similar tasks or applications.
Generality: 860
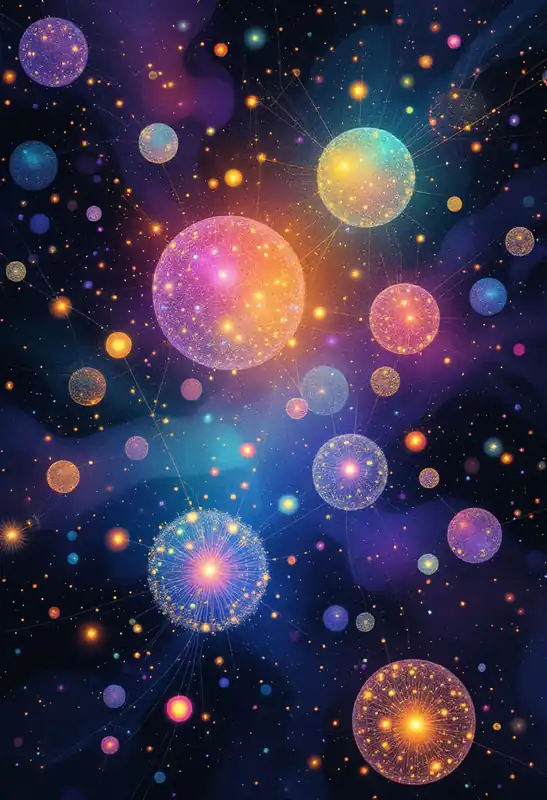
Latent Space
Abstract, multi-dimensional representation of data where similar items are mapped close together, commonly used in ML and AI models.
Generality: 805
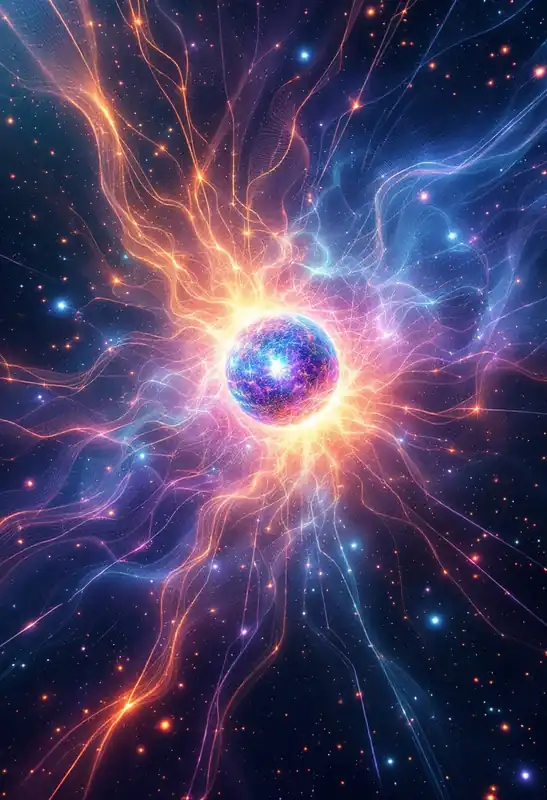
Embedding Space
Mathematical representation where high-dimensional vectors of data points, such as text, images, or other complex data types, are transformed into a lower-dimensional space that captures their essential properties.
Generality: 700
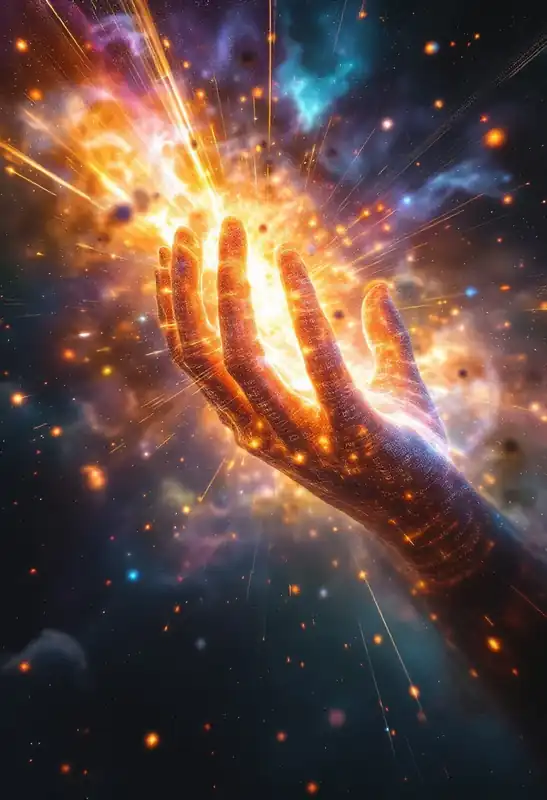
Gradient Clipping
A technique used to mitigate the exploding gradient problem during the training of neural networks by capping gradients to a specified value range.
Generality: 625
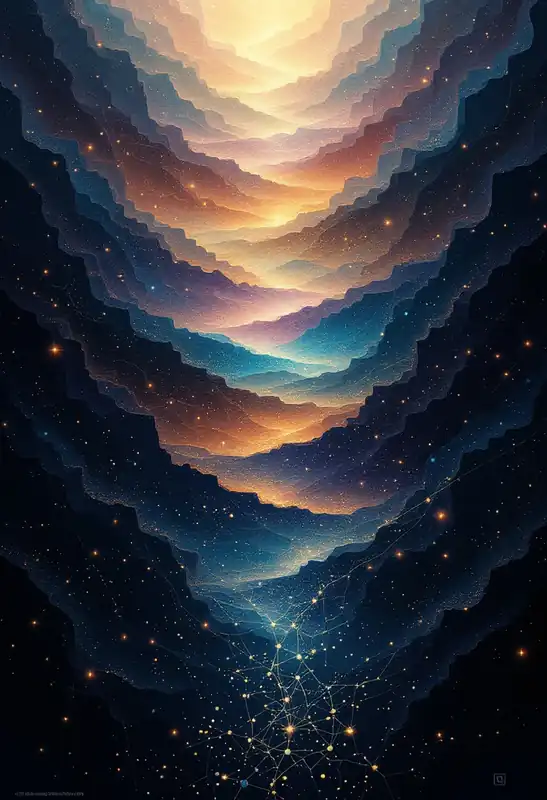
Model Layer
Discrete level in a neural network where specific computations or transformations are applied to the input data, progressively abstracting and refining the information as it moves through the network.
Generality: 805
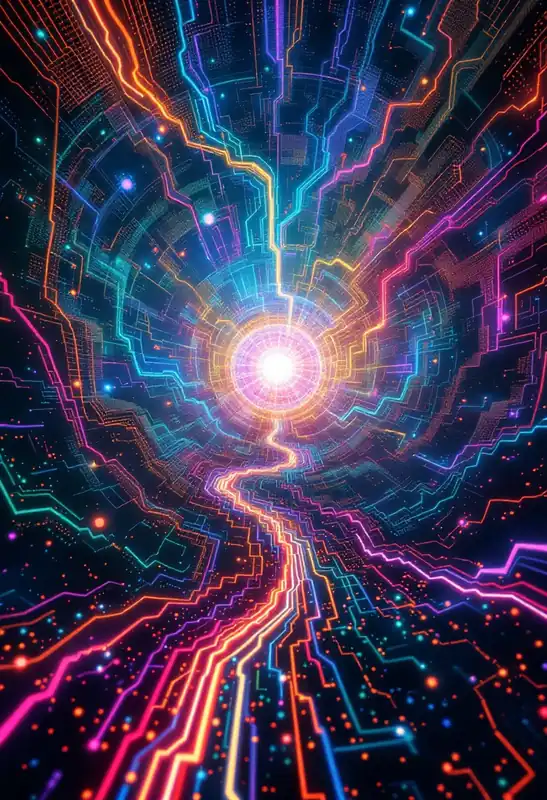
Adversarial Instructions
Inputs designed to deceive AI models into making incorrect predictions or decisions, highlighting vulnerabilities in their learning algorithms.
Generality: 740
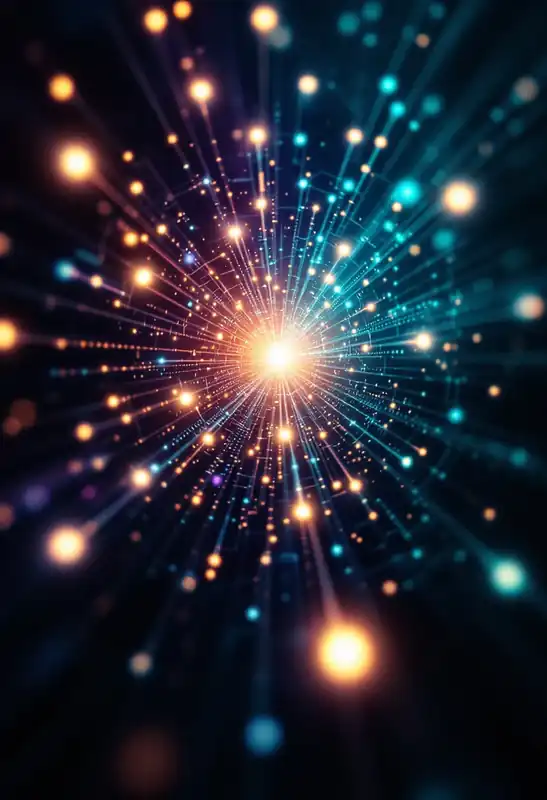
Attention Matrix
Component in attention mechanisms of neural networks that determines the importance of each element in a sequence relative to others, allowing the model to focus on relevant parts of the input when generating outputs.
Generality: 735
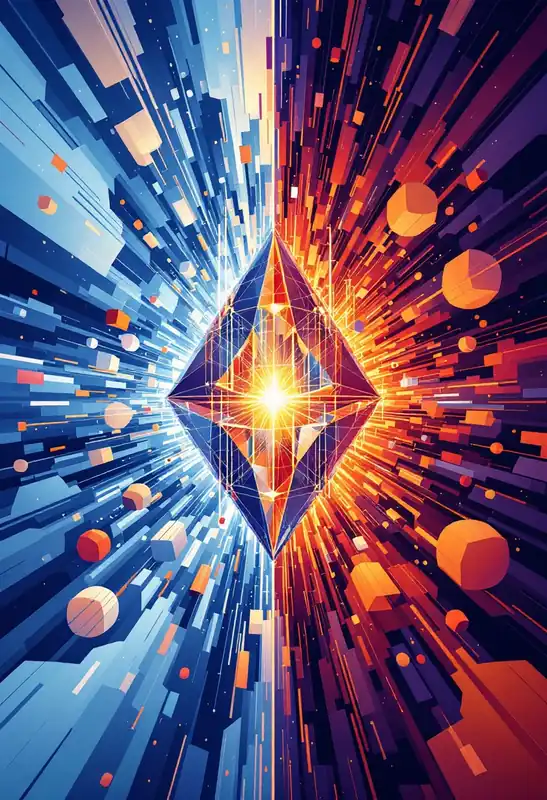
Discriminator
Model that determines the likelihood of a given input being real or fake, typically used in generative adversarial networks (GANs).
