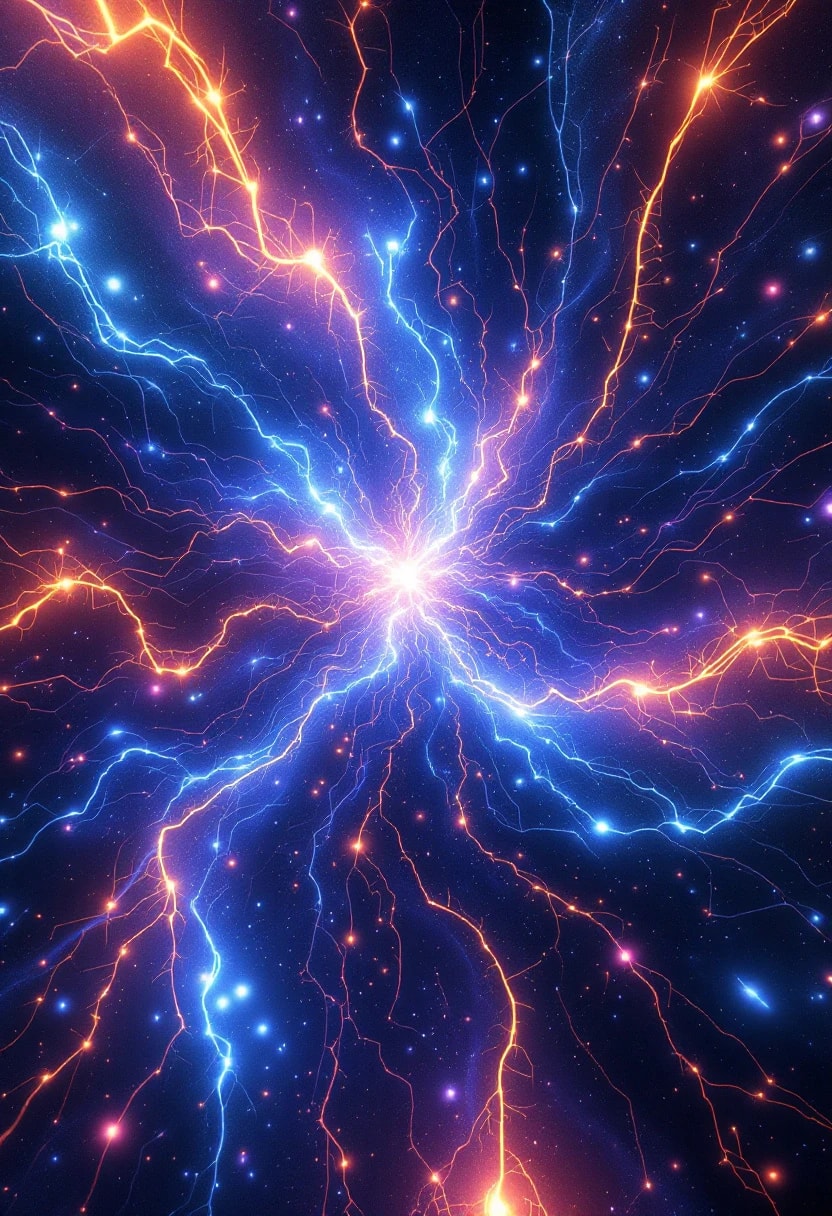
SNARC
Stochastic Neural Analog Reinforcement Calculator
Stochastic Neural Analog Reinforcement Calculator
Recognized as one of the earliest electronic neural network machines, SNARC simulated a rat navigating a maze using analog components and probabilistic logic.
SNARC holds significant historical importance in the evolution of AI, as it was the first computational model to employ principles resembling those found in modern neural networks. Built in 1951, it employed analog circuitry to simulate learning behavior, specifically designed to mimic a rat's trial-and-error process when navigating a maze. The SNARC was foundational in demonstrating the potential for machines to exhibit adaptive behavior through reinforcement learning using stochastic processes. It introduced concepts that would later underpin the development of more sophisticated neural network models, serving as a practical testbed for ideas that were theoretical at the time. Despite its rudimentary nature compared to contemporary neural networks, SNARC illustrated the applicability of biological learning processes to computational systems and set the stage for future developments in connected, multi-node systems that operate on probabilistic feedback to optimize their actions.
The development of SNARC occurred in 1951, marking a pivotal moment in AI history as it predated the popularization of AI and neural networks by several decades. Though it did not gain widespread attention or application outside of research circles immediately, the foundational work laid by SNARC influenced subsequent thinkers and practitioners within AI and ML, particularly those interested in neural computation and learning algorithms.
Claude Shannon and Marvin Minsky were key contributors to the creation of SNARC. Shannon, renowned for his work in information theory, provided the theoretical groundwork, while Minsky, who would later become an influential figure in AI research, spearheaded the project as part of his doctoral thesis work at Harvard University. Their collaborative efforts on SNARC were instrumental in pioneering approaches to artificial learning and computation that anticipated future developments in neural networks and AI systems.