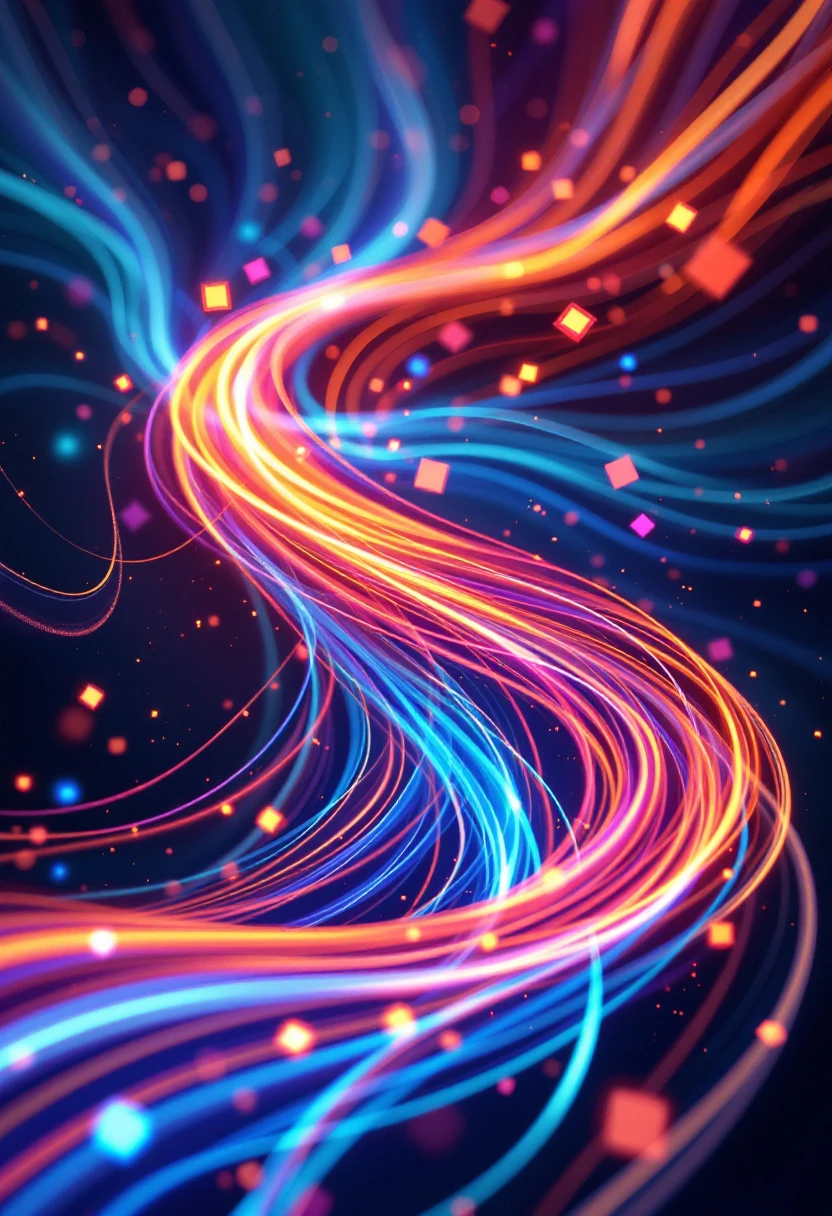
Temporal Data
Data that is associated with time, capturing the sequence and timing of events or states.
Temporal data plays a crucial role in AI by providing context for analyzing patterns and trends over time, enabling models to understand and predict time-dependent phenomena such as stock market trends, user behavior changes, or natural event sequences. This type of data is integral in applications like time-series forecasting, where algorithms need to capture temporal dynamics to make accurate predictions, and in dynamic systems modeling, where the evolution of state over time is pivotal. Handling temporal data necessitates specialized techniques that account for temporality's unique characteristics, such as autocorrelation and seasonality, making time a critical dimension in data analysis.
The use of temporal data dates back to the early stages of computerized data analysis, gaining significant traction in the late 20th century with the rise of time-series analysis methods, though it became particularly prominent with the advent of big data and the increasing demand for predictive analytics in industries post-2010.
Key contributors to the development of concepts related to temporal data include researchers in time-series analysis, such as George Box and Gwilym Jenkins, who laid the groundwork with the Box-Jenkins methodology in the 1970s, and Jeffrey Heer, who has influenced visual and computational methods for analyzing complex datasets with temporal components.