Vladimir Vapnik
(20 articles)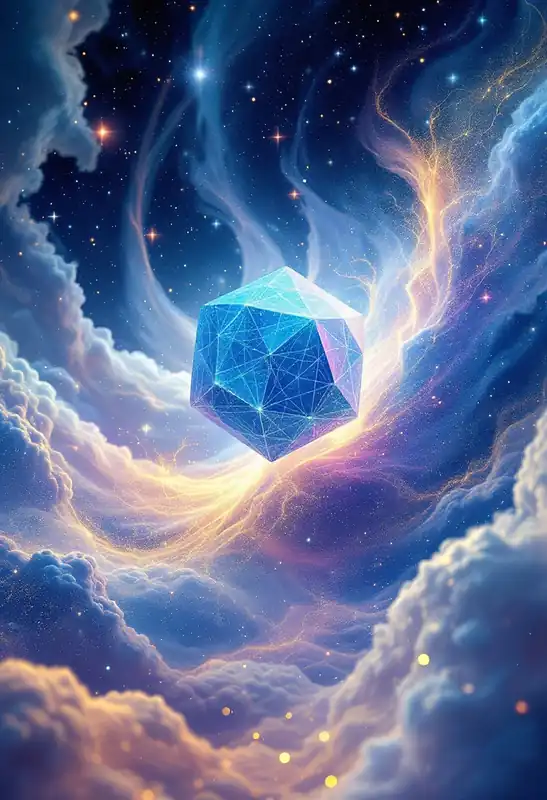
Generalization
Ability of a ML model to perform well on new, unseen data that was not included in the training set.
Generality: 891
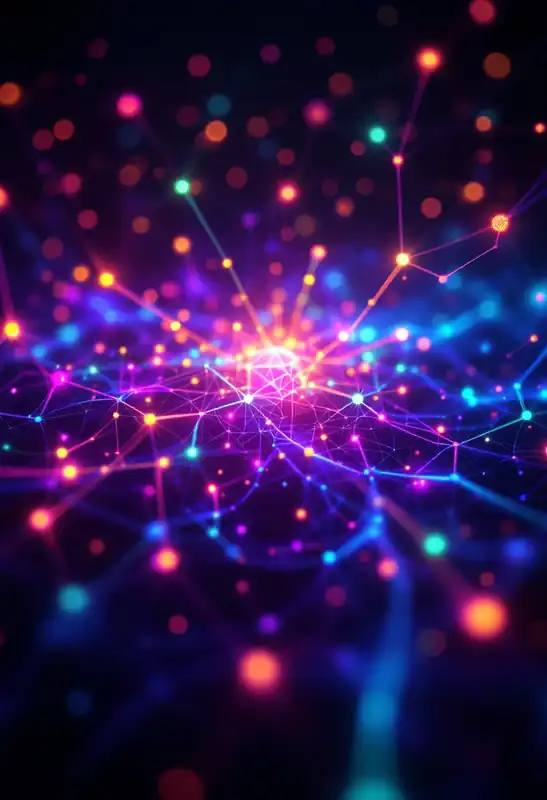
Supervised Classifier
Algorithm that, given a set of labeled training data, learns to predict the labels of new, unseen data.
Generality: 870
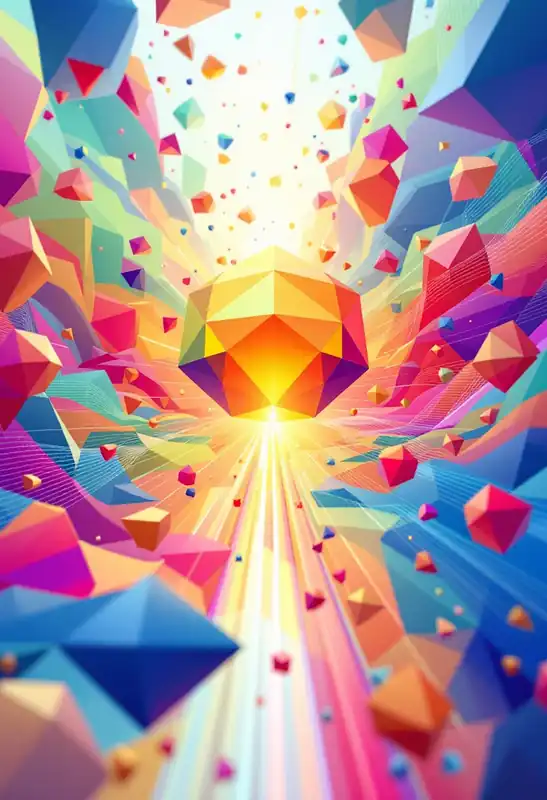
Supervised Learning
ML approach where models are trained on labeled data to predict outcomes or classify data into categories.
