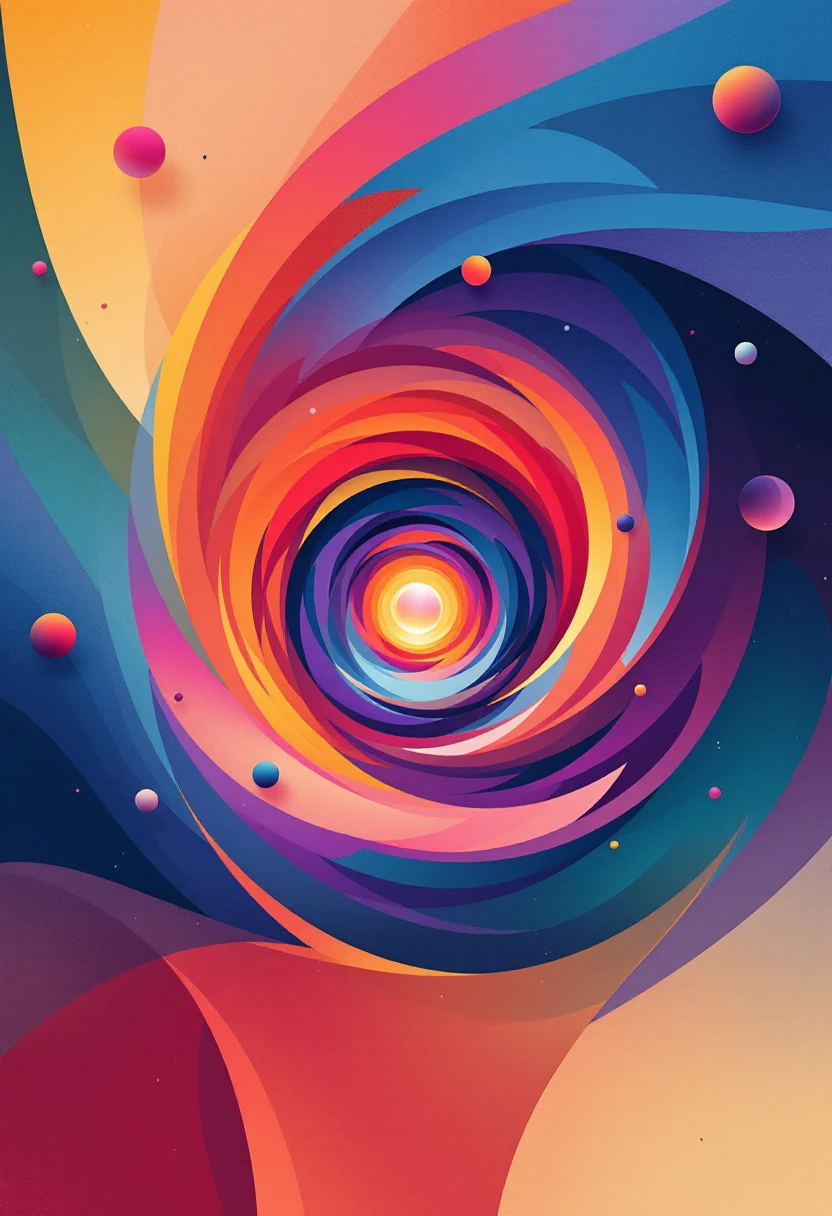
Interestingness
Measure of how engaging or surprising information is, often used in ML and computational creativity to prioritize novel and useful data.
In AI and machine learning, interestingness is a concept used to evaluate the novelty, relevance, or unexpectedness of data or outcomes. It plays a crucial role in areas like data mining, recommendation systems, and generative models, where the goal is to surface information that is not just statistically significant but also engaging or insightful to users. Algorithms often balance between predictable information and outliers that may offer new insights. Interestingness metrics can vary based on the application, incorporating factors like user preferences, novelty, relevance, and diversity. In computational creativity, for instance, interestingness helps AI systems generate creative outputs by encouraging unexpected combinations or patterns while maintaining coherence and value.
The concept of interestingness in AI dates back to the 1980s, when it first appeared in discussions related to knowledge discovery and data mining. However, it gained broader popularity with the advent of recommendation systems and advances in machine learning in the early 2000s, where the need to filter or prioritize large volumes of data became critical.
Pioneers in data mining like Rakesh Agrawal, and subsequent work by researchers in recommendation systems such as Yehuda Koren and Deepak Agarwal, have contributed to developing metrics and techniques that quantify and leverage interestingness in AI systems.