Generality: 815
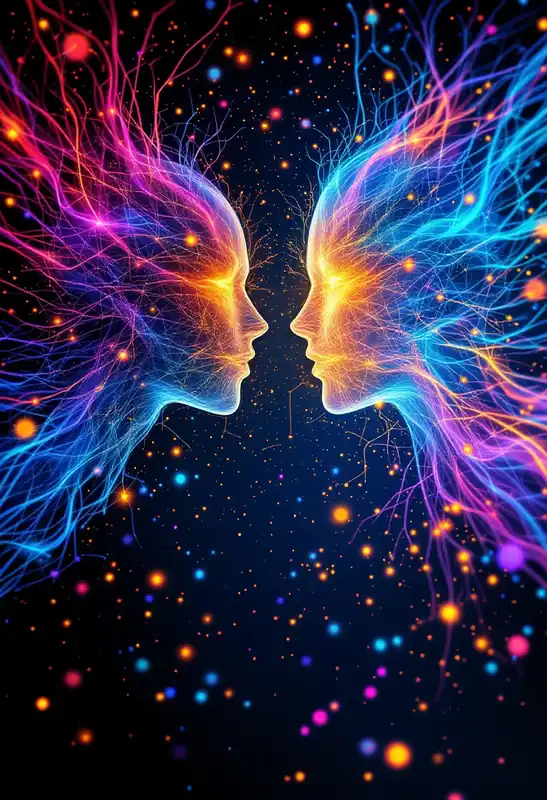
GAN
Generative Adversarial Network
Generative Adversarial Network
Class of AI algorithms used in unsupervised ML, implemented by a system of two neural networks contesting with each other in a game.
Generality: 865
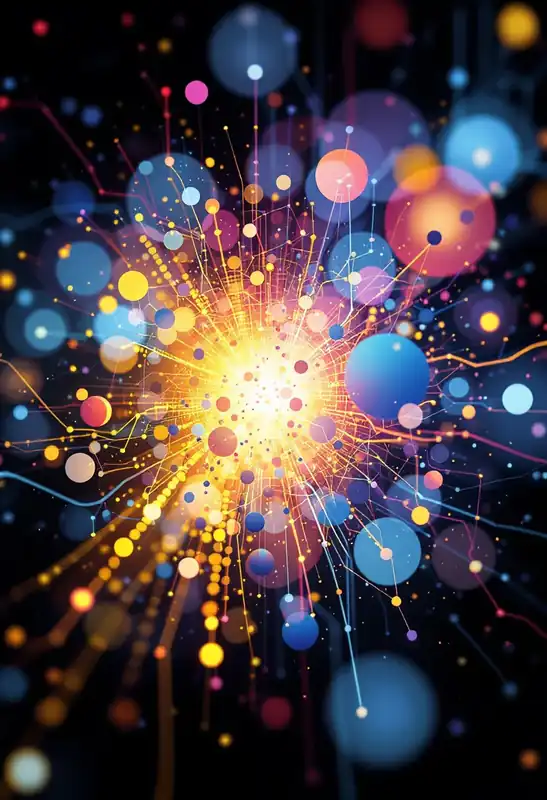
Attention Mechanisms
Dynamically prioritize certain parts of input data over others, enabling models to focus on relevant information when processing complex data sequences.
Generality: 830
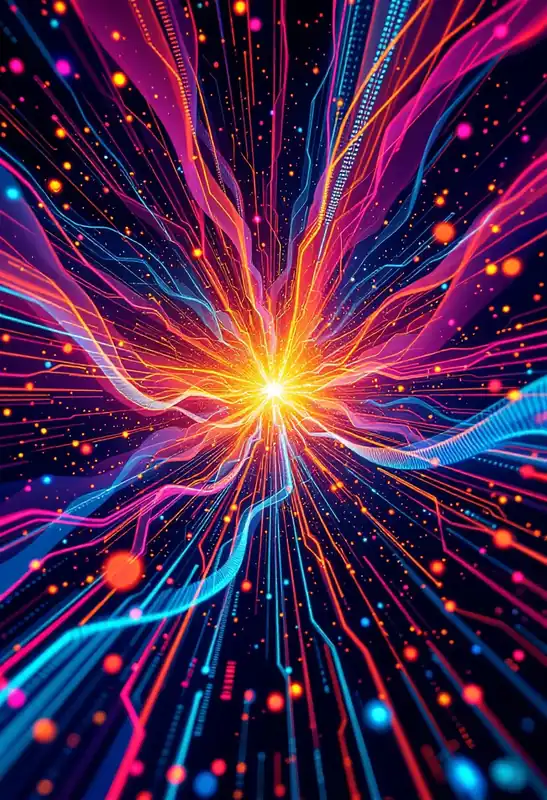
Attention
Refers to mechanisms that allow models to dynamically focus on specific parts of input data, enhancing the relevance and context-awareness of the processing.
Generality: 870
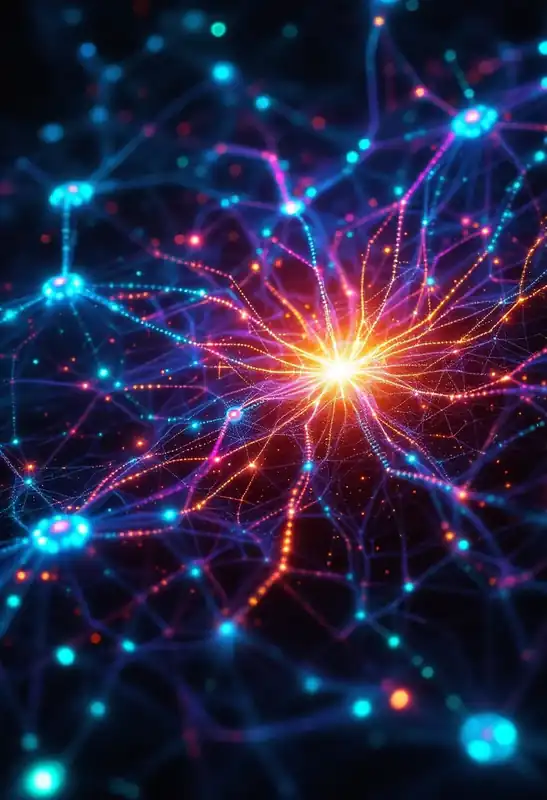
Attention Seeking
A behavior exhibited by neural networks, where they dynamically focus computational resources on important parts of the input, enhancing learning and performance.
Generality: 830
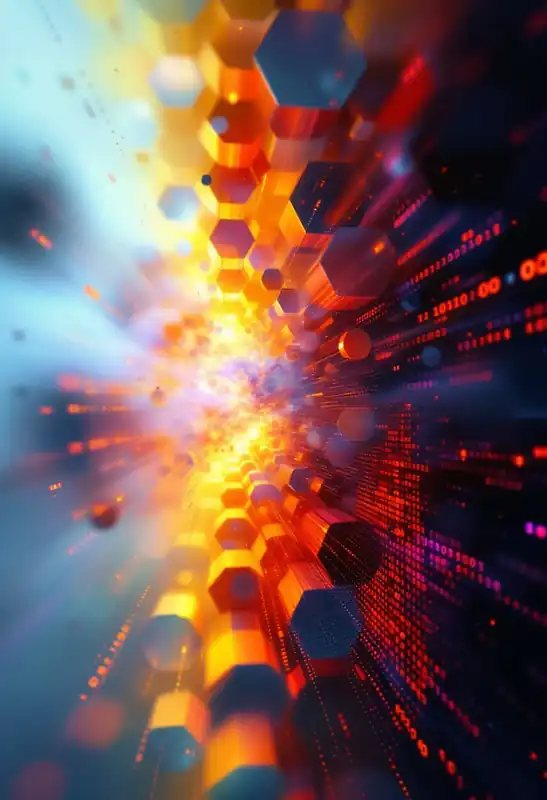
Mode Collapse
Phenomenon in Generative Adversarial Networks (GANs) where the generator produces limited, highly similar outputs, ignoring the diversity of the target data distribution.
Generality: 375
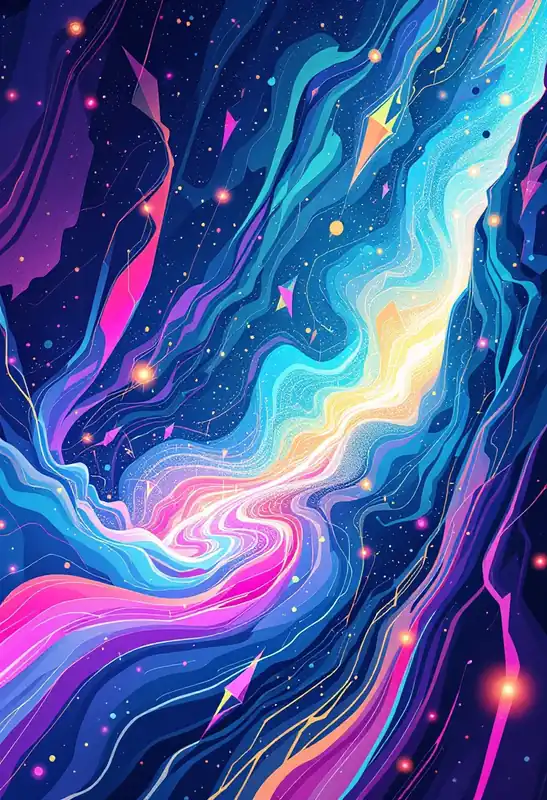
End-to-End Learning
ML approach where a system is trained to directly map input data to the desired output, minimizing the need for manual feature engineering.
Generality: 800
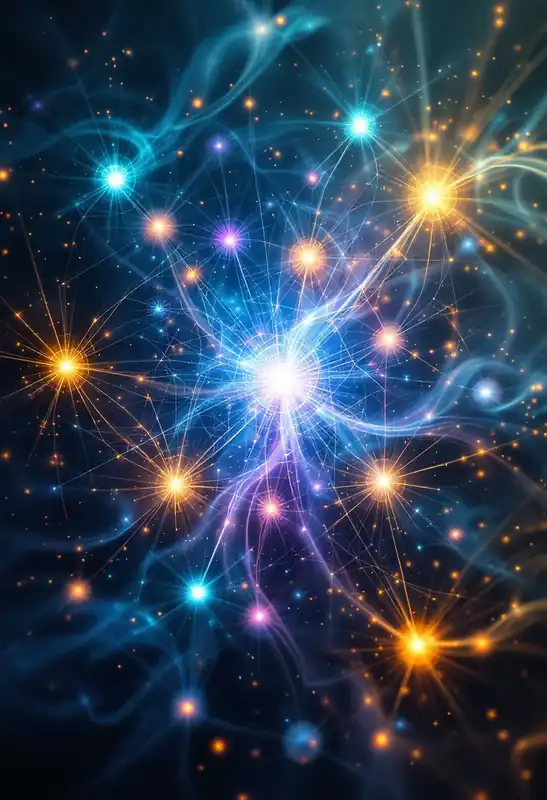
Conditional Generation
Process where models produce output based on specified conditions or constraints.