Generality: 882
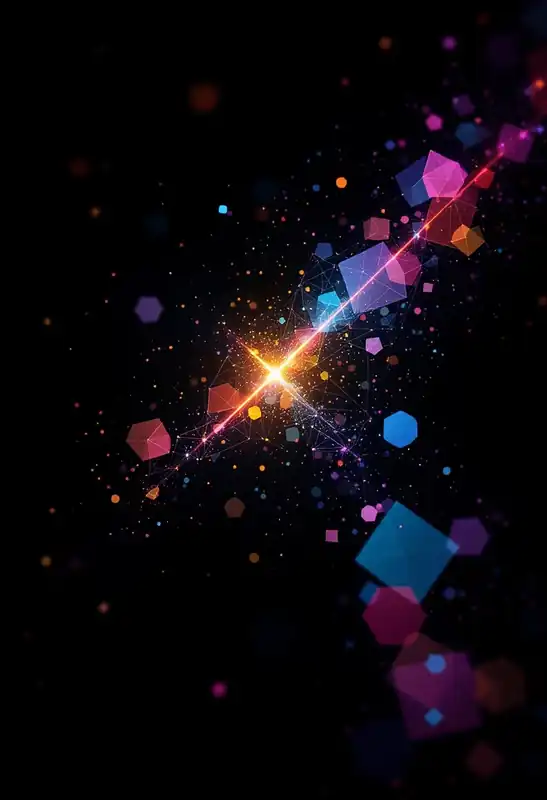
Linear Separability
The ability of a dataset to be perfectly separated into two classes using a straight line in two dimensions or a hyperplane in higher dimensions.
Generality: 500
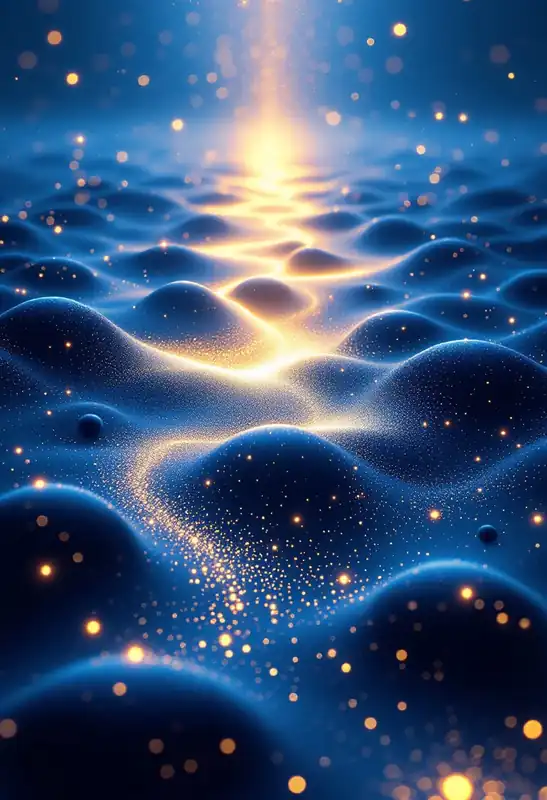
Regularization
Technique used in machine learning to reduce model overfitting by adding a penalty to the loss function based on the complexity of the model.
