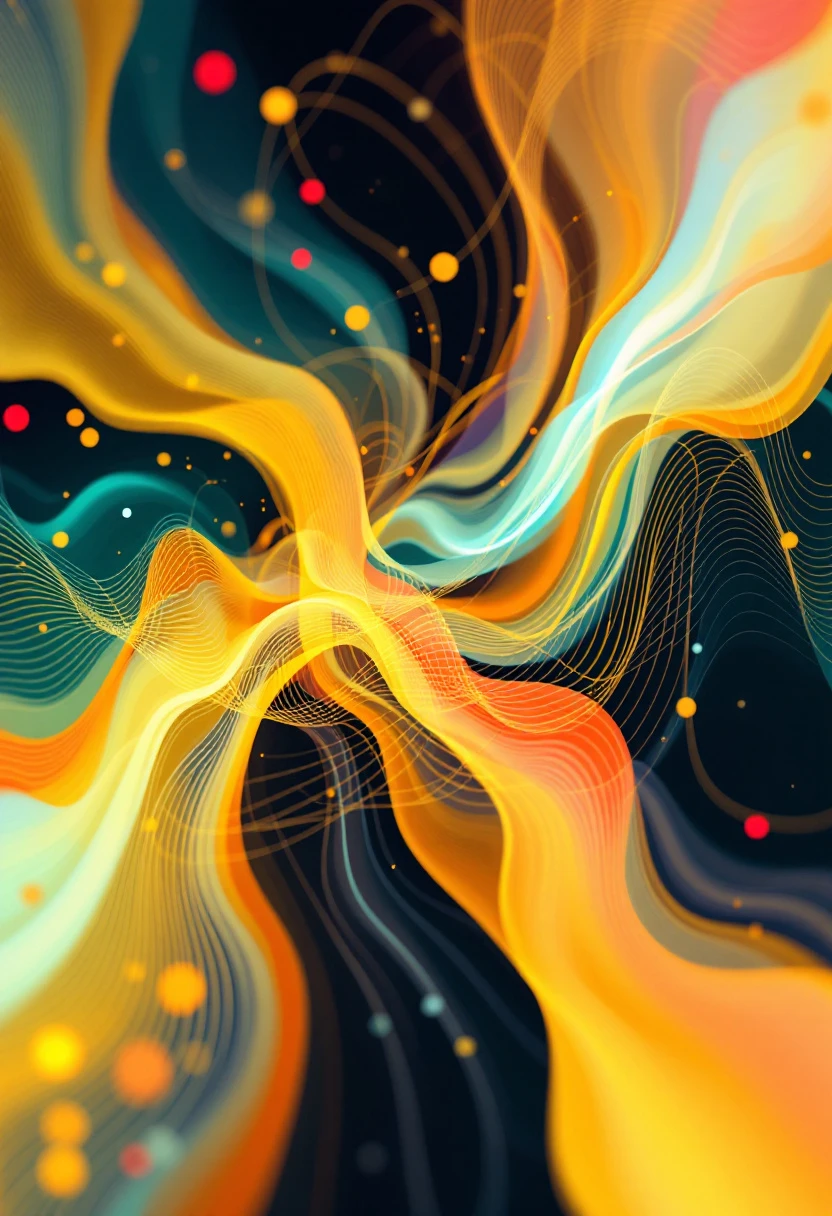
Numerical Data
Data that is represented in the form of numbers, allowing for quantitative analysis and computational applications in AI and ML.
Numerical data plays a crucial role in AI, offering a foundation for quantitative analysis and various computational methods. Extensively used in the training of AI and ML models, numerical data allows for the development of algorithms capable of identifying patterns, making predictions, and performing other analytics tasks by quantifying attributes. In AI, numerical data is often utilized for statistical modeling and optimization, providing insights through methods like linear regression, clustering, and neural networks. Its significance is upheld in areas like computer vision, natural language processing, and reinforcement learning, where numerical representation of information enhances computational efficiency and accuracy.
The use of numerical data in computational contexts dates back to the mid-20th century, paralleling advancements in digital computing. However, its prominence in AI and ML gained significant traction during the late 1990s and early 2000s with the explosive growth of data and increased computational power, marking a surge in data-driven methodologies.
Key contributors to the development and utilization of numerical data in AI include mathematicians and computer scientists who advanced data analysis and statistical methods, notably John Tukey for exploratory data analysis, and Ronald Fisher for establishing fundamental statistical techniques. The expansion of digital computation by figures like Alan Turing also laid the groundwork for the computational handling of numerical data.