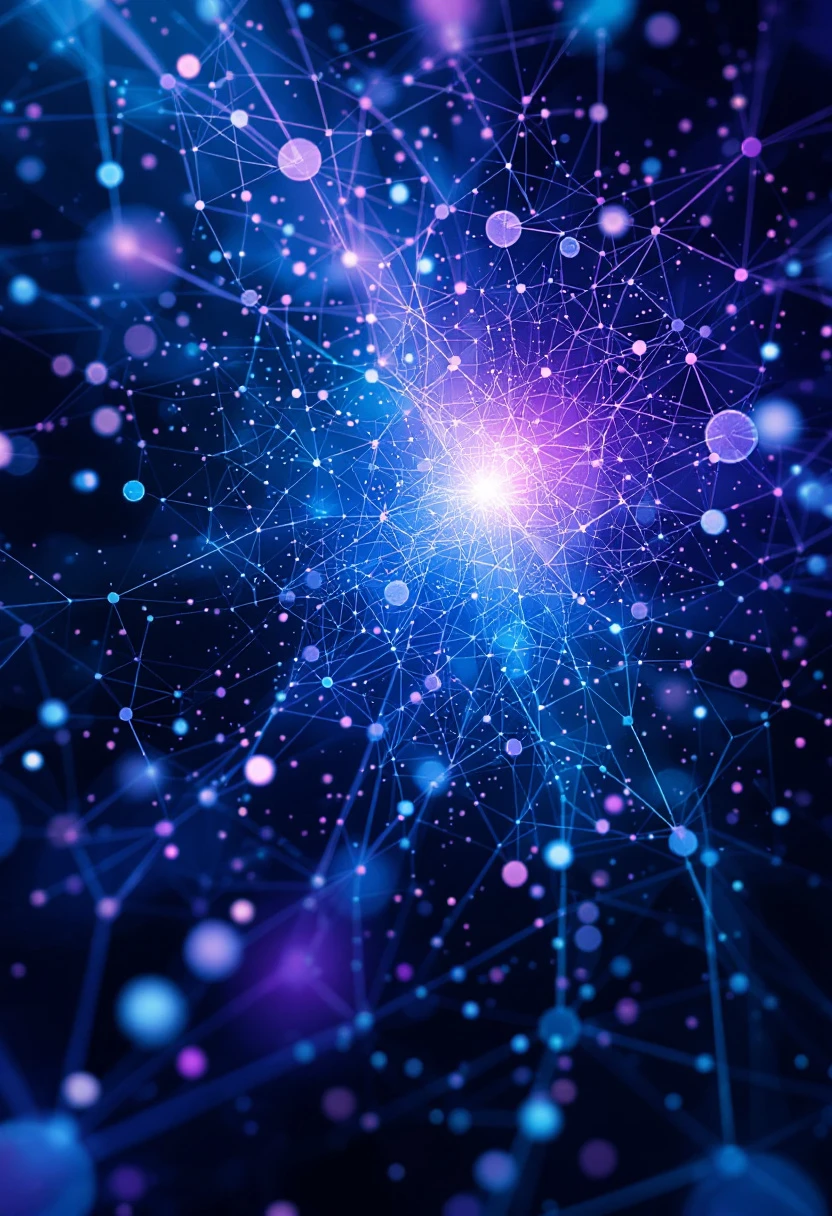
SRL
Statistical Relational Learning
Statistical Relational Learning
Combines statistics and relational data to construct models that can learn from complex, structured data involving multiple interdependent entities.
SRL (Statistical Relational Learning) addresses the challenge of learning in environments where data is not only represented as individual instances but also involves rich relational structure amongst many entities. It achieves this by integrating statistical methods with logical or relational learning, enabling more expressive models that can capture dependencies and interactions in structured data, like those found in social networks, biological data, or multi-relational databases. SRL models can generalize across different contexts by understanding both the properties of entities and the relations between them, making it crucial for applications requiring nuanced predictions based on interconnected data, such as fraud detection or biological network modeling.
The term and concept of SRL (Statistical Relational Learning) began emerging around the late 1990s and gained traction in the 2000s as computational resources and interest in leveraging relational data in AI increased, particularly due to the limitations perceived in traditional flat-file approaches to data handling.
Key contributors to SRL include researchers like Lise Getoor and Nir Friedman, who have significantly advanced the field through foundational research and development of frameworks that bridge statistical methods with relational data systems. Their work, along with others in the field, has helped establish SRL as a critical area in AI for effectively managing and analyzing complex datasets.