Generality: 830
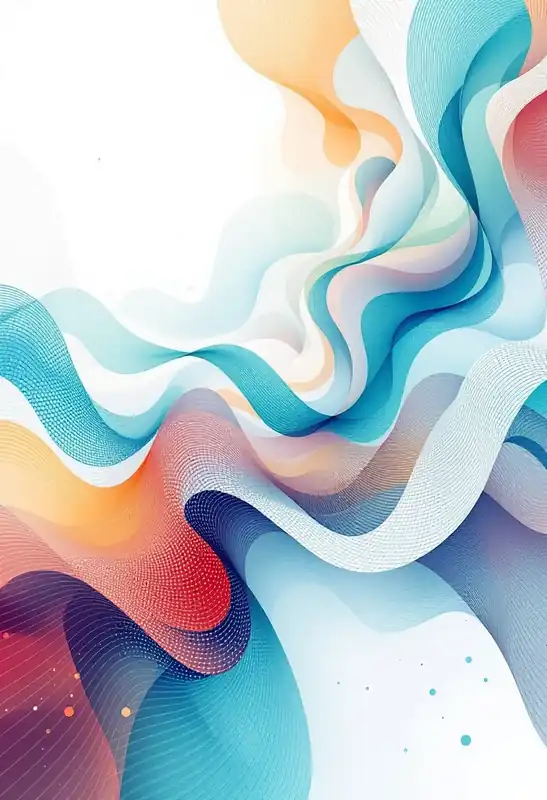
Sequence Model
Model designed to process and predict sequences of data, such as time series, text, or biological sequences.
Generality: 830
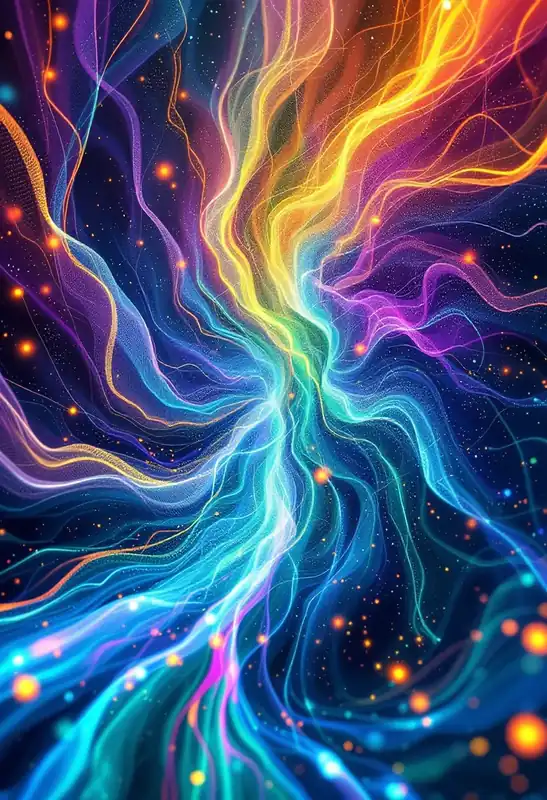
Generative AI
Subset of AI technologies that can generate new content, ranging from text and images to music and code, based on learned patterns and data.
Generality: 830
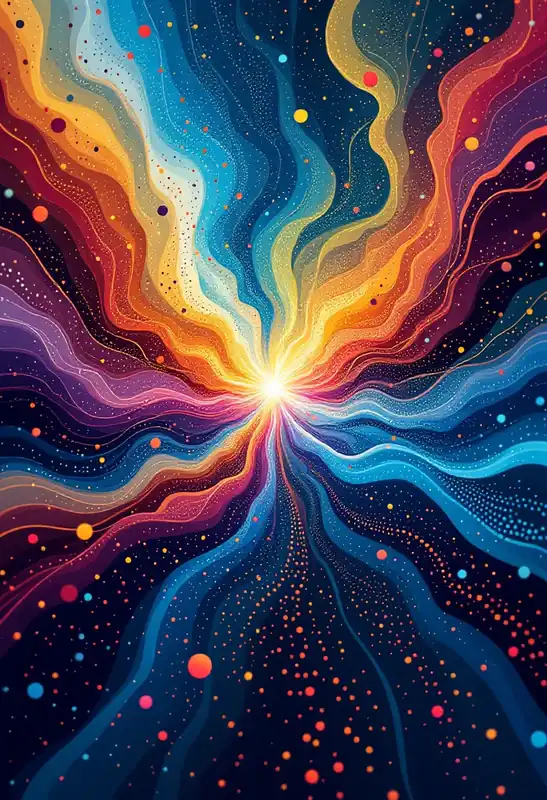
Recognition Model
Element of AI that identifies patterns and features in data through learning processes.
Generality: 790
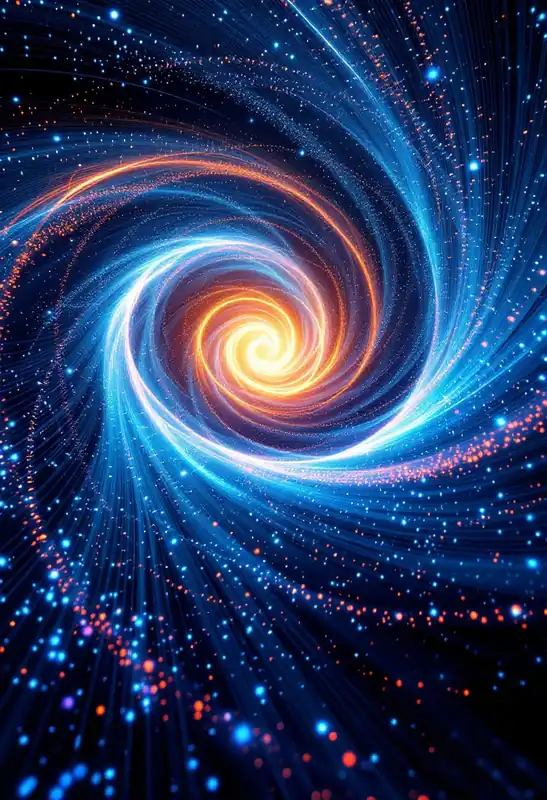
Autoregressive Sequence Generator
A predictive model harnessed in AI tasks, particularly involving times series, which leverages its own prior outputs as inputs in subsequent predictions.
Generality: 650
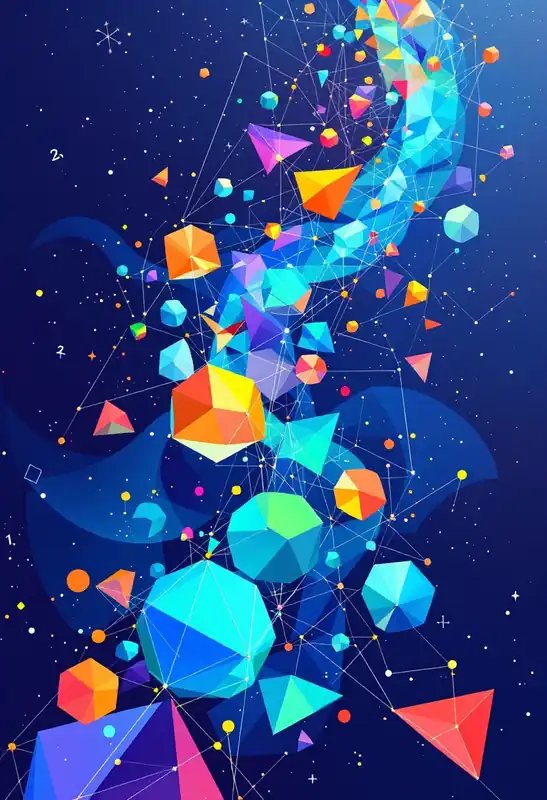
Sequential Models
Type of data models in AI where the arrangement of data points or events adhere to a specific order for predictive analysis and pattern recognition.
Generality: 815
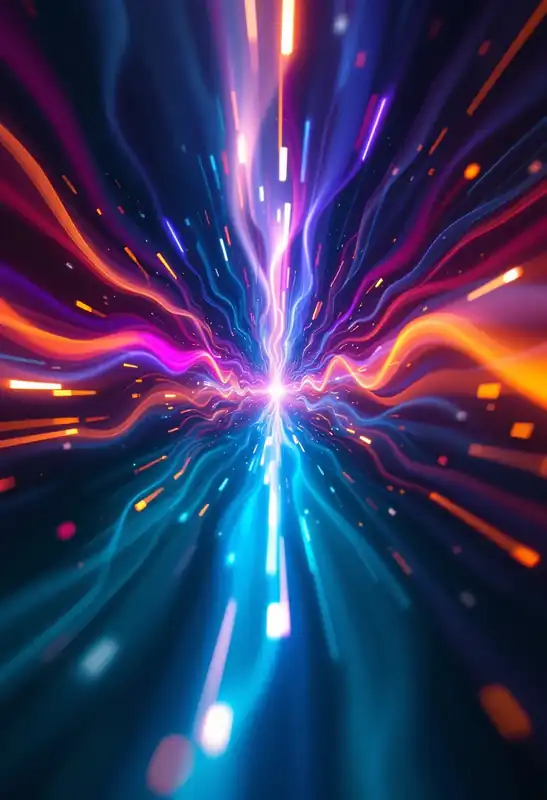
Convergence
The point at which an algorithm or learning process stabilizes, reaching a state where further iterations or data input do not significantly alter its outcome.
Generality: 845
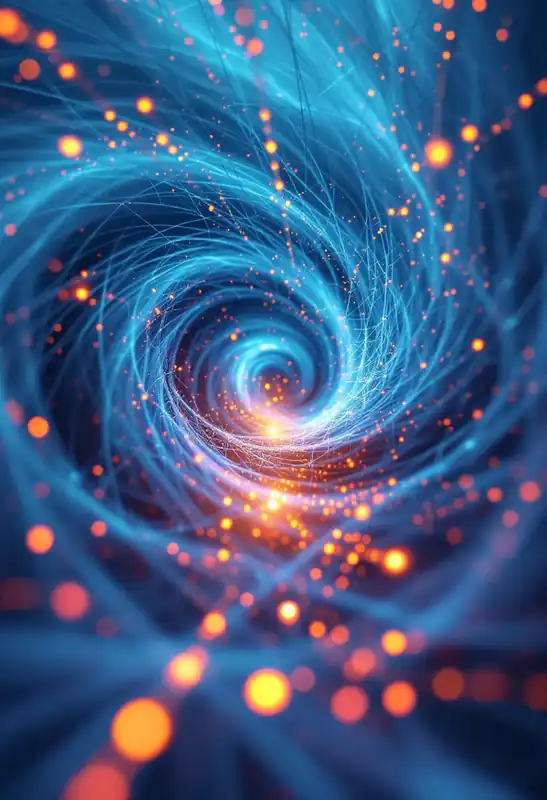
Overparameterized
ML model that has more parameters than the number of data points available for training.
