John Schulman
(12 articles)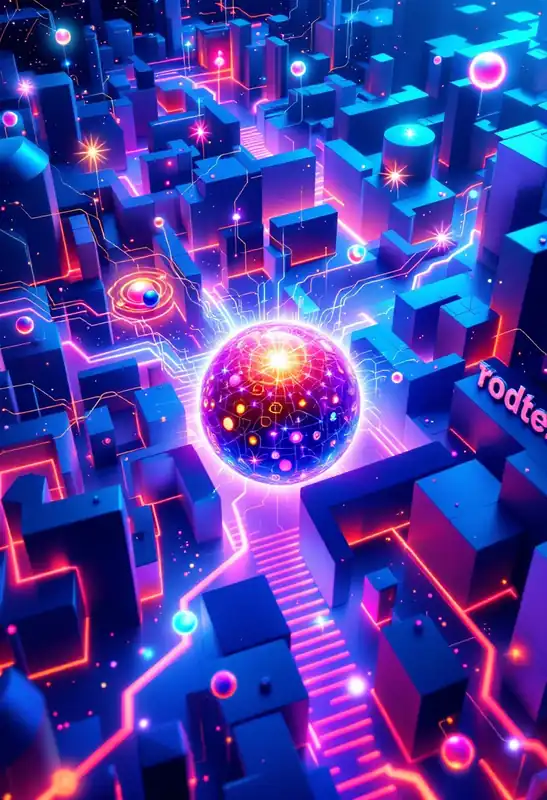
RL
Reinforcement Learning
Reinforcement Learning
Type of ML where an agent learns to make decisions by performing actions in an environment to achieve a goal, guided by rewards.
Generality: 890
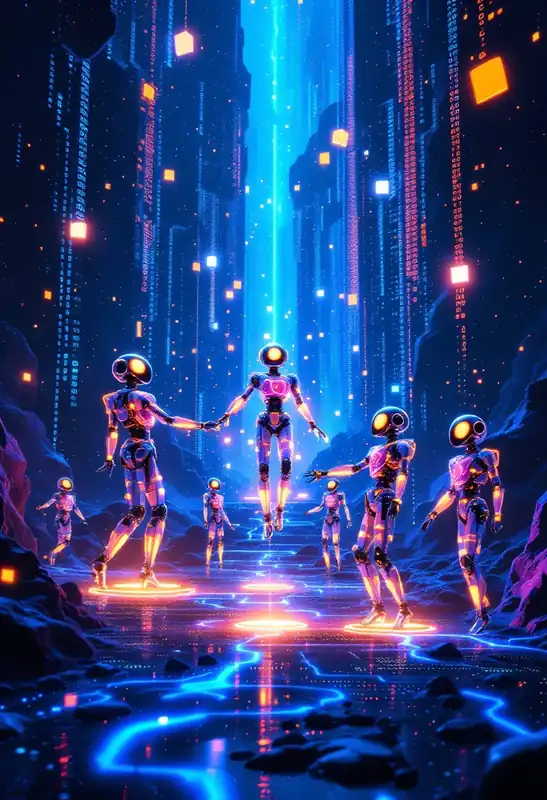
Motor Learning
Process by which robots or AI systems acquire, refine, and optimize motor skills through experience and practice.
