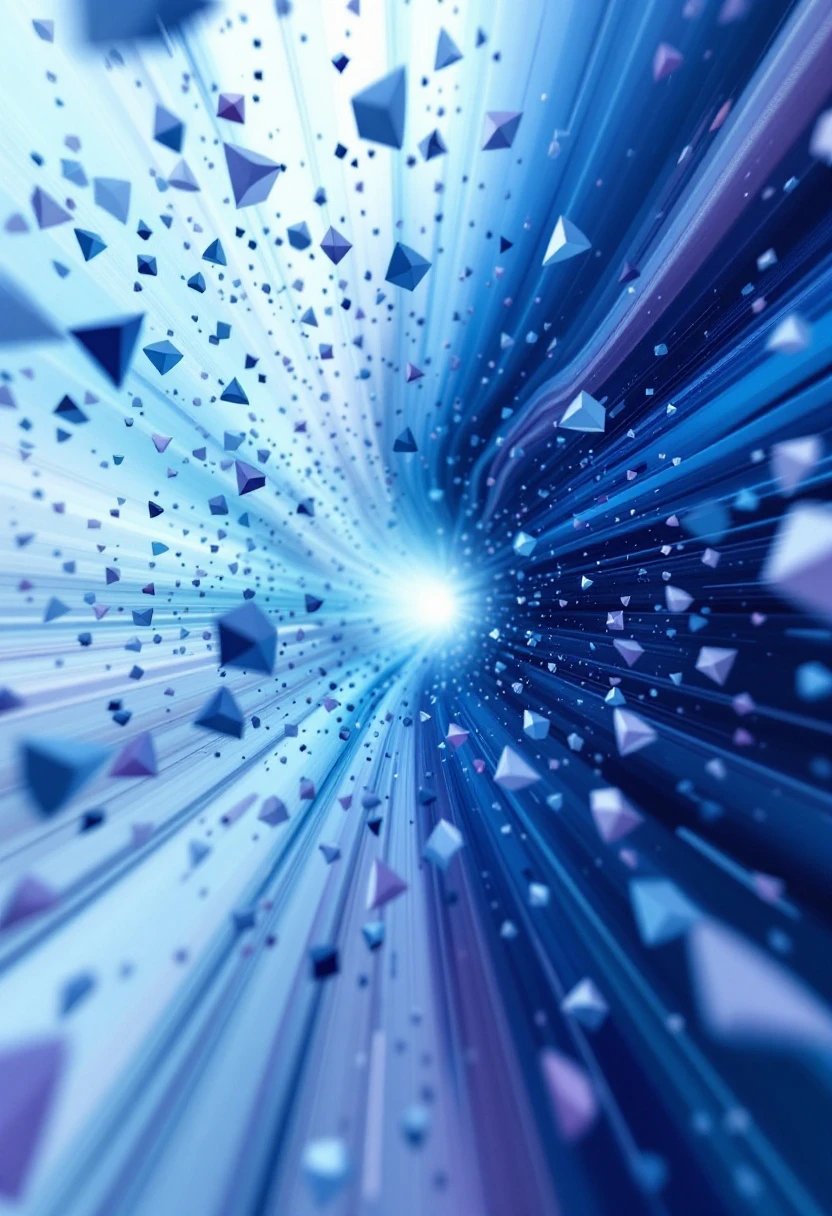
Empirical Risk Minimization
A foundational principle in statistics and ML (Machine Learning), focused on minimizing the average of the loss function over a sample dataset.
Empirical Risk Minimization is a critical concept in the theory of statistical learning and ML, highlighting the process of optimizing model parameters by minimizing the empirical risk or the average loss on the training dataset. This approach serves as a basis for many learning algorithms where the true distribution is unknown, and thus relies on the available sample to derive the best approximation by using an empirical loss function. The practical significance of empirical risk minimization is evident through its applications in diverse scenarios such as classification, regression, and other predictive ML tasks. By applying this principle, one aims to select a model that performs well on observed data, which theoretically leads to consistent estimators when the sample size approaches infinity, under certain regularity conditions.
The principle of empirical risk minimization was first iterated in the mid to late 20th century in the context of statistical learning theory, gaining significant traction and popularity through the work of Vladimir Vapnik and others in the 1970s and beyond, as computational methods and theoretical insights converged to refine learning techniques.
Vladimir Vapnik has been pivotal in popularizing and formalizing the concept of empirical risk minimization, particularly through his contributions to statistical learning theory and development of the Support Vector Machine, underscoring the balance between theoretical foundations and practical applications in AI advancements.