Generality: 555
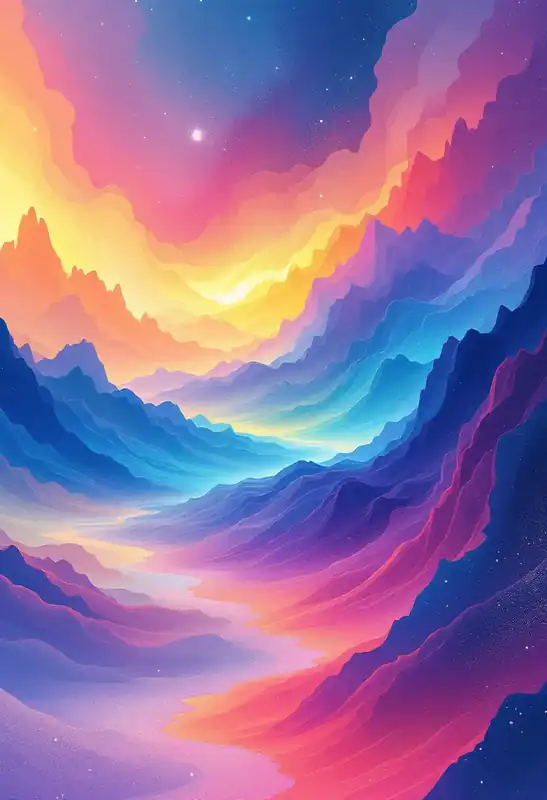
Loss Landscape
The topographical representation of a neural network's loss function showcasing the variations in loss values across different parameter settings.
Generality: 500
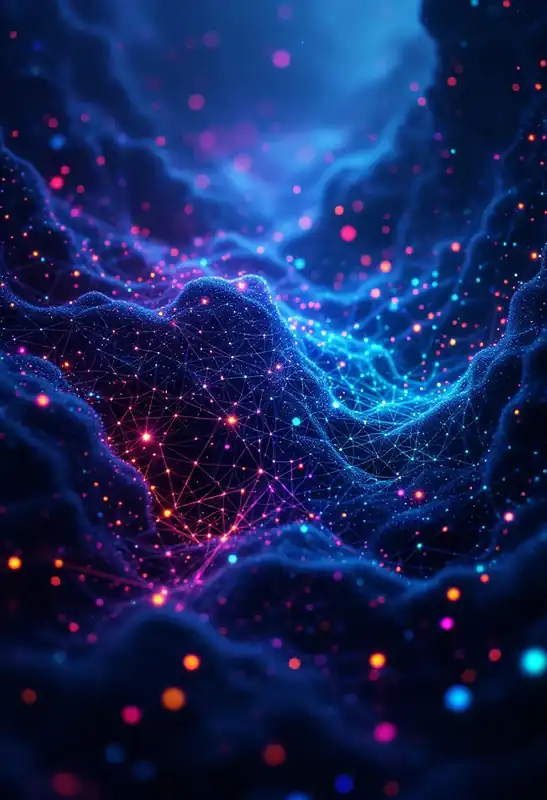
Attention Network
Type of neural network that dynamically focuses on specific parts of the input data, enhancing the performance of tasks like language translation, image recognition, and more.
Generality: 830
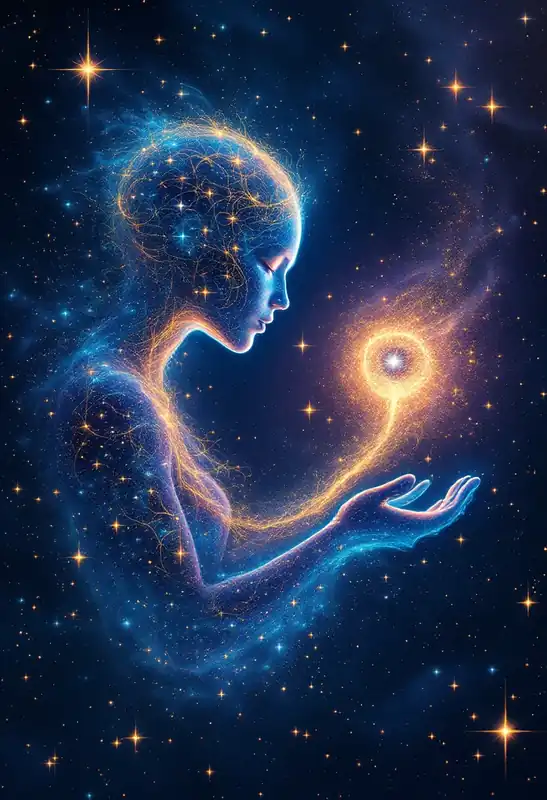
Teacher Model
Pre-trained, high-performing model that guides the training of a simpler, student model, often in the context of knowledge distillation.
Generality: 561
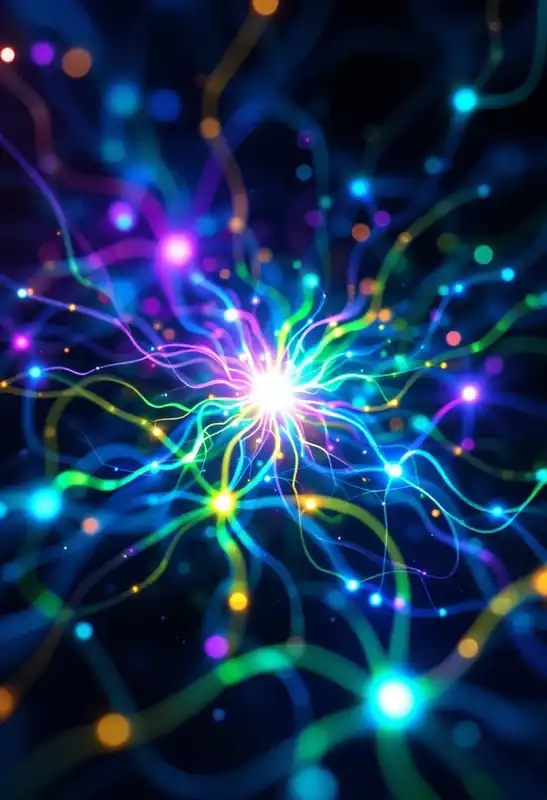
Activation Data
Intermediate outputs produced by neurons in a neural network when processing input data, which are used to evaluate and update the network during training.
Generality: 575
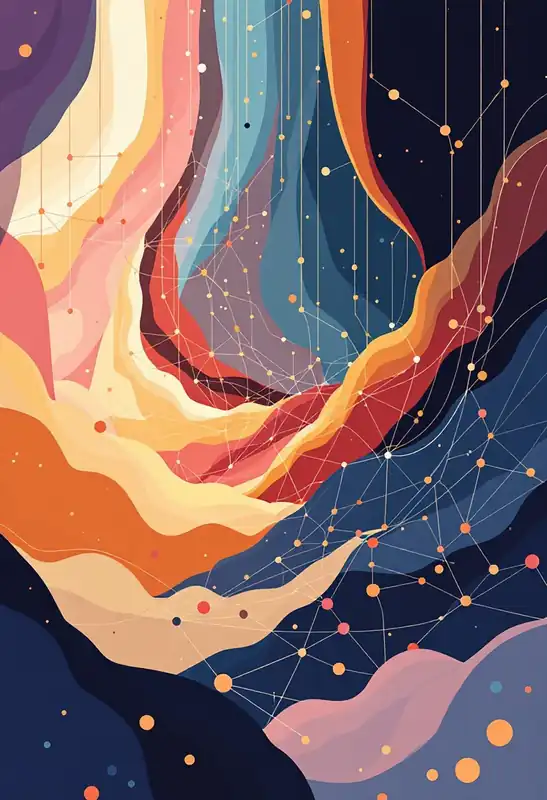
Variance Scaling
Variance scaling is a technique used in machine learning to ensure weights of layers are initialized in a way that maintains consistent variance of activations throughout a neural network.
Generality: 525
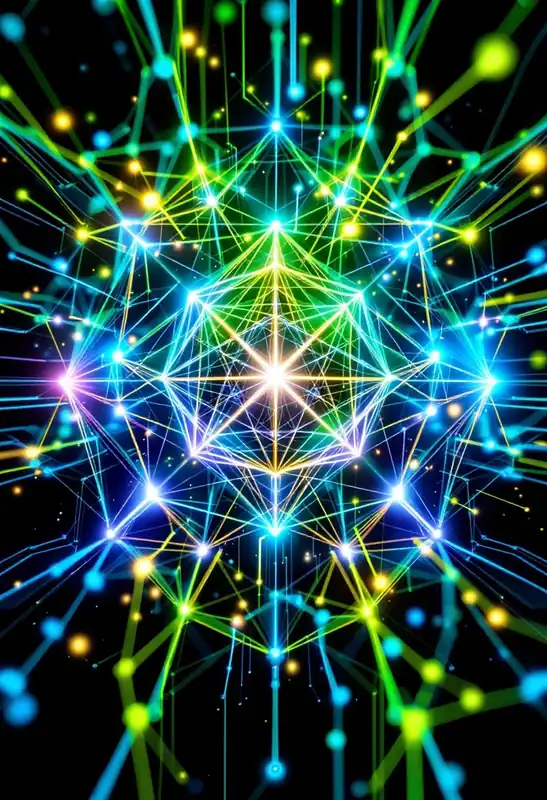
GLU
Gated Linear Unit
Gated Linear Unit
Neural network component that uses a gating mechanism to control information flow, improving model efficiency and performance.