Generality: 845
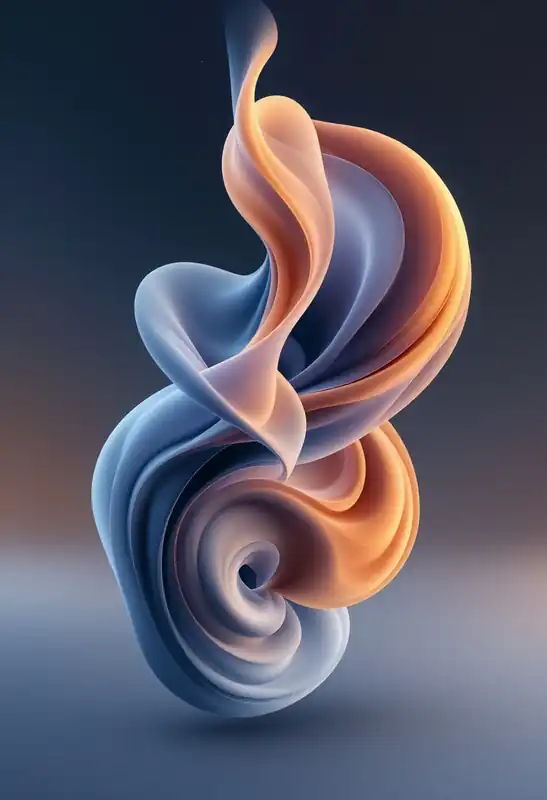
Bias-Variance Trade-off
In ML, achieving optimal model performance involves balancing bias and variance to minimize overall error.
Generality: 818
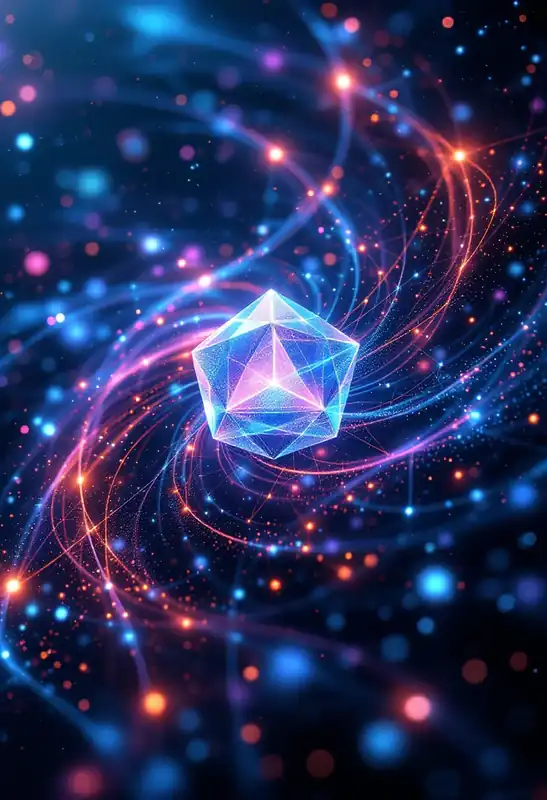
VC Dimension
Vapnik-Chervonenkis
Vapnik-Chervonenkis
Measure of the capacity of a statistical classification algorithm, quantifying how complex the model is in terms of its ability to fit varied sets of data.
