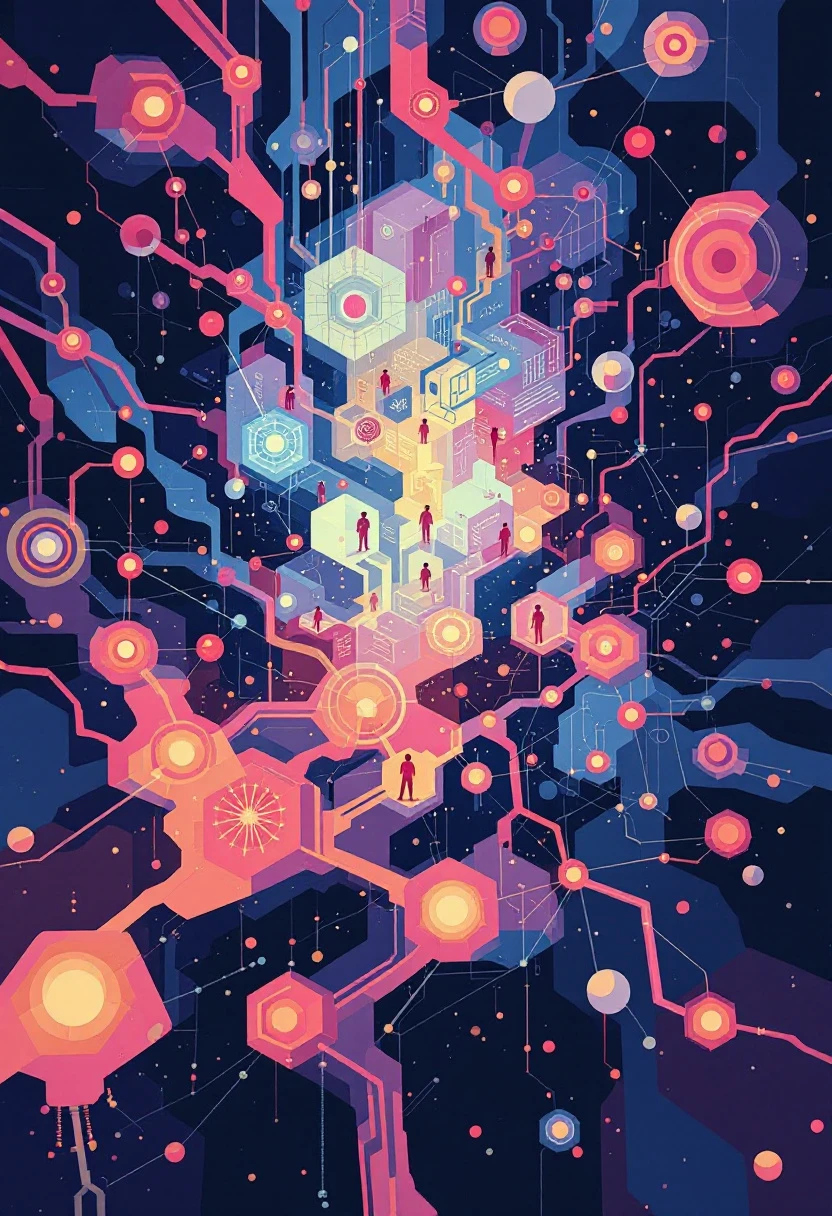
Automation Bias
Tendency of humans to over-rely on automated systems, potentially neglecting or undervaluing human input or overriding system feedback.
Automation bias reflects the cognitive inclination to favor suggestions from automated decision-making systems over contradictory information or advice from human sources, leading to potential errors in judgment or decision-making. This bias becomes particularly significant in AI, where reliance on AI-driven outputs can overshadow critical human oversight, causing a reduction in the engagement of human analytical skills and leading to over-trust in system recommendations. In high-stakes domains like healthcare, aviation, and finance, where AI systems are increasingly integrated, automation bias can lead to serious consequences if unchecked; thus, understanding its implications is essential for developing frameworks that balance automated input with human intuition, ensuring robust approaches to AI system deployment.
The term "automation bias" emerged in the mid-1990s, as AI systems began to play more integral roles in decision-making processes. Its significance grew with the proliferation of AI-backed tools in various industries, spurring research into human-computer interaction and emphasizing the necessity to mitigate over-reliance on automation from the early 2000s onwards.
Key contributors to the understanding and study of automation bias include researchers like Raja Parasuraman and Victor Riley, who explored human interaction with automated systems and highlighted the cognitive consequences and impact of increased automation on human decision-making proficiency. Their work laid important groundwork for developing strategies to counteract automation bias, emphasizing the importance of maintaining a balance between technology and human expertise.