Autonomous Maintenance Software
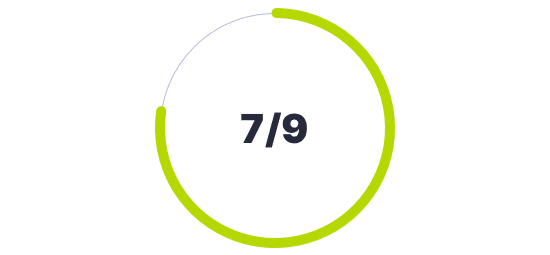
Technology Readiness Level (TRL)
Prototype is fully demonstrated in operational environment.
Artificial intelligence maintenance techniques are being employed in a wide range of industries, covering everything from policing to prevention, decision-making, and operational problem-solving. They extend from intelligent maintenance optimization models, simulations, and intra-logistical processes to practical applications, such as selecting optimal repair methods or cost budgeting. With the ever-increasing complexity of big industries, automated and artificial intelligence algorithms have been essential tools to deal with such an immense amount of data and crossing parameters. Several inputs, such as energy consumption, material consumption, carbon footprint, and efficiency, have to be taken into consideration to extend the life of existing assets for as long as possible.
Existing hybrid AI techniques are employed in industrial maintenance management, meaning that each AI method succeeds in assessing specific dimensions of intelligence. For instance, fuzzy logic is largely employed when dealing with uncertainty, like risk-based inspection, and has been successfully used in the gas and oil industry to estimate risk by combining the likelihood of occurrence and the potential consequences. On the other hand, neural network algorithms are often used in modeling and simulating statistical analysis for optimization. It helps to estimate the remaining life of machines and components, predicting what impact degradation of individual pieces would have on the final product. It has been primarily used in sustainable management maintenance models of supply chains.
The most common AI application in maintenance is genetic algorithms due to the powerful optimization tools able to deal with complex maintenance planning issues, like maintenance and inspection schedule. Also, genetic algorithm solutions can “evolve” with mutation, and weaker solutions become extinct. However, genetic algorithms may require high computing power and processing due to their intricate nature, but they continue to be a powerful solution for large-scale and elaborate applications of unaccounted variables.
Nevertheless, complex AI systems could autonomously operate maintenance tools and robots, freeing human workers from dirty, dangerous tasks while eliminating errors and reducing variability. Upon integration with factory AI operating systems, robots would represent to manufacturing what a medical doctor means to a human. It would be able to identify which facilities require regular maintenance and optimize systems while preventing disturbances. Instead of deploying personnel when things break down in those settings, these systems could make it possible for places to run autonomously for long periods, allowing for factories to be created farther away from residential areas, deeply shaping the architecture of cities.
Future Perspectives
These AI hybrid implementations could autonomously acquire data at all levels of complexity by cross-checking information, improving and updating themselves, and coming up with sophisticated solutions, thus, working like the nervous system of the whole factory. Also, with everything interconnected, the hacking risk is likely to rise. Preemptive strategies should be developed and implemented to prevent these attacks before this technology is deployed.
Image generated by Envisioning using Midjourney