Generality: 806
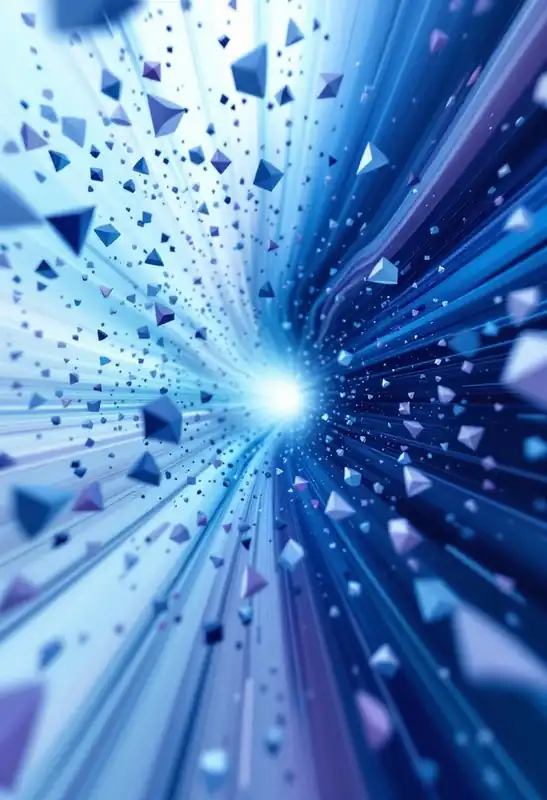
Empirical Risk Minimization
A foundational principle in statistics and ML (Machine Learning), focused on minimizing the average of the loss function over a sample dataset.
Generality: 814
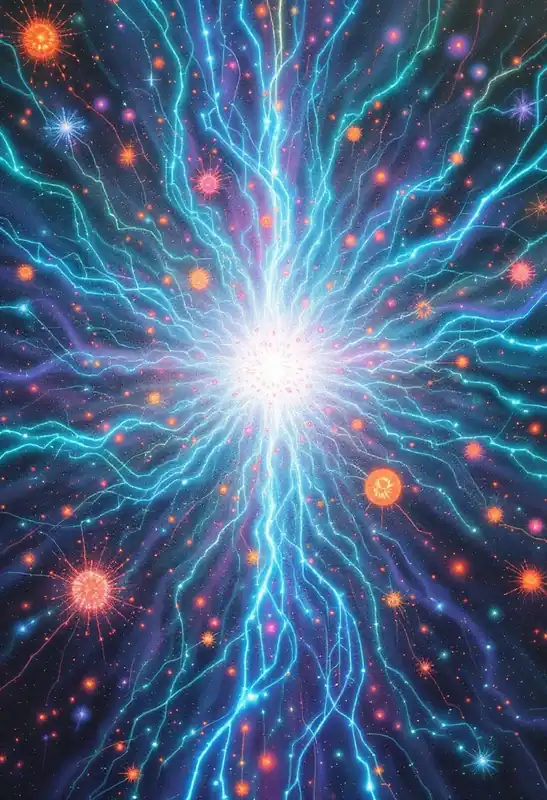
Overfitting
When a ML model learns the detail and noise in the training data to the extent that it negatively impacts the performance of the model on new data.
Generality: 890
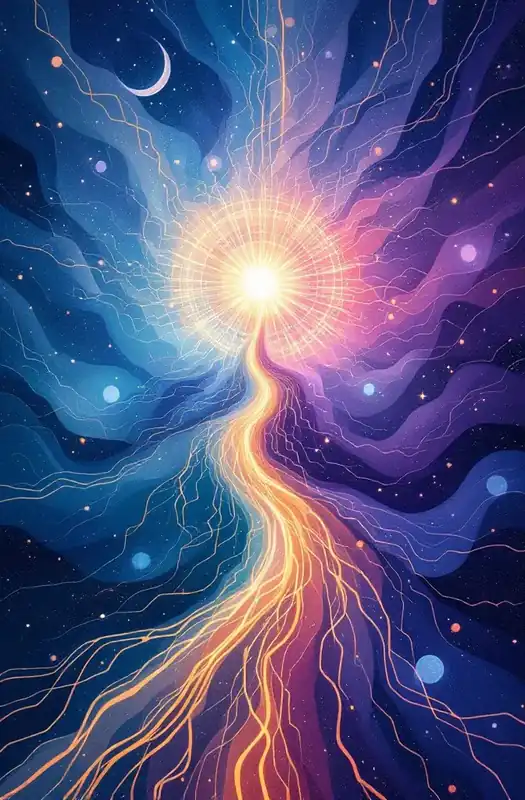
Inductive Bias
Assumptions integrated into a learning algorithm to enable it to generalize from specific instances to broader patterns or concepts.
