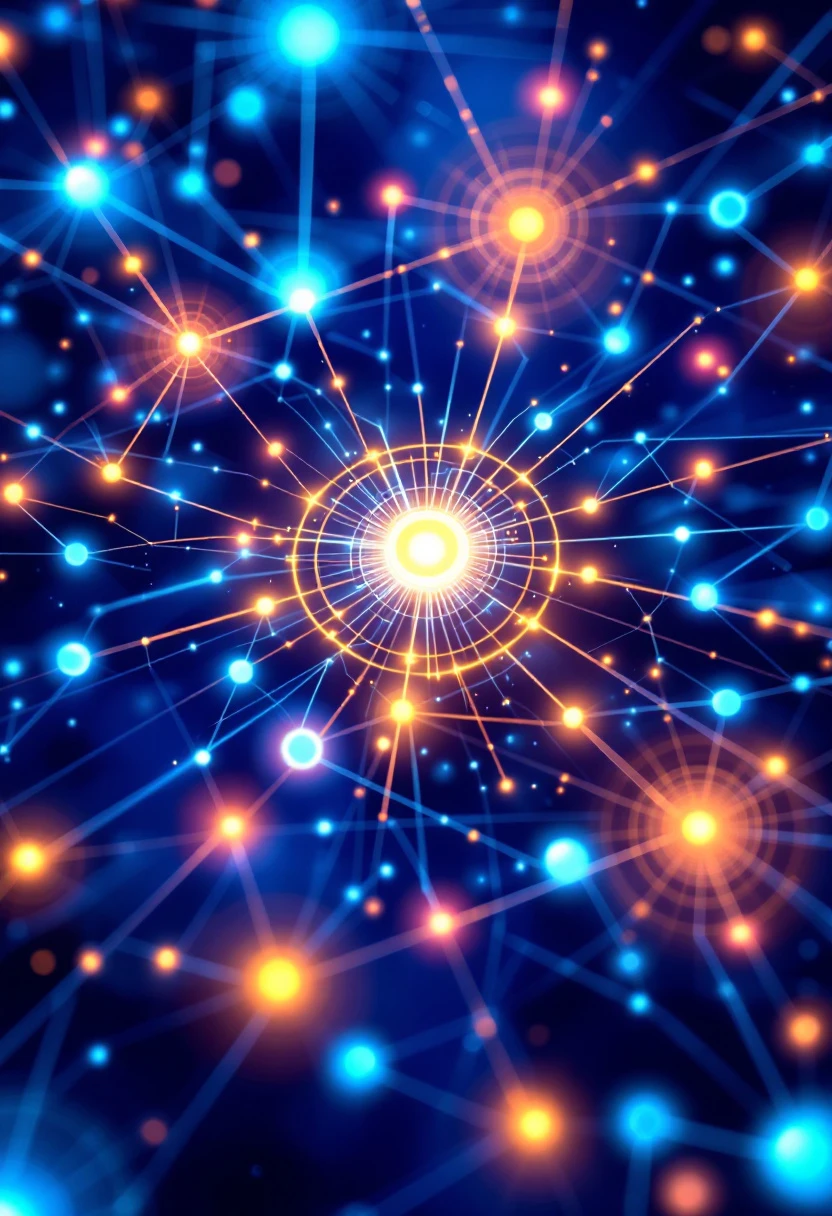
Commonsense Reasoning
The ability of AI systems to make presumptions about the type of
Commonsense reasoning in AI refers to the challenge of enabling machines to simulate human-like understanding by using implicit knowledge about the world, which is not readily available from explicit data. This concept is significant as it addresses a crucial gap in AI's current capabilities, allowing systems to reason about everyday life scenarios, interpret nuanced human language, and make intuitive leaps similar to human cognition. Applications range from improving human-computer interaction, developing autonomous agents for complex environments, to supporting advanced decision-making systems that require an understanding of real-world contexts. Achieving effective commonsense reasoning is challenging because it involves integrating diverse forms of knowledge like physical reasoning, social norms, and cultural practices, requiring advancements in knowledge representation, natural language processing, and cognitive architectures.
The term "commonsense reasoning" gained recognition in the AI field in the 1980s, although its roots can be traced back to earlier AI research pursuits aimed at mimicking human problem-solving abilities. It became particularly prominent in research during the 1990s with endeavors like Doug Lenat’s Cyc project, which aimed to encode vast quantities of everyday human knowledge to improve AI understanding.
Key contributors to the development of commonsense reasoning include Marvin Minsky, who emphasized the importance of using human-like reasoning in AI, and Doug Lenat, whose Cyc project represents one of the early comprehensive attempts to equip machines with a broad base of commonsense knowledge. Their efforts have paved the way for continued research and innovation in bridging human cognitive abilities with AI capabilities.