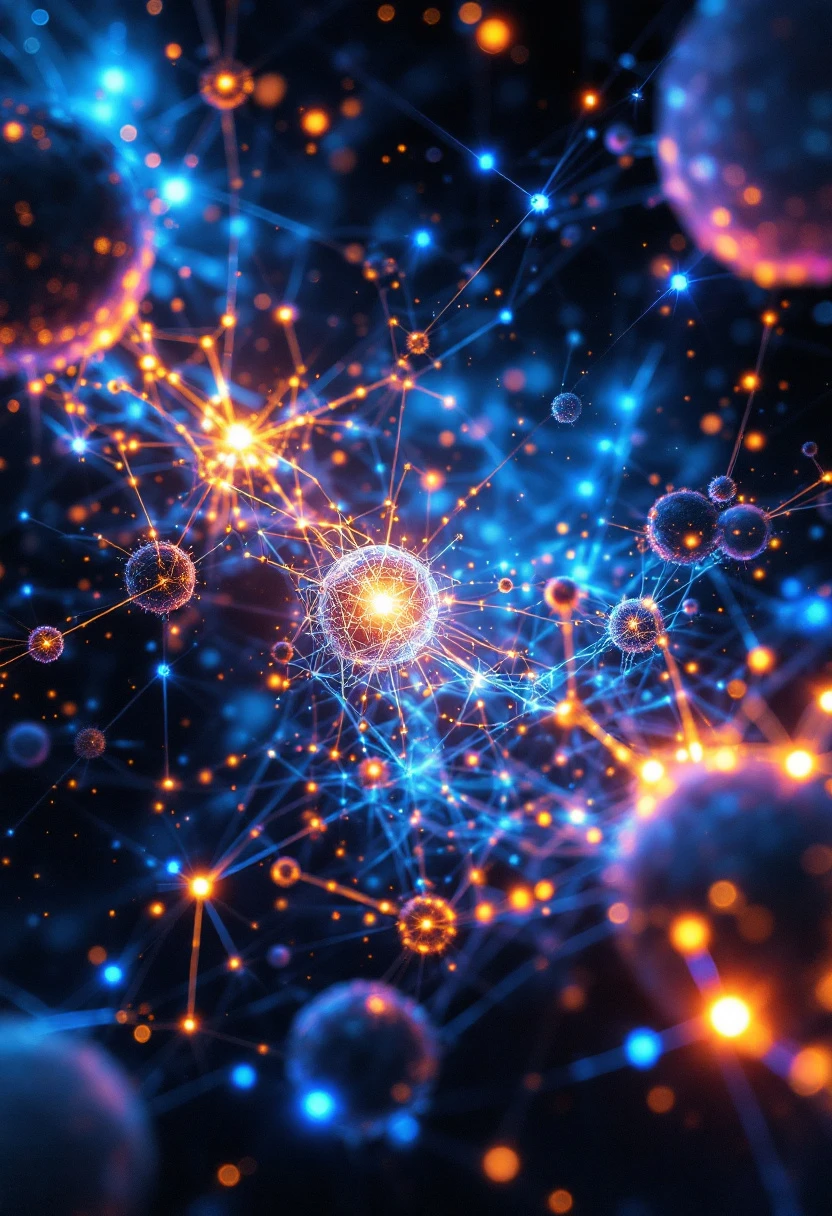
Neural Network
Computing system designed to simulate the way human brains analyze and process information, using a network of interconnected nodes that work together to solve specific problems.
Neural networks form the backbone of artificial intelligence, particularly in the field of deep learning. They are composed of layers of nodes, or "neurons," each of which connects to several other nodes in the subsequent layer, forming a web-like structure. The connections between these neurons are weighted by previous learning tasks and are adjusted as new data flows through the network, a process known as "training." Neural networks are adept at recognizing patterns and making predictions based on complex data inputs, making them highly valuable for tasks ranging from voice recognition and image classification to more complex decision-making tasks in autonomous vehicles and financial modeling.
The concept of the neural network has its roots in the 1940s, with early models developed by Warren McCulloch and Walter Pitts in 1943. The technology gained significant popularity in the 1980s when the backpropagation algorithm was popularized, which efficiently trained multi-layer neural networks.
Warren McCulloch and Walter Pitts were pivotal in laying the foundational theory of neural networks with their logical calculus of ideas immanent in nervous activity. Frank Rosenblatt further contributed by creating the perceptron, an early neural network, in 1958. The resurgence of interest in neural networks in the 1980s is largely credited to researchers like Geoffrey Hinton, who helped refine the backpropagation training algorithm, making it feasible to train deep neural networks effectively.
Explainer
Functional AGI Visualization
Observe how AGI systems process information
Understanding the AI Connection
This visualization represents a simplified version of how modern AI systems process information:
- Input Nodes (1-3): Represent raw data input, similar to how AI systems receive data from various sources (text, images, sensors).
- Reasoning Node: Simulates how AI analyzes patterns and applies logical rules to understand relationships in data.
- Learning Node: Represents the system's ability to adapt and improve from experience, similar to how neural networks adjust their weights during training.
- Output Node: Shows the final result after processing, which could be a decision, prediction, or generated content.
The glowing connections show how information flows through the system, similar to how neural networks propagate and transform data through multiple layers to arrive at meaningful outputs.