Generality: 675
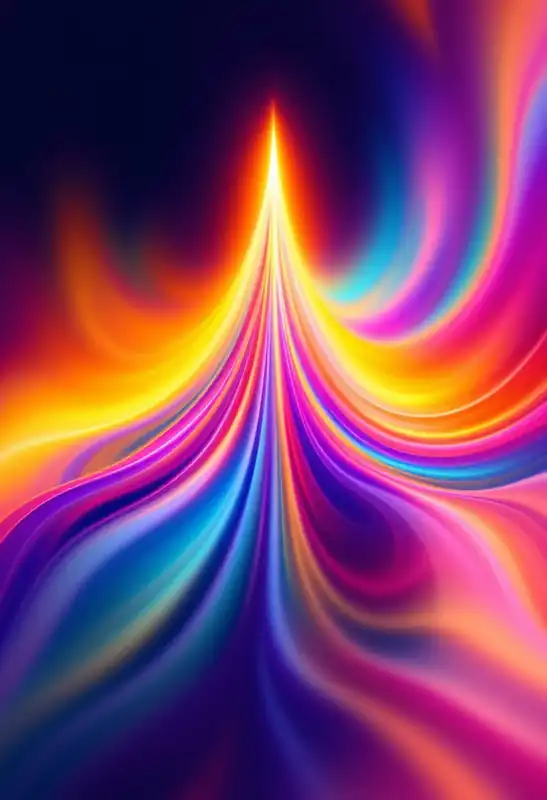
Policy Gradient
Class of algorithms in RL that optimizes the parameters of a policy directly through gradient ascent on expected future rewards.
Generality: 675
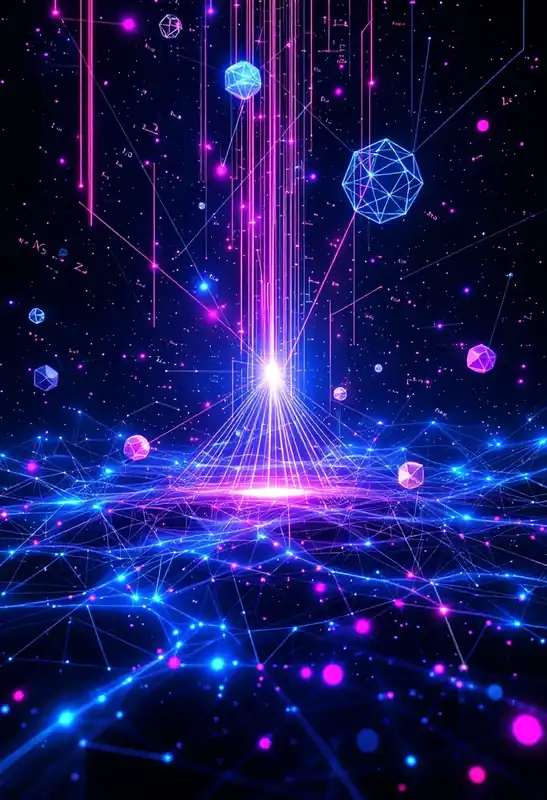
IRL
Inverse Reinforcement Learning
Inverse Reinforcement Learning
Technique in which an algorithm learns the underlying reward function of an environment based on observed behavior from an agent, essentially inferring the goals an agent is trying to achieve.
Generality: 658
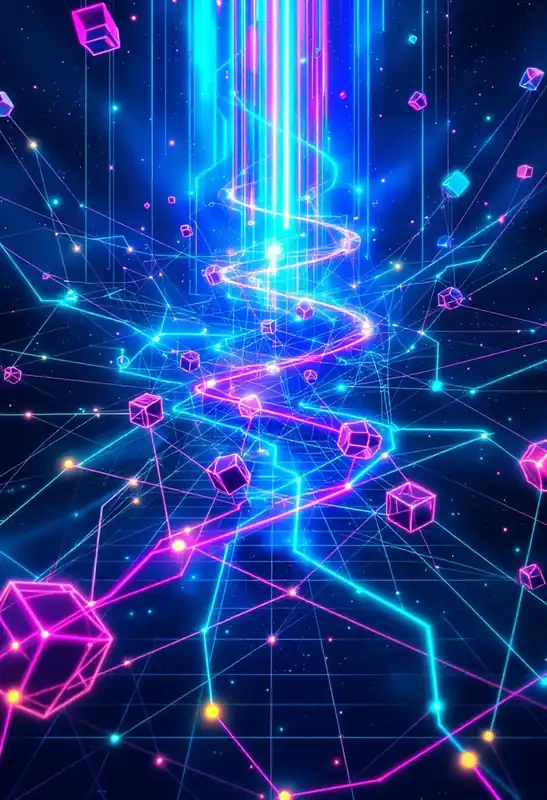
DRL
Deep Reinforcement Learning
Deep Reinforcement Learning
Combines neural networks with a reinforcement learning framework, enabling AI systems to learn optimal actions through trial and error to maximize a cumulative reward.