Generality: 827
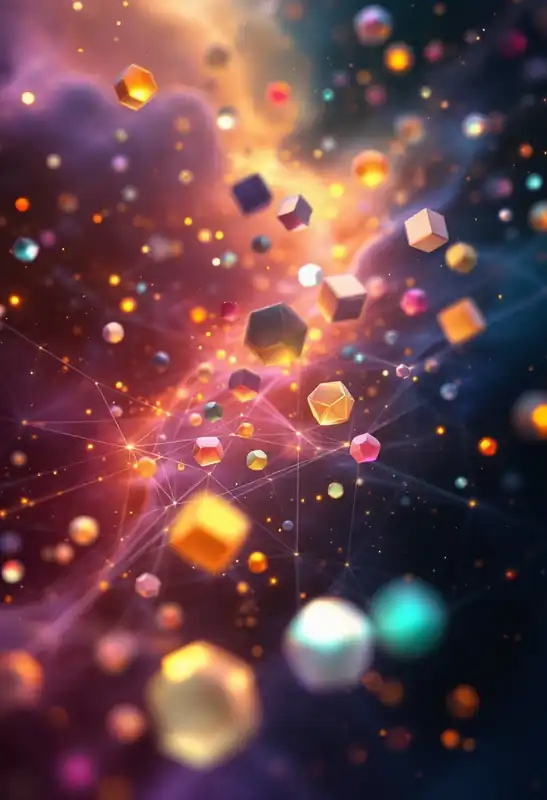
Boosting
ML ensemble technique that combines multiple weak learners to form a strong learner, aiming to improve the accuracy of predictions.
Generality: 800
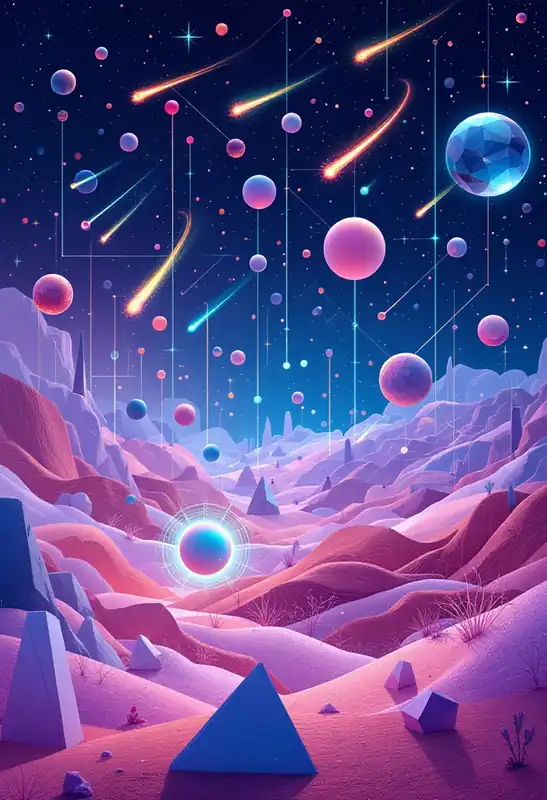
Ensamble Algorithm
Combines multiple machine learning models to improve overall performance by reducing bias, variance, or noise.
