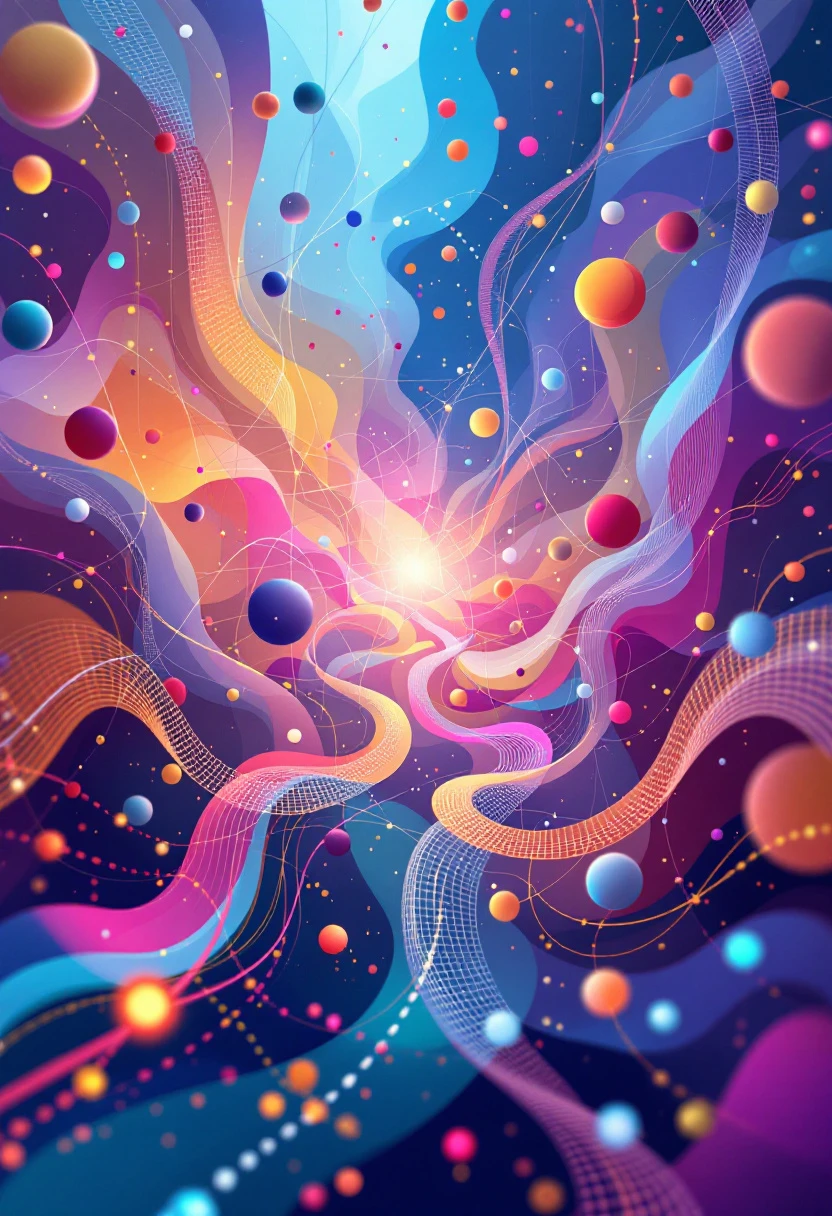
Pattern Recognition
The identification and classification of patterns in data using computational algorithms, essential for enabling machines to interpret, learn from, and make decisions based on complex datasets.
Pattern recognition is a cornerstone of AI and involves the use of algorithms to identify regularities in data. This capability allows AI systems to interpret and classify inputs from various forms whether visual, auditory, or otherwise structured. It leverages techniques from statistics, ML, and neural networks to discern patterns from noise, forming the backbone of applications like facial recognition, speech analysis, and anomaly detection. Significantly, pattern recognition systems can enhance over time through learning, making them adaptive to new patterns, evolving user inputs, or changing environments, which is critical for the advancement of intelligent systems.
The first notable use of pattern recognition dates back to the 1960s, gaining particular prominence with the advent of increased computational power and advances in statistical methods during the late 1980s and early 1990s, which allowed for more sophisticated and scalable pattern recognition techniques.
Pioneers in the field of pattern recognition include the likes of Azriel Rosenfeld, who significantly contributed to the development of image analysis and computer vision, and Satoshi Watanabe, known for laying foundational work in pattern recognition theory, along with many contributions from groups such as the Pattern Recognition Society, whose work collectively advanced the broader application of these technologies.