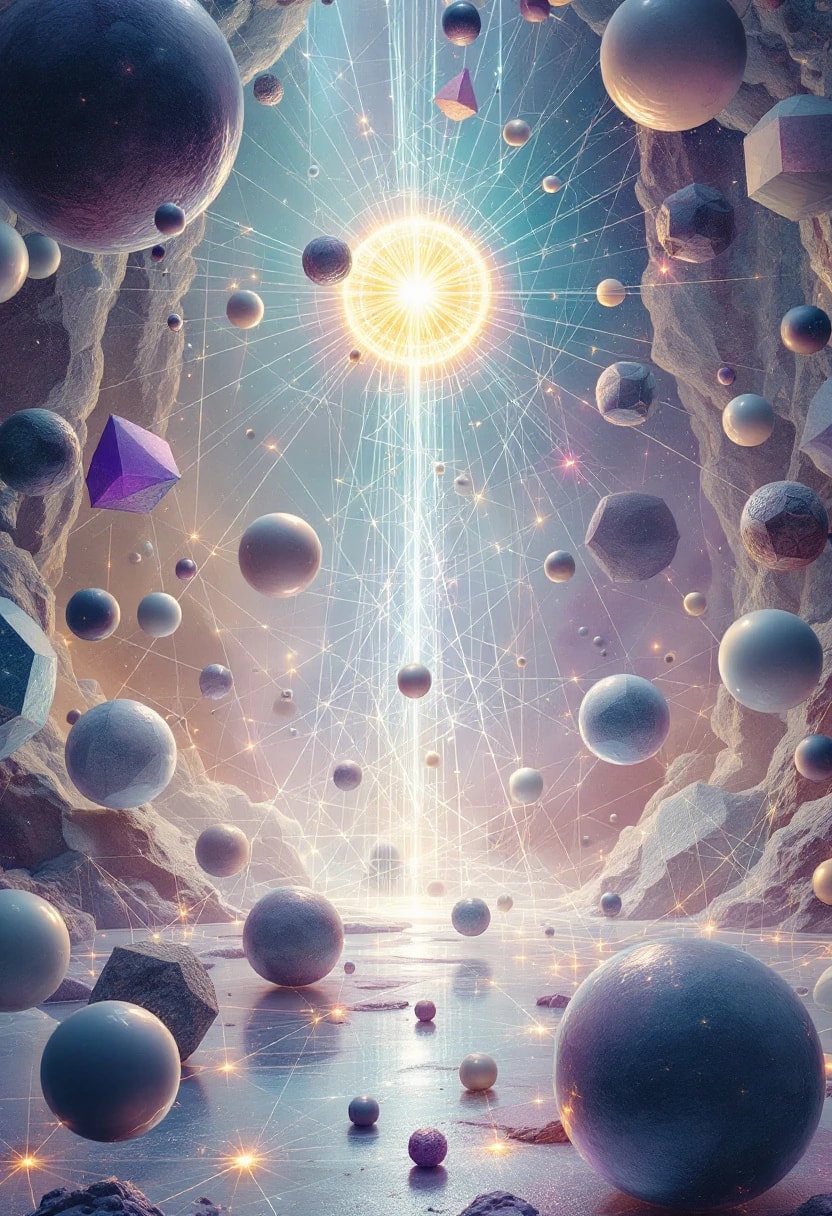
Spatial Intelligence
Ability of a system to understand, reason, and manipulate spatial relationships and properties within its environment.
Spatial intelligence in AI involves the development of algorithms and models that can interpret and process spatial data, allowing machines to understand and navigate three-dimensional spaces. This capability is crucial for applications such as robotics, autonomous vehicles, and geographic information systems (GIS). Spatial intelligence encompasses tasks like object recognition, scene reconstruction, and spatial reasoning, enabling AI systems to interact with the physical world more effectively. Techniques such as convolutional neural networks (CNNs), point cloud processing, and spatial-temporal modeling are often employed to enhance an AI system's spatial understanding and decision-making capabilities.
The concept of spatial intelligence has been integral to AI since the advent of robotics and computer vision in the 1960s and 1970s. However, it gained significant traction in the early 2000s with advances in machine learning and the increased availability of spatial data from sources like LiDAR, satellite imagery, and GPS.
Key figures in the development of spatial intelligence include pioneers in computer vision and robotics such as David Marr, who contributed to the understanding of visual processing, and researchers like Sebastian Thrun, who advanced autonomous vehicle technology. Institutions such as MIT and Stanford University have also been instrumental in pushing the boundaries of spatial intelligence research.