Generality: 860
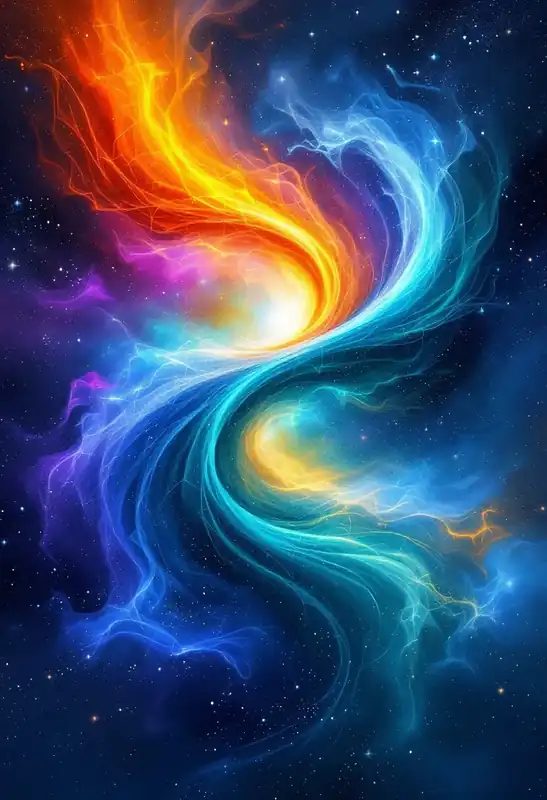
Bias-Variance Dilemma
Fundamental problem in supervised ML that involves a trade-off between a model’s ability to minimize error due to bias and error due to variance.
Generality: 893
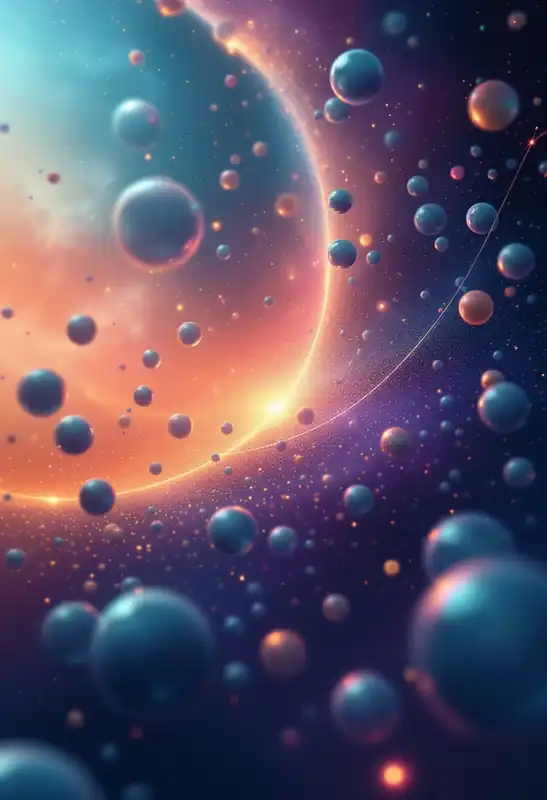
Margin
In the context of AI, particularly in Support Vector Machines (SVM), margin refers to the separation between data points of different classes, signifying the distance between the decision boundary and the closest data points of the classes.
Generality: 500
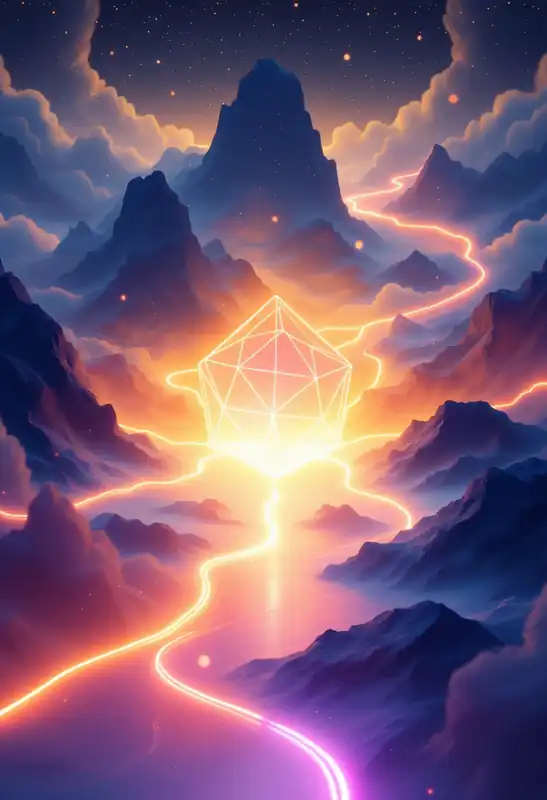
Ensemble Methods
ML technique where multiple models are trained and used collectively to solve a problem.