Generality: 855
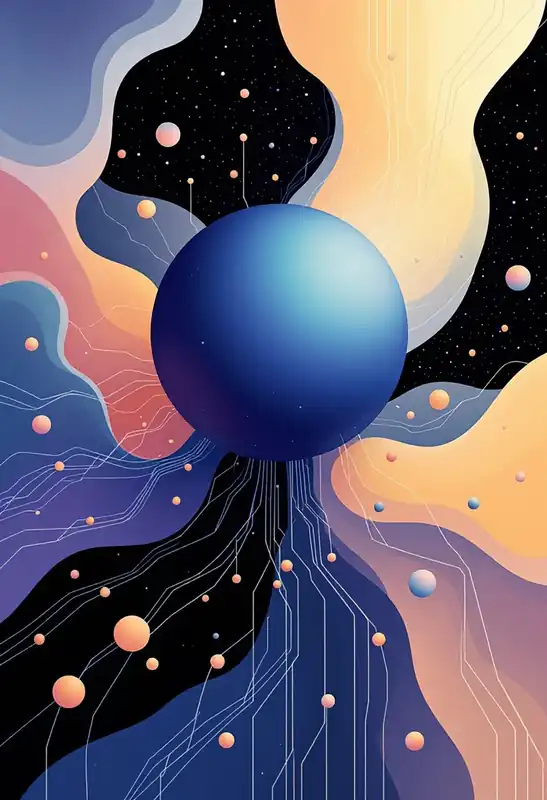
TRPO
Trust Region Policy Optimization
Trust Region Policy Optimization
Advanced algorithm used in RL to ensure stable and reliable policy updates by optimizing within a trust region, thus preventing drastic policy changes.
Generality: 635
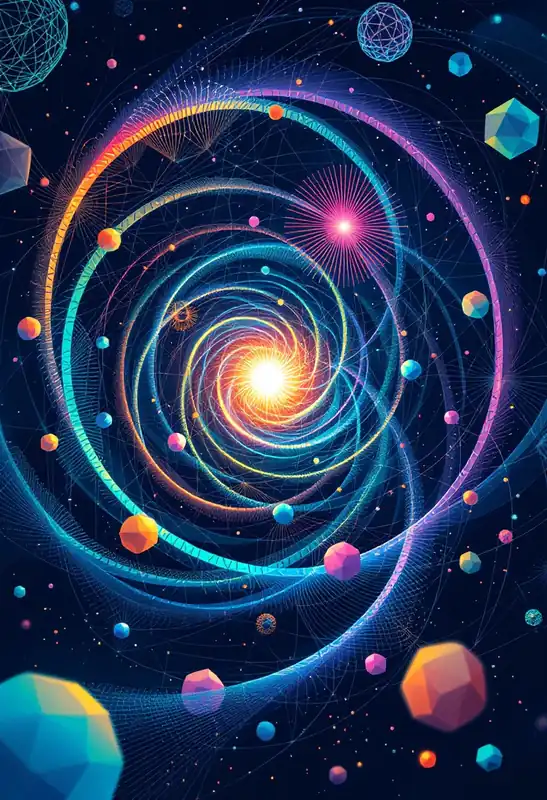
DQN
Deep Q-Networks
Deep Q-Networks
RL technique that combines Q-learning with deep neural networks to enable agents to learn how to make optimal decisions from high-dimensional sensory inputs.
