In Silico Farming
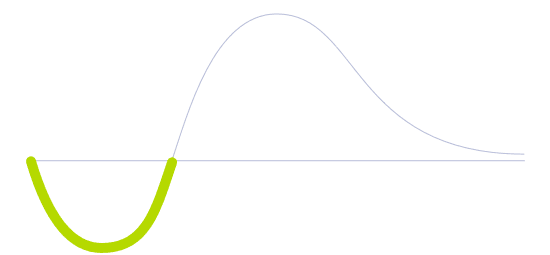
Technology Life Cycle
Initial phase where new technologies are conceptualized and developed. During this stage, technical viability is explored and initial prototypes may be created.
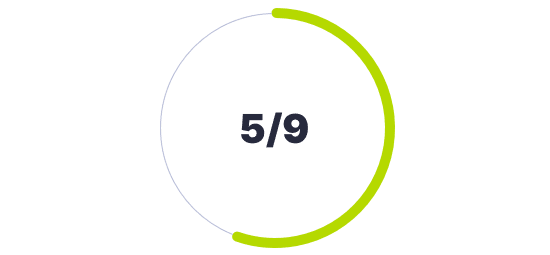
Technology Readiness Level (TRL)
Validation is conducted in relevant environments, where simulations are carried out as close to realistic circumstances.
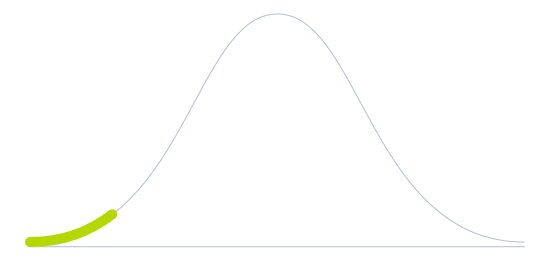
Technology Diffusion
First to adopt new technologies. They are willing to take risks and are crucial to the initial testing and development of new applications.
In silico studies are computer simulations with 3D models reflecting the real world as closely as possible. Unlike digital twins, in silico designs can react to specific inputs and provide conclusions for further analysis. In silico farming is a farming ecosystem transformed into code, where planting patterns, tillage systems, sunlight and shading, water availability, microbial interactions, and many other variables are digitally tested. By modeling the coupled plant-soil atmosphere management ecosystem, the simulated spatial resolution and precision output could potentially be comparable to having a large number of physical sensors distributed in the field.
Recently, in silico trials are being applied in crop science to monitor highly accurate digital plants to help speed up selective breeding. Accordingly, researchers can build statistical models that identify mathematical relationships using agricultural data and then create simulations based on those equations, allowing the measured traits to play out on the screen. Once they visualize the crops, scientists can manipulate the data to see which factors result in the fastest-growing, most drought-resistant, or least pest-susceptible plants possible.
Currently, this method cannot help individual farmers to manage their operations. It is mainly used by international organizations, national governments, and multinational corporations that run what-if scenarios about climate change and agriculture policy.
Future Perspectives
In silico farming is a bottom-up approach, meaning the process begins by simulating the properties of individual plants and then extrapolating the data and behavior to determine the yield for whole crops. Machine learning methods, on the other hand, offer a top-down approach to crop prediction. It processes data captured by drones and satellites with publicly available remote sensing data, subsequently tracking and predicting the impact of environmental factors on crops, including weather changes, soil parameters, and possible plant diseases.
The integration of top-down predictive models with bottom-up simulations at the individual plant level would make it possible to reap the best of both worlds: high spatial resolution and sound predictive forecasts for entire crops. In the future, instead of merely responding to food shortages, it could be possible to preemptively set appropriate food reserve levels, identify low-yield regions, and predict where to send food aid. If computer simulations scale up, they could boost the democratization of precision, taking mono, polyculture, and agroforestry farming to new levels.
Image generated by Envisioning using Midjourney