Generality: 860
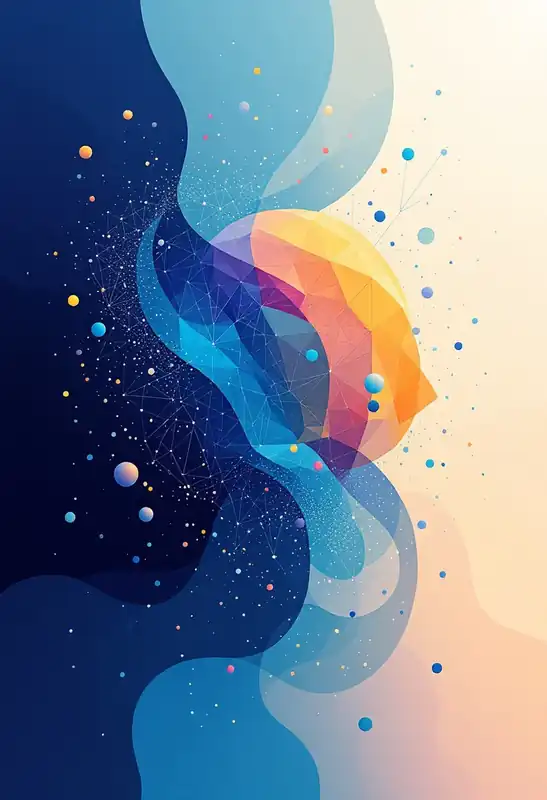
Ensemble Learning
ML paradigm where multiple models (often called weak learners) are trained to solve the same problem and combined to improve the accuracy of predictions.
Generality: 795
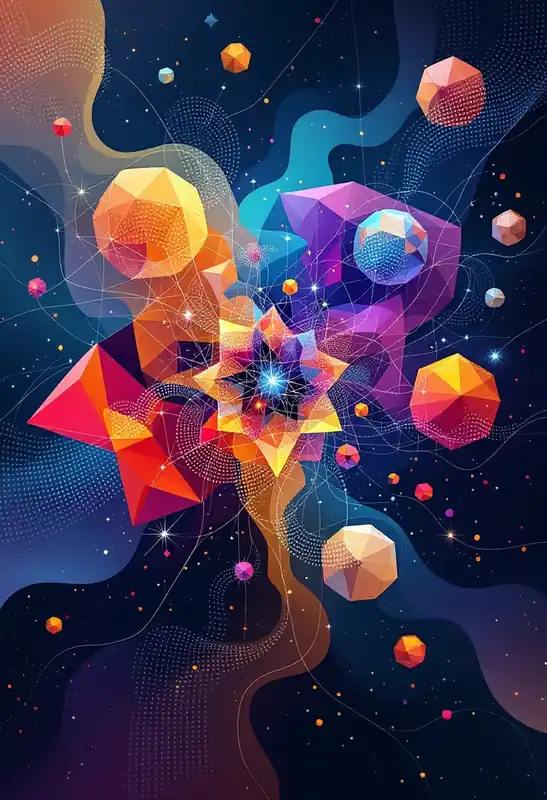
Meta-Classifier
Algorithm that combines multiple ML models to improve prediction accuracy over individual models.
