Algorithmic Bias Detection Tool
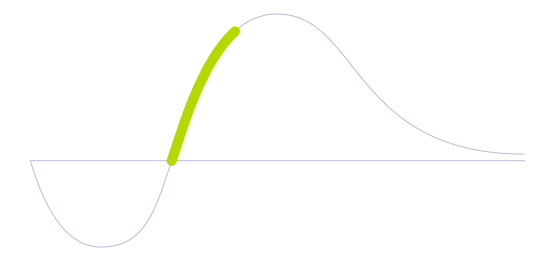
Technology Life Cycle
Marked by a rapid increase in technology adoption and market expansion. Innovations are refined, production costs decrease, and the technology gains widespread acceptance and use.
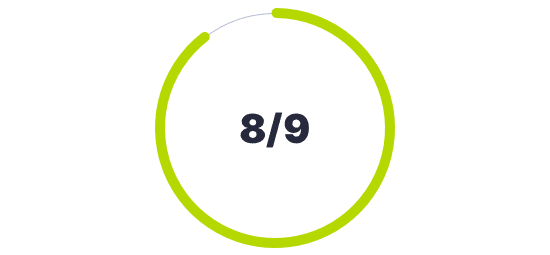
Technology Readiness Level (TRL)
Technology is developed and qualified. It is readily available for implementation but the market is not entirely familiar with the technology.
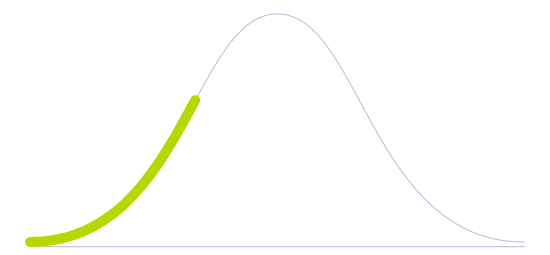
Technology Diffusion
Embrace new technologies soon after Innovators. They often have significant influence within their social circles and help validate the practicality of innovations.
As machine learning infiltrates society, we have realized that algorithms are not always perfect: algorithmic bias has already been detected in several examples. Although machine learning is a continuous form of statistical discrimination by its very nature, the kind of bias primarily addressed is unwanted variety, placing privileged groups at a systematic advantage and unprivileged groups at a systematic disadvantage. Examples include predictive policing systems that have been caught in runaway feedback loops of discrimination and hiring tests that end up excluding applicants from low-income neighborhoods or prefer male applicants to female ones.
Systems can be designed to scan algorithmic models and detect bias at different points in the machine learning pipeline: either in the training data or the learned model, which correlates to varying categories of bias mitigation techniques. The adversarial de-biasing procedure is currently one of the most popular methods to combat discrimination. It relies on adversarial training to remove bias from latent representations learned by the model. Besides that, metrics for datasets can measure outcomes that provide an advantage to a specific recipient or group that historically held a systematic position of power and partition a population into groups that should have equality in terms of benefits received.
This solution could reduce unfair outcomes and recommend specific changes to how the mathematical models interpret the data — inducing a reparation program through machine learning instead of perpetuating a centuries-old system that generates disadvantages for certain societal groups. By examining several public models, the amount of influence that sensitive variables — race, gender, class, and religion — have on data can be measured along with an estimated correlation between said variables. Researchers could also visualize how a given model’s outcomes are skewed and take preventative measures to make the model immune to these biases. This tool can influence various areas such as criminal justice, healthcare, finance, hiring, and recruitment.
There is an even more straightforward yet effective way of avoiding bias in machine learning models. Hiring a more diverse team of software engineers and data scientists by including people from different backgrounds such as gender, race, age, and diversified body and mental capabilities could inevitably help broaden the point of view of the algorithms they program.
Future Perspectives
Given the ubiquitous nature of algorithms and their deep-reaching impact on society, scientists are trying to help prevent injustice by creating tools that detect underlying unfairness in these programs. Even though the best technologies still serve as a means to an end, these solutions are essential in paving the way toward establishing trust. By encoding variational “fair” encoders, dynamic upsampling of training data based on learned representations, or preventing disparity through distributional optimization, these tools could possibly help create ethical and transparent principles for developing new AI technologies.
Since many bias-detection techniques can overlap with ethical challenges in different areas, such as structures for good governance, appropriate data sharing, and explainability of models, an all-encompassing solution to algorithmic bias must be established in both legal and technical terms to bridge the gap and minimize potential conflicts based on bias and prejudice. Otherwise, an unchecked market with access to increasingly powerful predictive tools can gradually and imperceptibly worsen social inequality, perhaps even leading to a new era of information warfare. In light of this dystopian outcome, governments worldwide, including Singapore, South Korea, and the United Arab Emirates, have announced AI ethics as a new board/committee/ministry to be integrated into their political system.
Image generated by Envisioning using Midjourney