Generality: 665
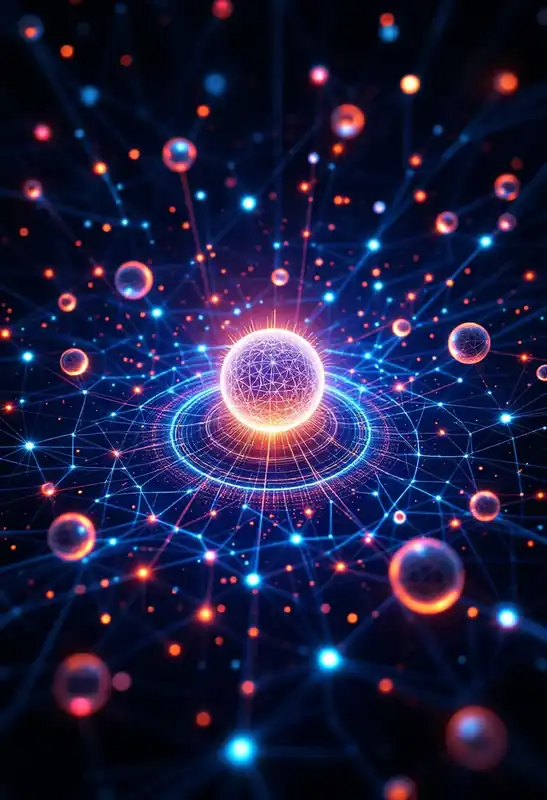
Federated Learning
ML approach enabling models to be trained across multiple decentralized devices or servers holding local data samples, without exchanging them.
Generality: 805
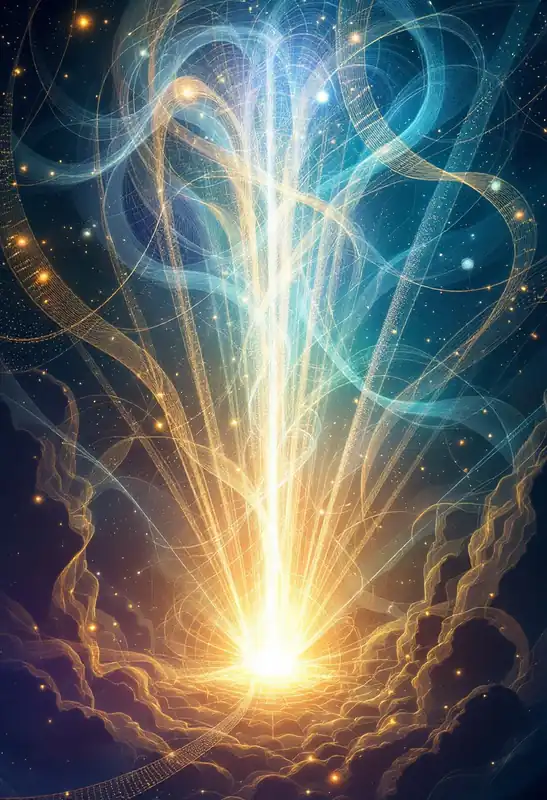
Inference Acceleration
Methods and hardware optimizations employed to increase the speed and efficiency of the inference process in machine learning models, particularly neural networks.
Generality: 775
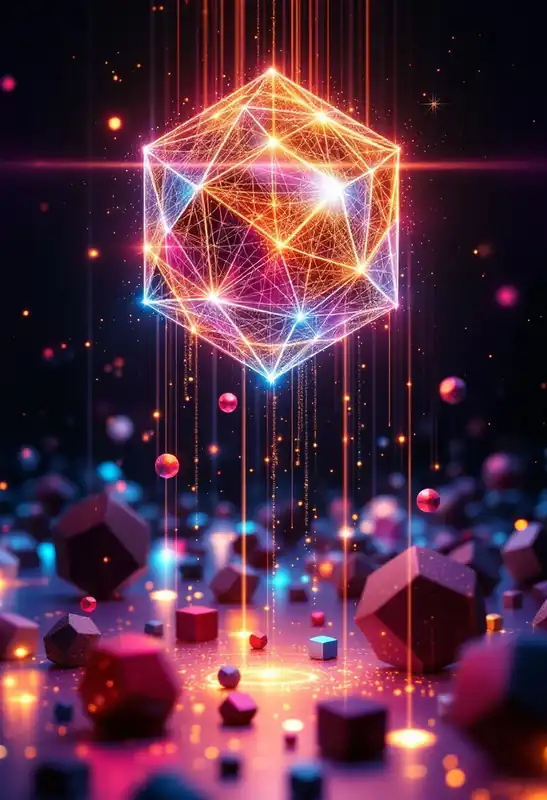
Federated Training
Decentralized machine learning approach where multiple devices or nodes collaboratively train a shared model while keeping their data localized, rather than aggregating it centrally.
Generality: 805
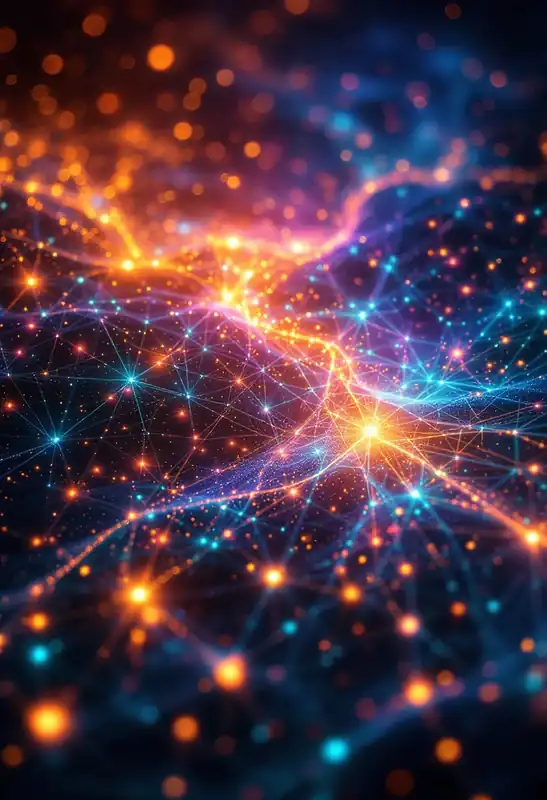
Attention Pattern
Mechanism that selectively focuses on certain parts of the input data to improve processing efficiency and performance outcomes.
Generality: 820
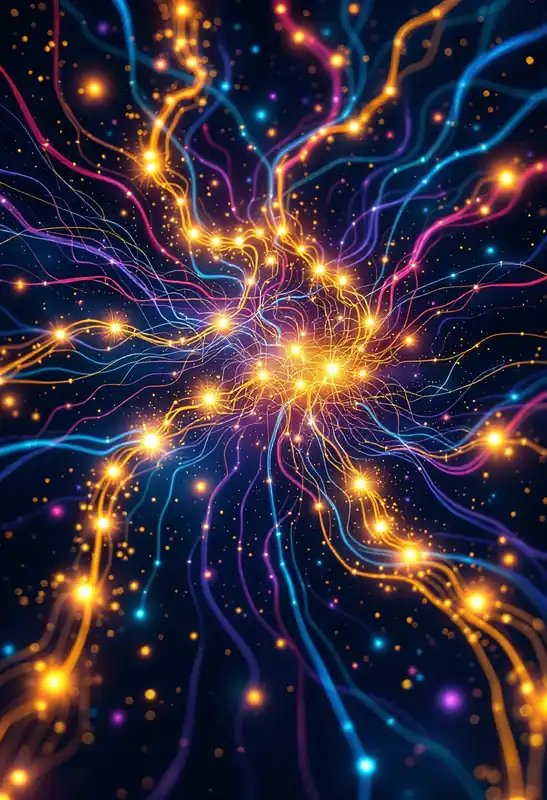
Expressive Hidden States
internal representations within a neural network that effectively capture and encode complex patterns and dependencies in the input data.
Generality: 695
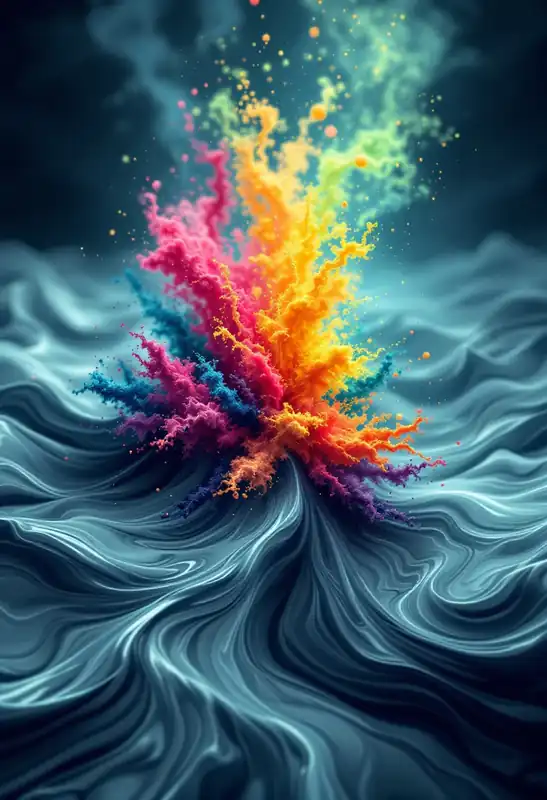
Out of Distribution
Data that differs significantly from the training data used to train a machine learning model, leading to unreliable or inaccurate predictions.
Generality: 675
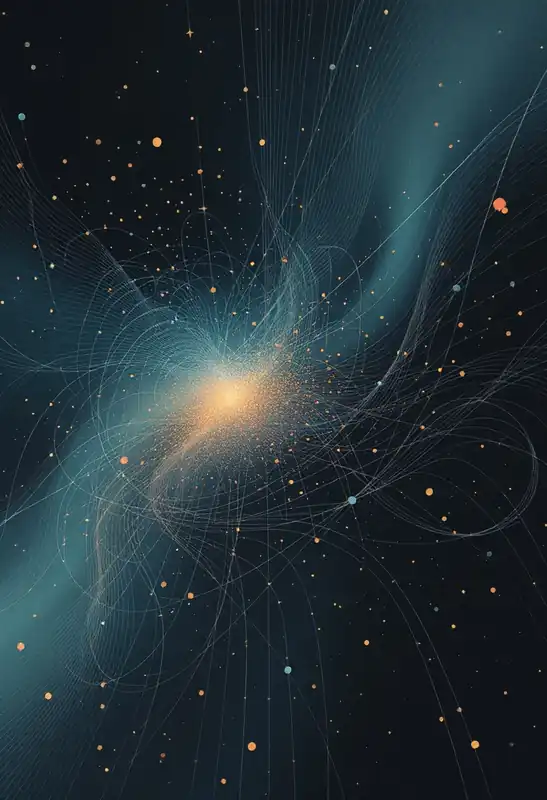
Point-wise Feedforward Network
Neural network layer that applies a series of linear and non-linear transformations to each position (or
Generality: 625
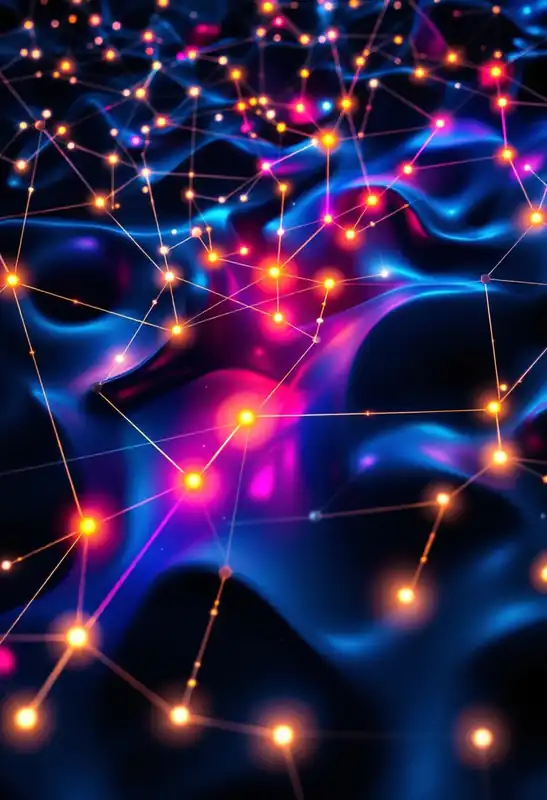
Masking
Technique used in NLP models to prevent future input tokens from influencing the prediction of current tokens.