Generality: 811
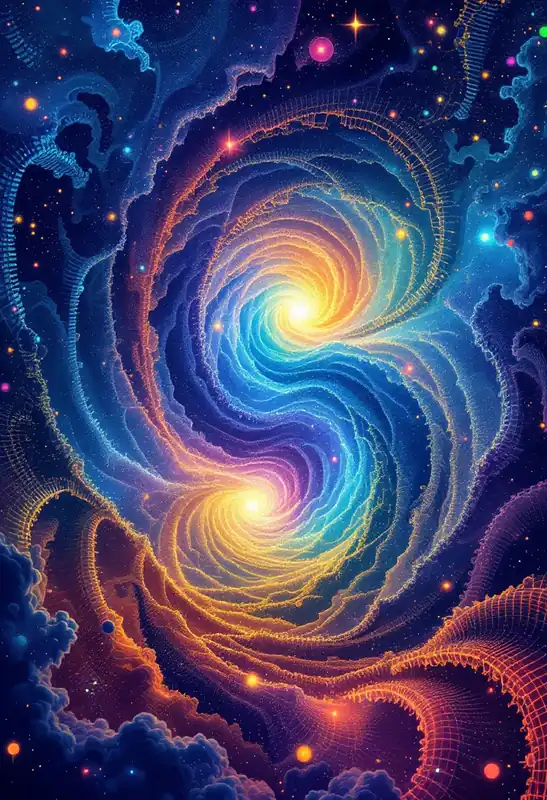
Kernel Method
A set of algorithms that enable machine learning models to perform in high-dimensional spaces without directly computing those dimensions.
Generality: 500
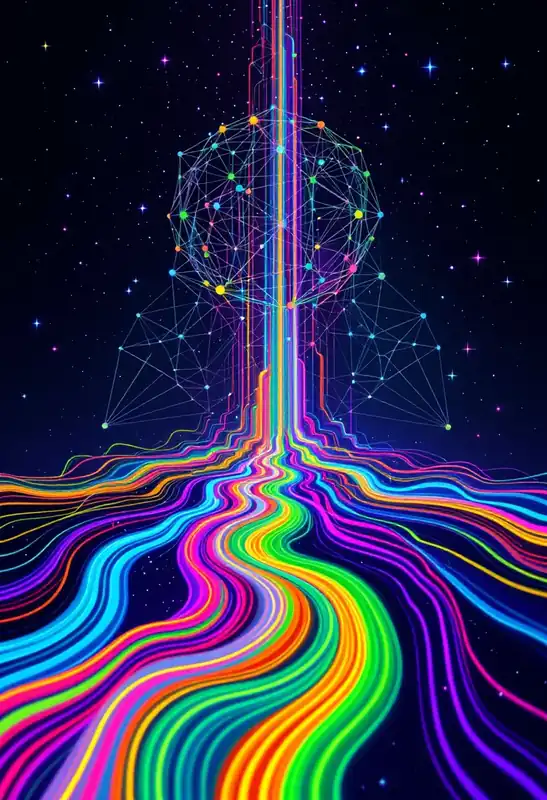
Classifier
ML model that categorizes data into predefined classes.
Generality: 861
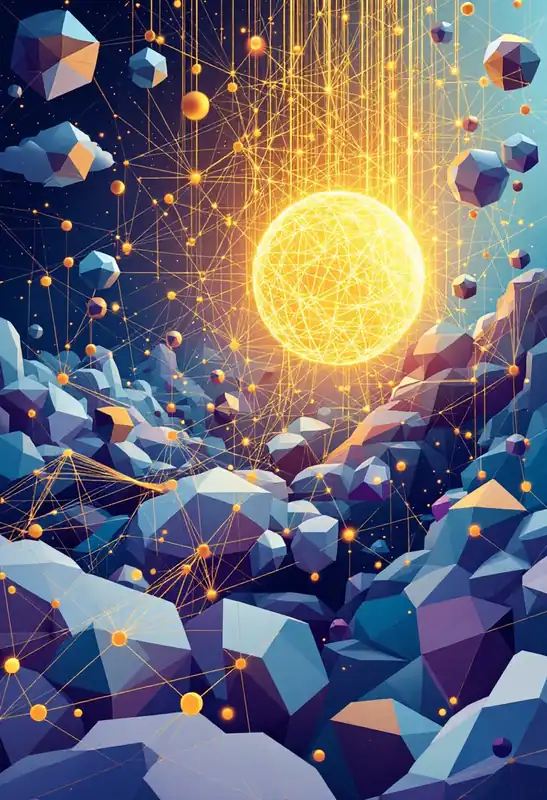
Discriminative AI
Algorithms that learn the boundary between classes of data, focusing on distinguishing between different outputs given an input.
Generality: 840