Generality: 853
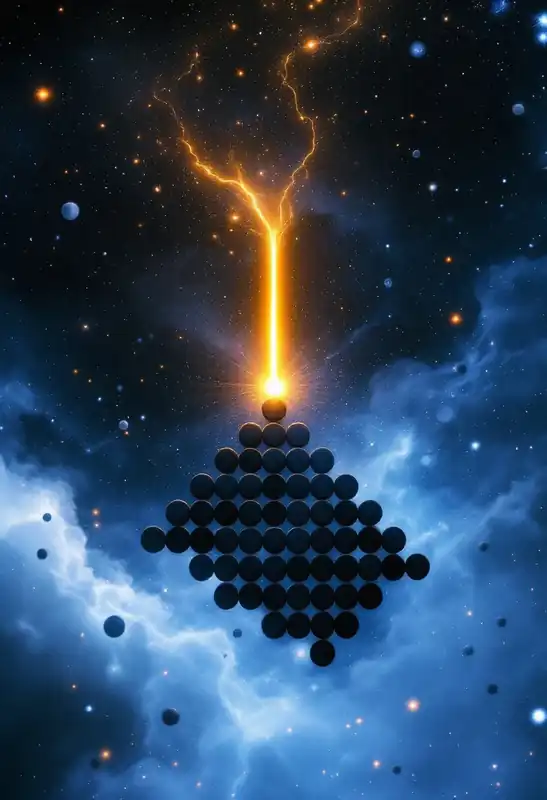
Move 37
Pivotal move made by AlphaGo in its second game against Go champion Lee Sedol, which showcased the superior strategic capabilities of AI in the game of Go.
Generality: 140
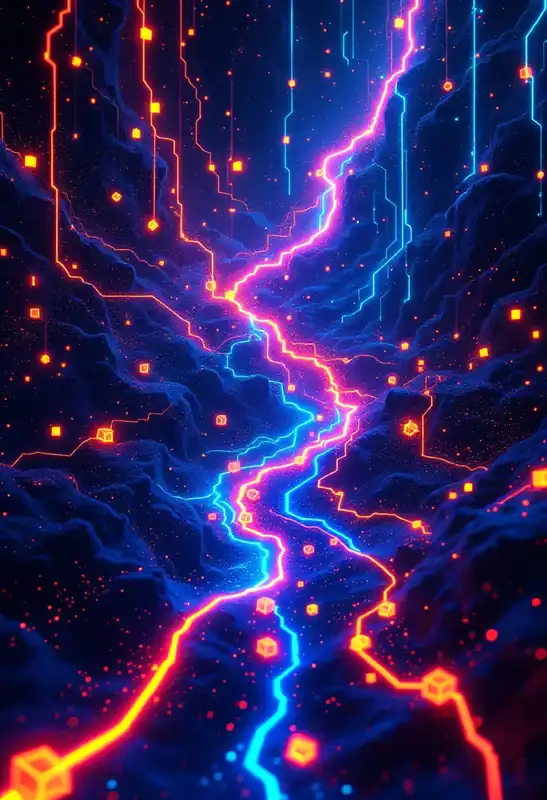
RLHF
Reinforcement Learning from Human Feedback
Reinforcement Learning from Human Feedback
Technique that combines reinforcement learning (RL) with human feedback to guide the learning process towards desired outcomes.
Generality: 625
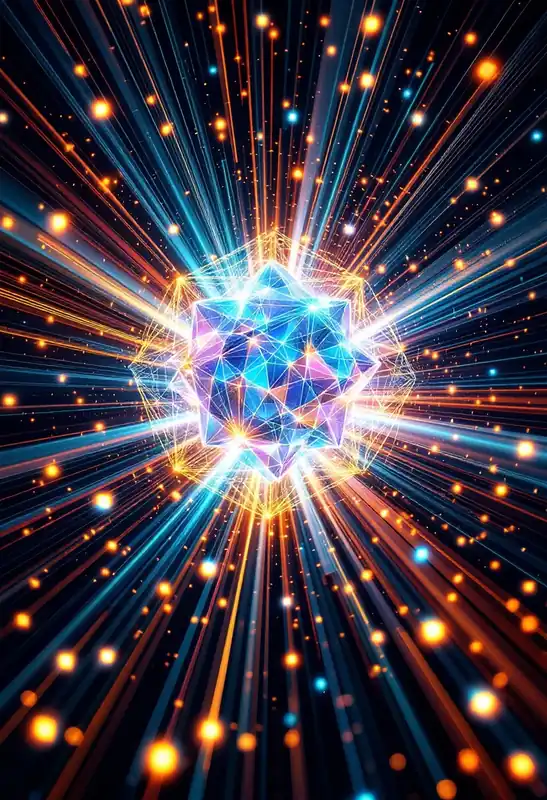
Sample Efficiency
Ability of a ML model to achieve high performance with a relatively small number of training samples.