Generality: 639
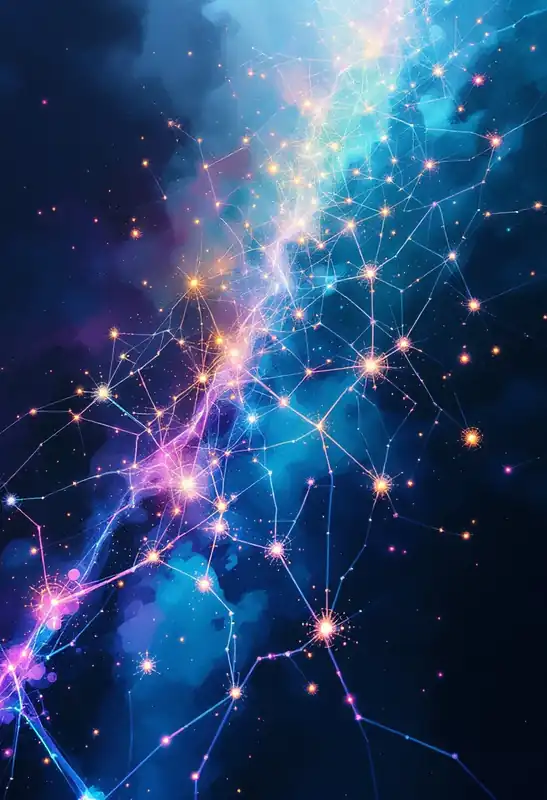
Ablation
Method where components of a neural network are systematically removed or altered to study their impact on the model's performance.
Generality: 650
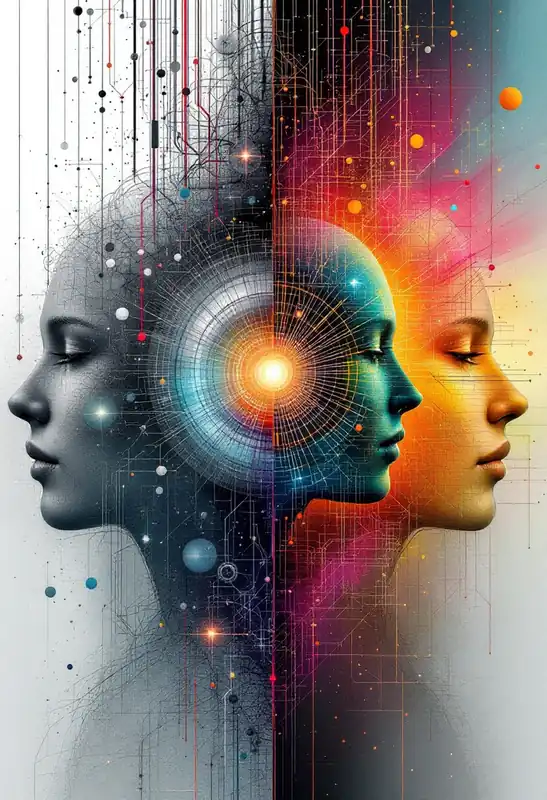
Zero-shot Capability
The ability of AI models to perform tasks or make predictions on new types of data that they have not encountered during training, without needing any example-specific fine-tuning.
Generality: 775
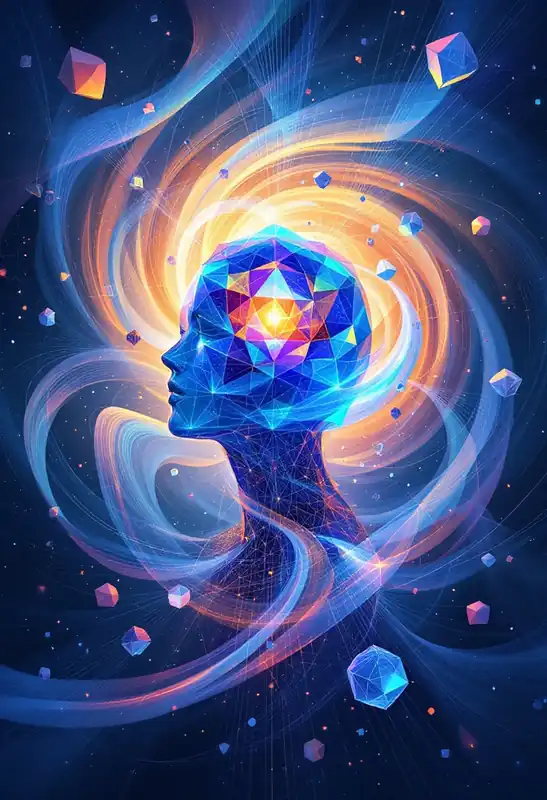
SSL
Self-Supervised Learning
Self-Supervised Learning
Type of ML where the system learns to predict part of its input from other parts, using its own data structure as supervision.
Generality: 815
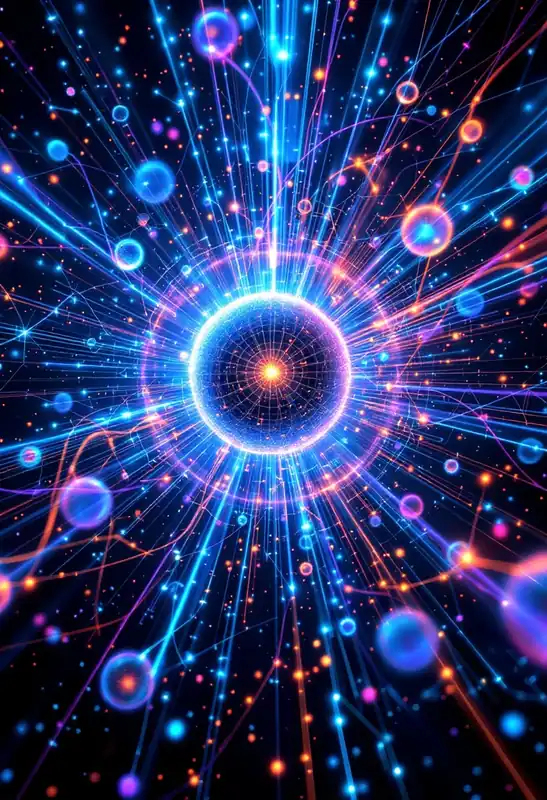
LLM
Large Language Model
Large Language Model
Advanced AI systems trained on extensive datasets to understand, generate, and interpret human language.
Generality: 827
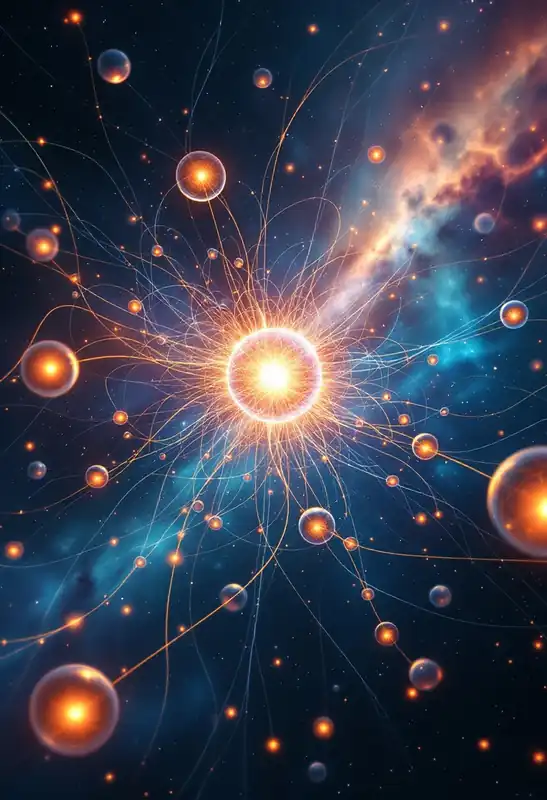
Next Token Prediction
Technique used in language modeling where the model predicts the following token based on the previous ones.
Generality: 735
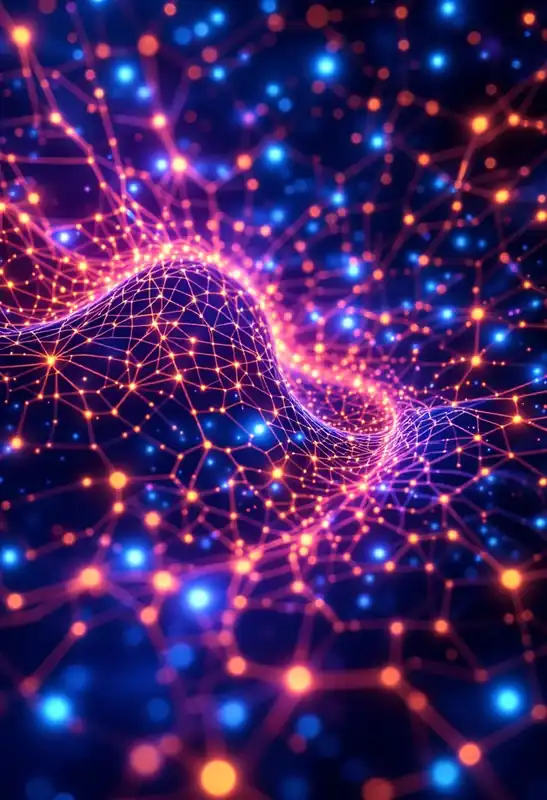
xLSTM
Extended form of Long Short-Term Memory (LSTM), integrating enhancements for scalability and efficiency in DL models.
