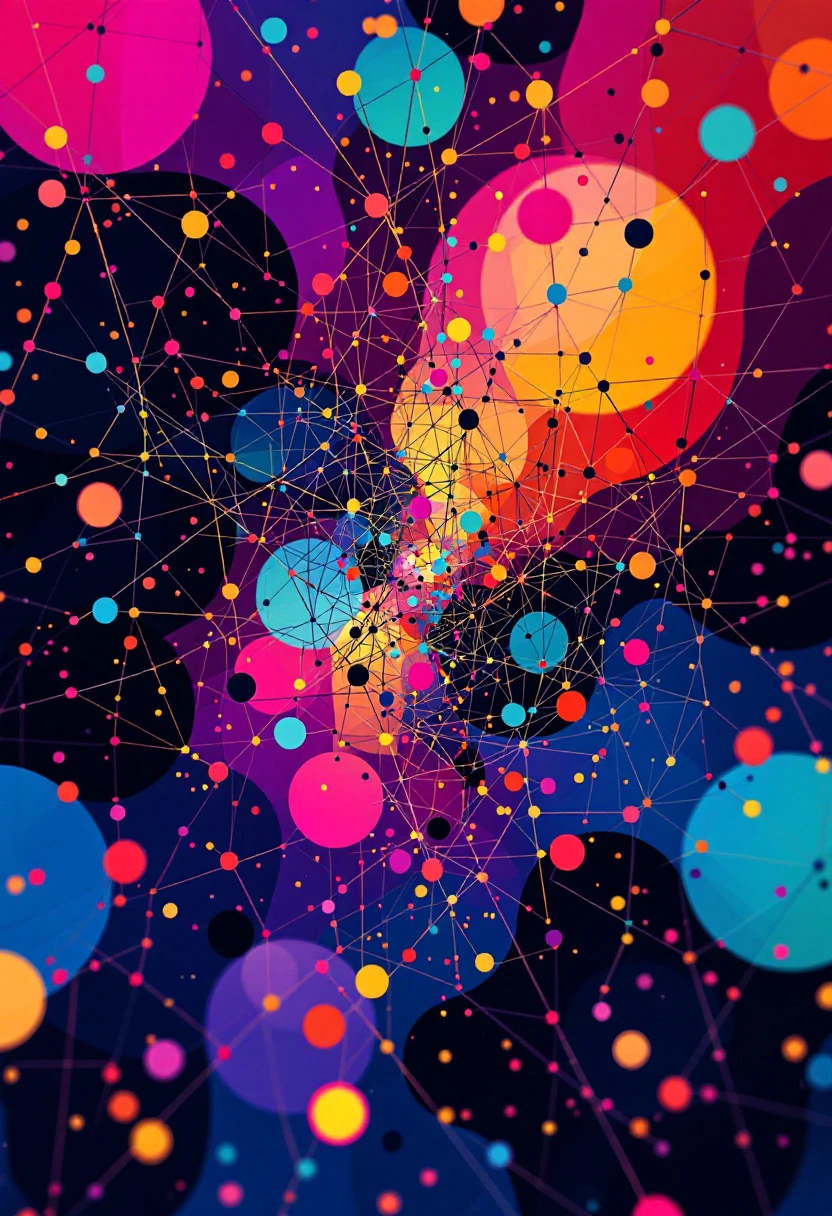
Graph Theory
Field of mathematics and computer science focusing on the properties of graphs, which are structures made up of vertices (or nodes) connected by edges.
Graph theory plays a crucial role in AI for modeling relationships and interactions in complex systems, including networks of information, social networks, biological networks, and more. Its significance in AI stems from its ability to provide a framework for solving problems related to routing, connectivity, and optimization. In machine learning, graphs are used in various algorithms that operate on data represented as graphs, such as graph neural networks (GNNs), to capture the relationships between entities in a way that enhances predictive modeling and classification tasks.
Graph theory originated in 1736 with Leonhard Euler's solution to the Königsberg bridge problem, marking the beginning of the field. Its relevance to computer science and AI has grown significantly with the advent of large-scale data processing and the need for efficient data structuring and analysis methods.
While Leonhard Euler is credited with the founding of graph theory, many other mathematicians and computer scientists have contributed to its development, including Gustav Kirchhoff, who introduced the concept of trees in 1847, and Paul Erdős and Alfréd Rényi, who developed random graph theory in the mid-20th century. In the context of AI, researchers like Yoshua Bengio and Thomas Kipf have been instrumental in applying graph theory to deep learning, particularly in the development of graph neural networks.
Explainer
The Traveling Salesman Problem
Graph Theory Foundation
Cities are nodes (vertices) and possible routes are edges. The distance between any two cities represents the edge weight.
How to Read This Visualization:
Why 100 routes?
With 10 cities, there are 362,880 possible routes (9! different paths). Checking them all would take too long, so this demo samples 100 random routes to demonstrate how AI can find good solutions without exhaustive search.
How is distance measured?
Distances are measured in screen pixels between cities on the map. While not representing real-world distances, this helps us compare different routes - shorter pixel lengths mean more efficient paths.
Graph theory helps us model the TSP as a network where cities are nodes and possible routes are edges. AI algorithms then search this graph for the shortest possible route, demonstrating how theoretical concepts power practical solutions.