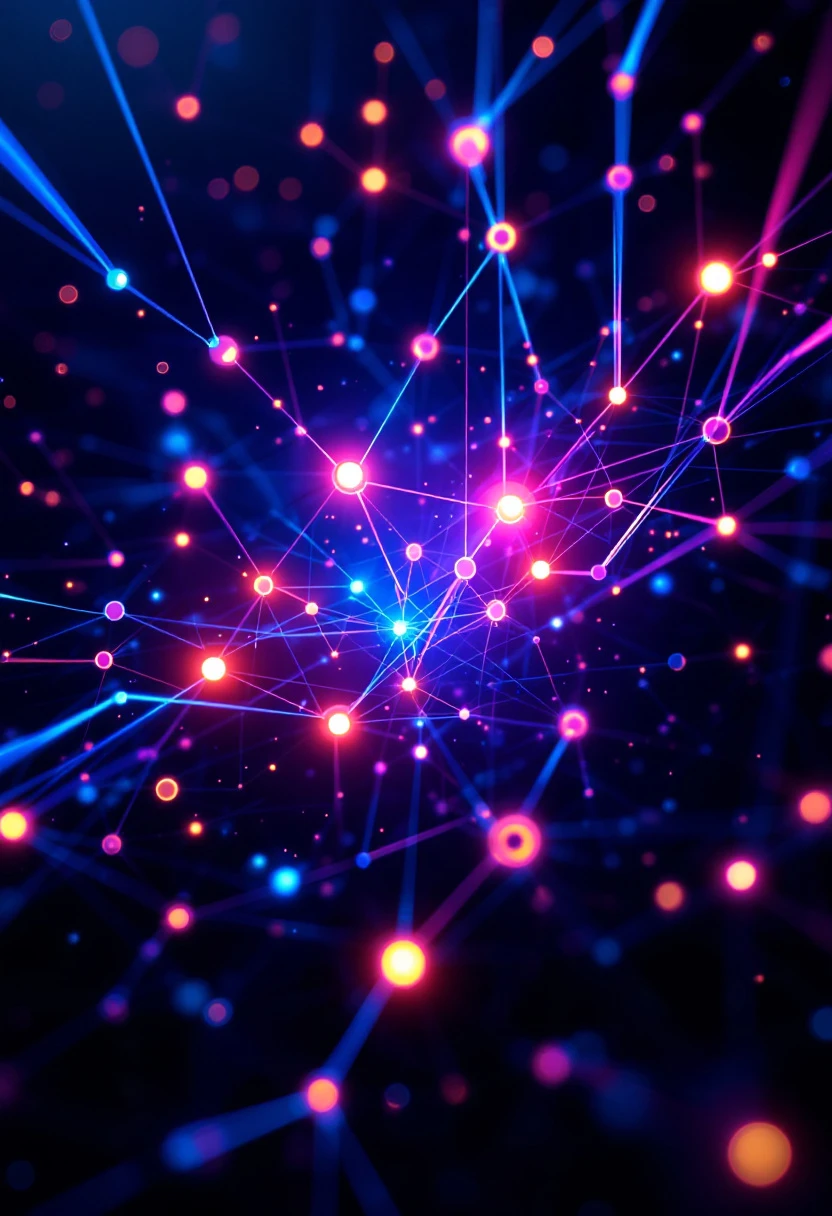
Principle of Rationality
The assumption that an AI agent will act in a way that maximizes its expected utility based on its understanding of the environment.
The Principle of Rationality in AI posits that an agent is rational when it acts to achieve the best outcome given its pre-existing model of the environment, available information, and computational capabilities. This principle serves as a foundational guideline for designing AI systems, aiming to ensure that agents behave optimally by following policies that maximize expected reward or utility. It is closely associated with decision theory and game theory in understanding and modeling decision-making processes. The principle plays a crucial role in the development of AI algorithms, particularly in areas like reinforcement learning, where agents learn optimal behaviors through interactions with their environment and feedback from reward signals.
The Principle of Rationality's modern conceptualization dates back to the mid-20th century. It became widely recognized in AI research during the 1970s as academics began adapting decision-theoretic frameworks to AI problem-solving and agent-based modeling.
Key contributors to the development of the Principle of Rationality include researchers like John von Neumann, whose work on game theory laid early groundwork, and Herbert Simon, who integrated human decision-making insights into AI. These contributions helped shape the principle's application in AI strategy and logic.