Generality: 675
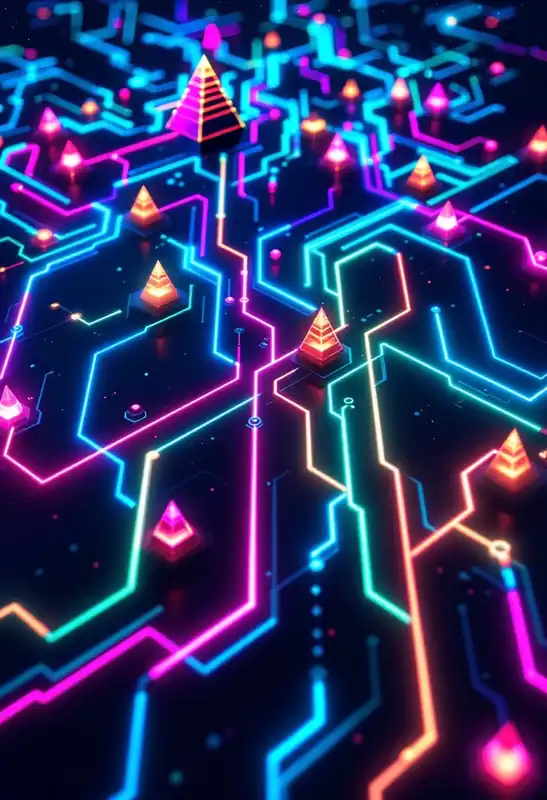
Base Model
Pre-trained AI model that serves as a starting point for further training or adaptation on specific tasks or datasets.
Generality: 790
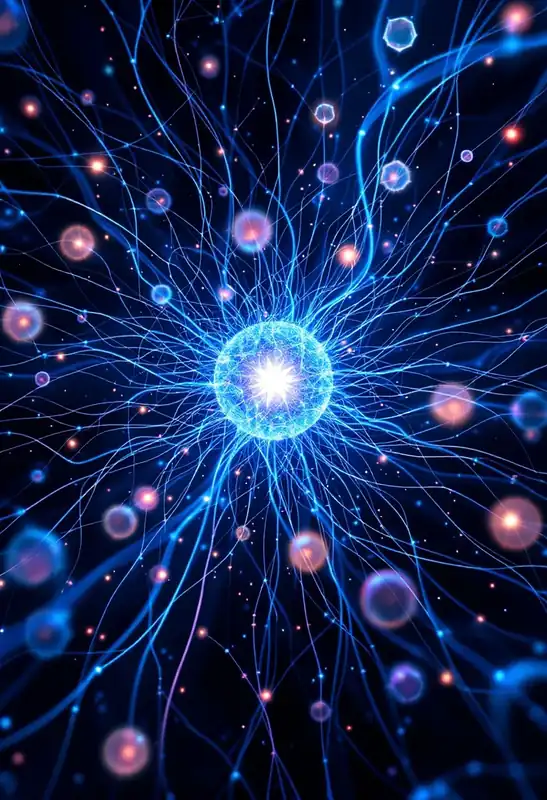
DLMs
Deep Language Models
Deep Language Models
Advanced ML models designed to understand, generate, and translate human language by leveraging DL techniques.
Generality: 874
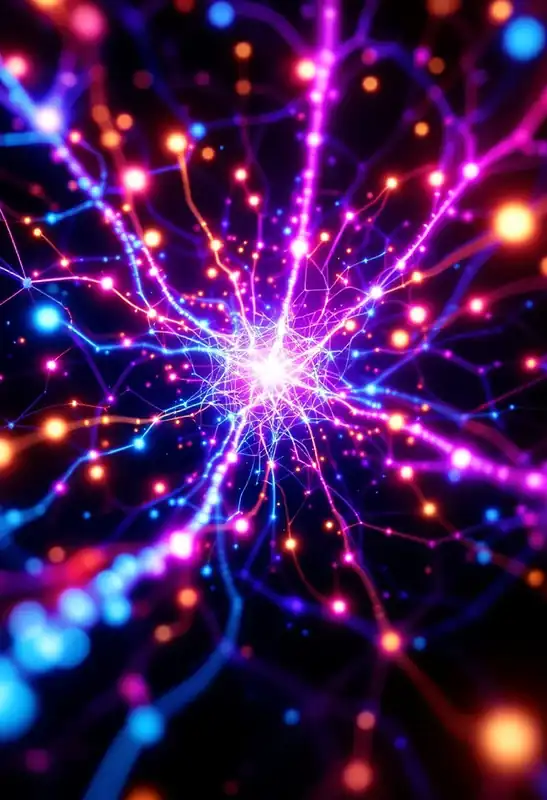
Self-Supervised Pretraining
ML approach where a model learns to predict parts of the input data from other parts without requiring labeled data, which is then fine-tuned on downstream tasks.
Generality: 725
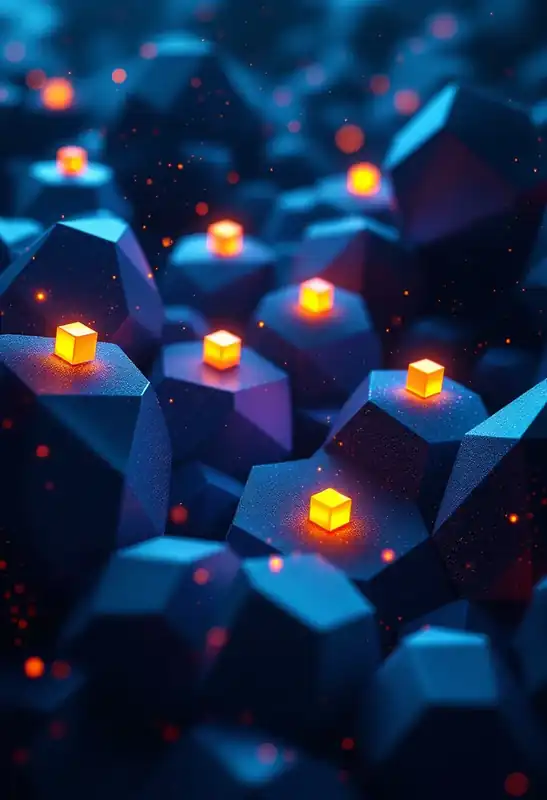
Adapter Layer
Neural network layer used to enable transfer learning by adding small, trainable modules to a pre-trained model, allowing it to adapt to new tasks with minimal additional training.
Generality: 625
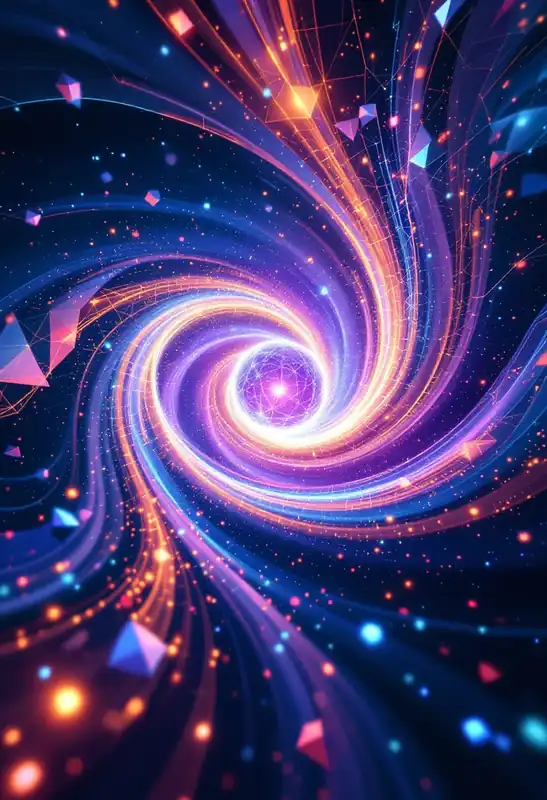
Continual Pre-Training
Process of incrementally training a pre-trained ML model on new data or tasks to update its knowledge without forgetting previously learned information.
Generality: 670
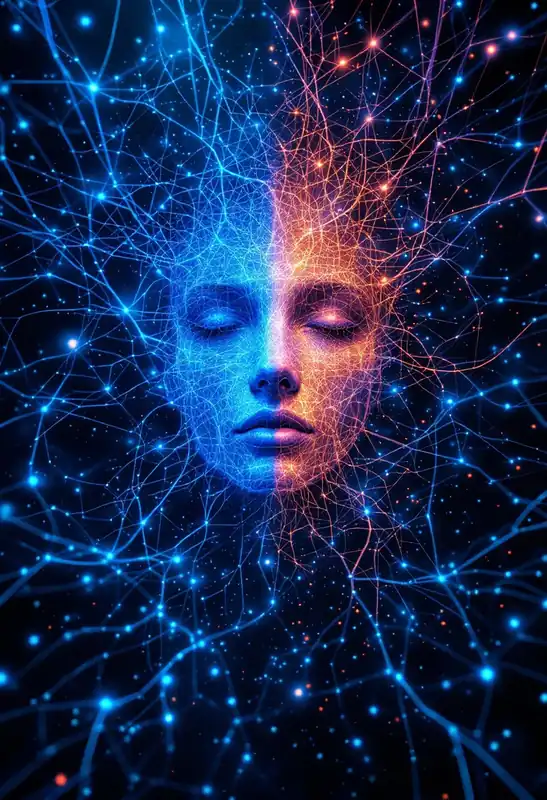
Post-Training
Techniques and adjustments applied to neural networks after their initial training phase to enhance performance, efficiency, or adaptability to new data or tasks.
Generality: 650
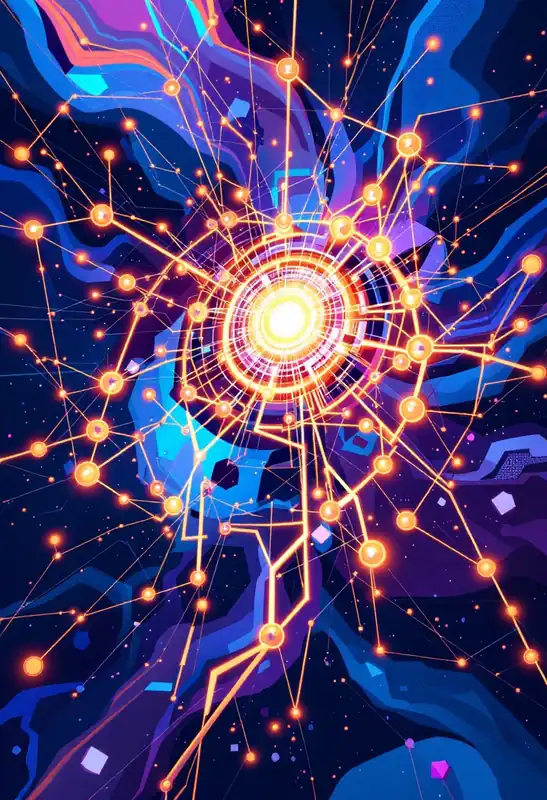
AMI
Advanced Machine Intelligence
Advanced Machine Intelligence
Refers to high-level AI systems possessing the capability to perform complex cognitive tasks with or without human-like reasoning.