Generality: 815
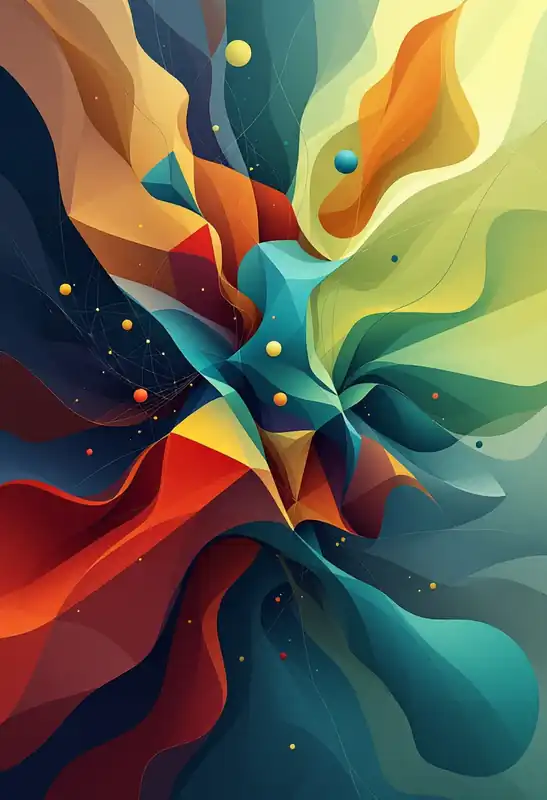
PPO
Proximal Policy Optimization
Proximal Policy Optimization
RL algorithm that aims to balance ease of implementation, sample efficiency, and reliable performance by using a simpler but effective update method for policy optimization.
Generality: 670
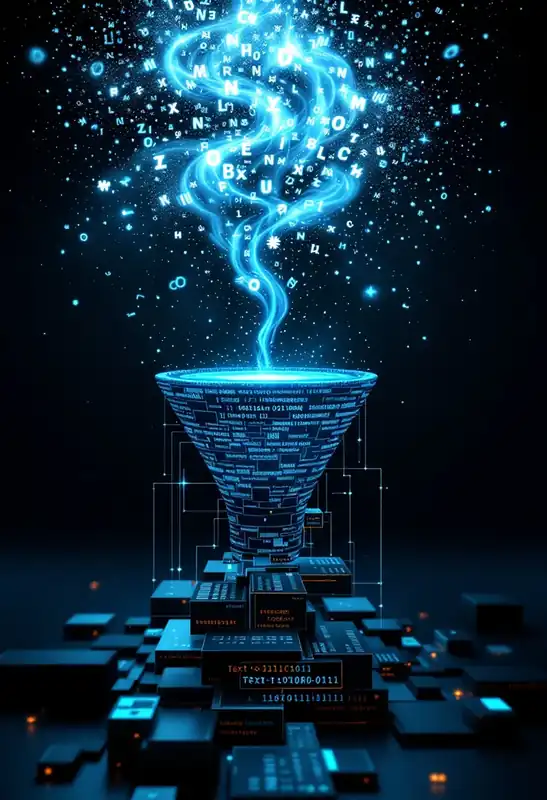
Text-to-Code Model
AI models designed to translate natural language descriptions into executable code snippets, facilitating automation in software development and assisting developers.
Generality: 665