Generality: 873
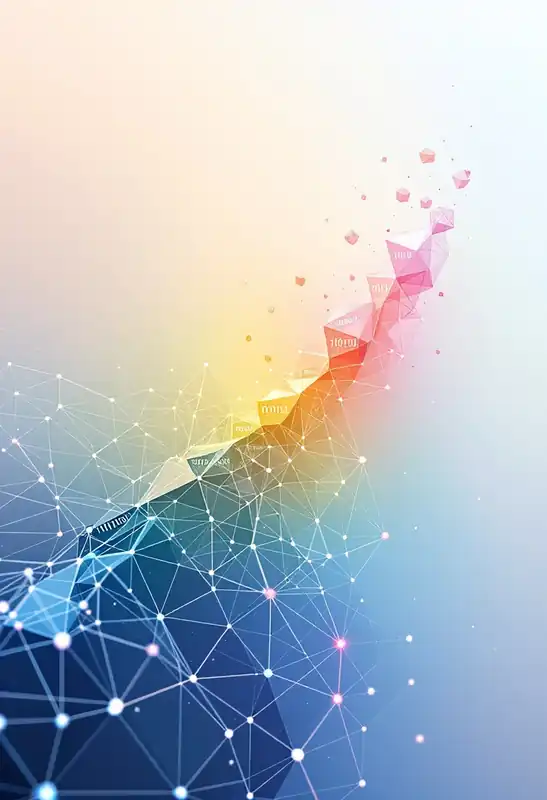
Scaling Laws
Mathematical relationships that describe how the performance of machine learning models, particularly deep learning models, improves as their size, the amount of data, or computational resources increases.
Generality: 835
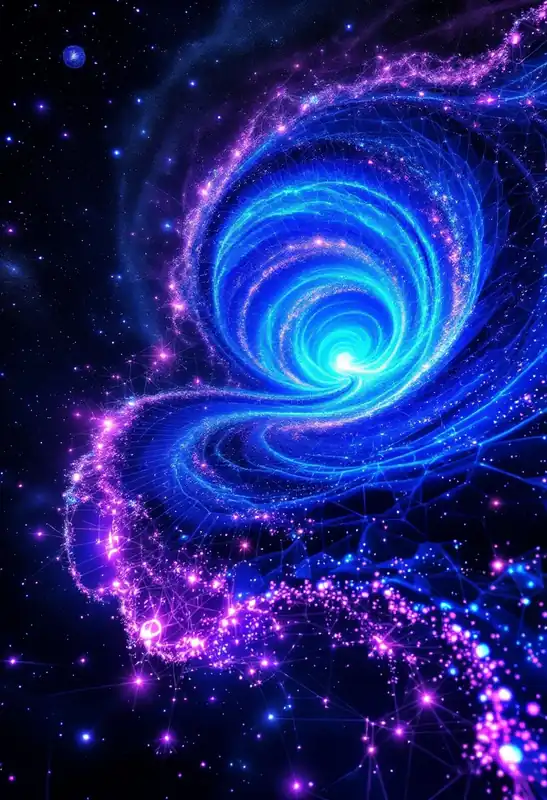
Scaling Hypothesis
Enlarging model size, data, and computational resources can consistently improve task performance up to very large scales.
Generality: 765
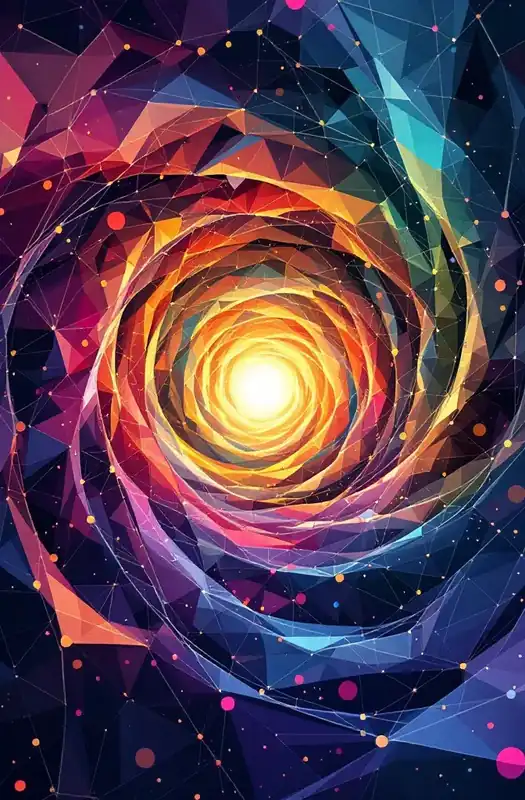
Generative Model
A type of AI model that learns to generate new data instances that mimic the training data distribution.
Generality: 840
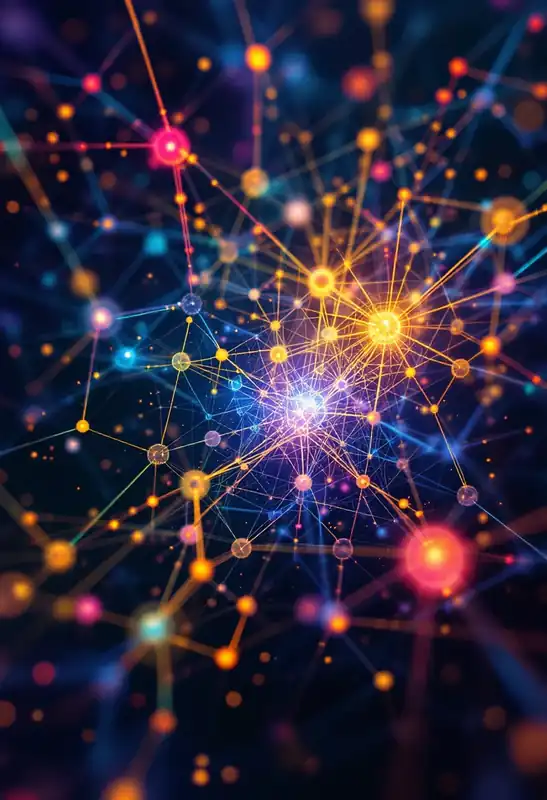
Parametric Knowledge
Information and patterns encoded within the parameters of a machine learning model, which are learned during the training process.
Generality: 849
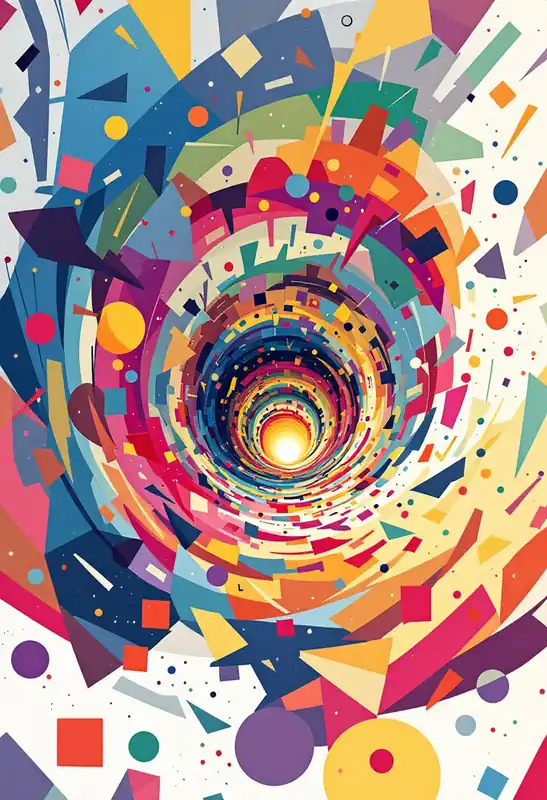
Model Collapse
Phenomenon where a ML model, particularly in unsupervised or generative learning, repeatedly produces identical or highly similar outputs despite varying inputs, leading to a loss of diversity in the generated data.
Generality: 650
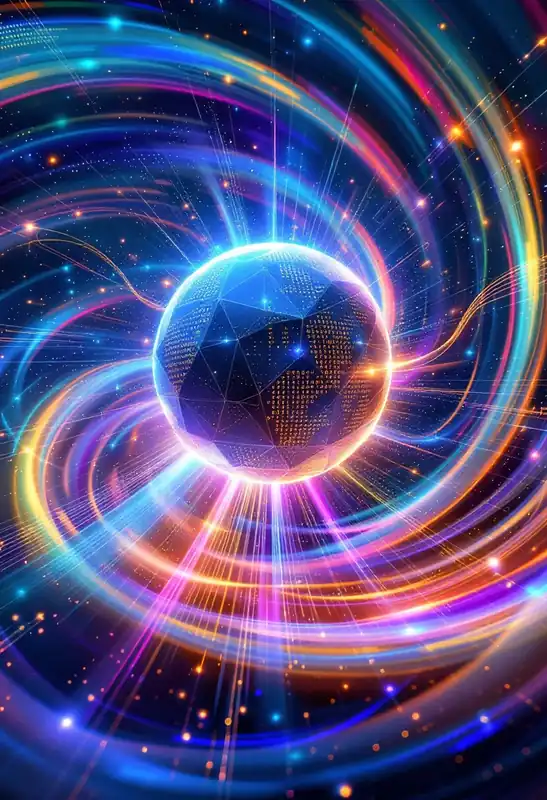
Transformative AI
AI systems capable of bringing about profound, large-scale changes in society, potentially altering the economy, governance, and even human life itself.
Generality: 825
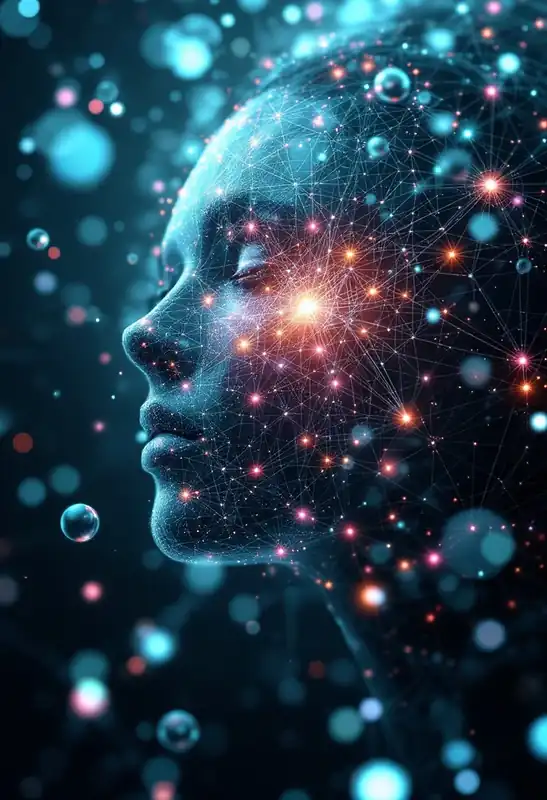
Foundation Model
Type of large-scale pre-trained model that can be adapted to a wide range of tasks without needing to be trained from scratch each time.
Generality: 835